Learning effective stochastic differential equations from microscopic simulations: combining stochastic numerics and deep learning.
CoRR(2021)
摘要
We identify effective stochastic differential equations (SDE) for coarse observables of fine-grained particle- or agent-based simulations; these SDE then provide coarse surrogate models of the fine scale dynamics. We approximate the drift and diffusivity functions in these effective SDE through neural networks, which can be thought of as effective stochastic ResNets. The loss function is inspired by, and embodies, the structure of established stochastic numerical integrators (here, Euler-Maruyama and Milstein); our approximations can thus benefit from error analysis of these underlying numerical schemes. They also lend themselves naturally to "physics-informed" gray-box identification when approximate coarse models, such as mean field equations, are available. Our approach does not require long trajectories, works on scattered snapshot data, and is designed to naturally handle different time steps per snapshot. We consider both the case where the coarse collective observables are known in advance, as well as the case where they must be found in a data-driven manner.
更多查看译文
关键词
stochastic differential equations,effective stochastic differential equations,stochastic numerics,deep learning
AI 理解论文
溯源树
样例
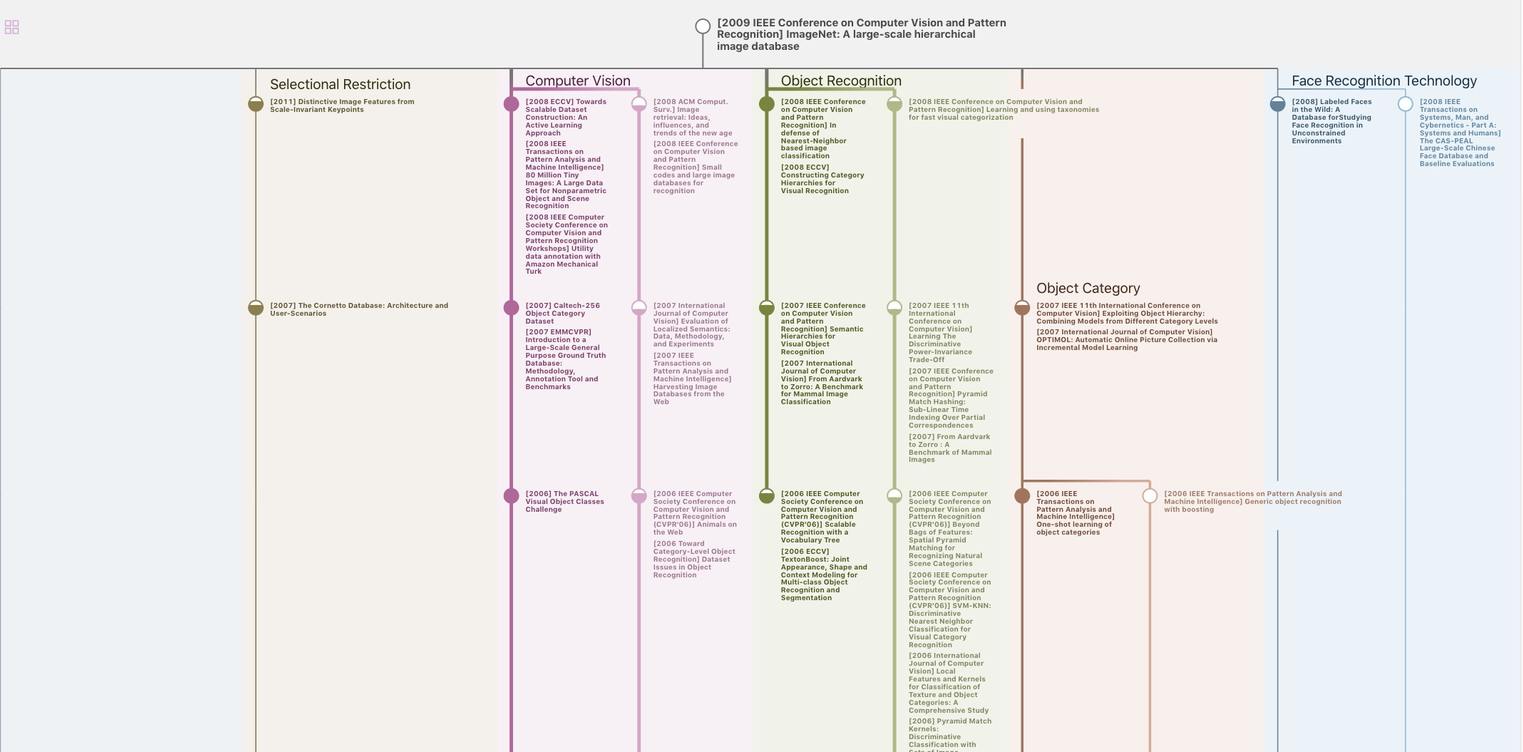
生成溯源树,研究论文发展脉络
Chat Paper
正在生成论文摘要