How Self-Supervised Learning Can be Used for Fine-Grained Head Pose Estimation?
CoRR(2021)
摘要
Recent progress of Self-Supervised Learning (SSL) demonstrates the capability of these methods in computer vision field. However, this progress could not show any promises for fine-grained tasks such as Head Pose estimation. In this article, we have tried to answer a question: How SSL can be used for Head Pose estimation? In general, there are two main approaches to use SSL: (1) Using pre-trained weights which can be done via SSL tasks, (2) Leveraging SSL as an auxiliary co-training task besides of Supervised Learning (SL) tasks at the same time. In this study, modified versions of jigsaw puzzling and rotation as SSL pre-text tasks are used and the best architecture for our proposed Hybrid Multi-Task Learning (HMTL) is found. Finally, the HopeNet method as a baseline SL is selected and the impact of SSL pre-training and ImageNet pre-training on both HMTL and SL are compared. The error rate reduced by the HMTL method up to 13% compare to the SL. Moreover, HMTL method showed that it was good with all kinds of initial weights: random, ImageNet and SSL pre-training weights. Also, it was observed, when puzzled images are used for SL alone, the average error rate placed between SL and HMTL, showed the importance of local spatial features compare to global spatial features.
更多查看译文
关键词
head,learning,estimation,self-supervised,fine-grained
AI 理解论文
溯源树
样例
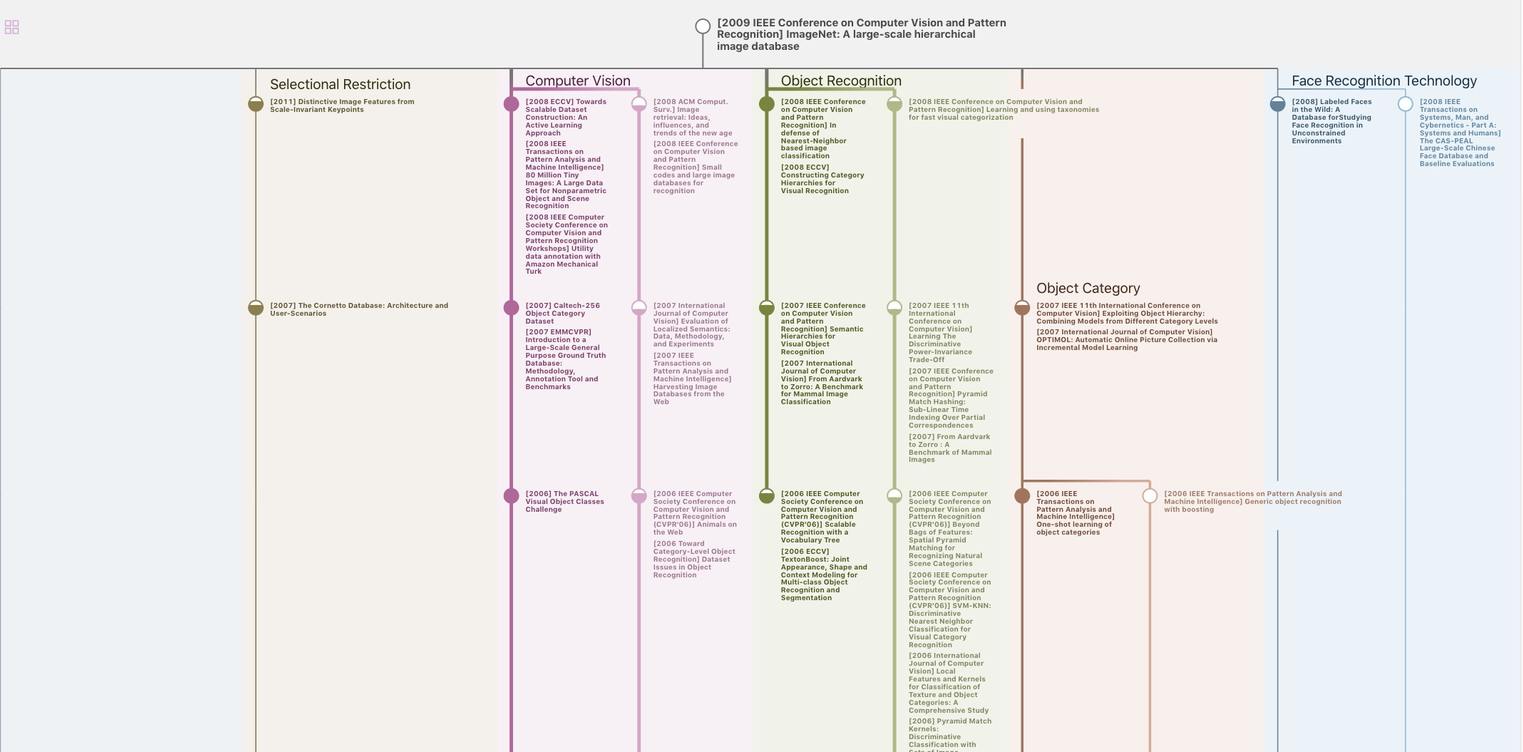
生成溯源树,研究论文发展脉络
Chat Paper
正在生成论文摘要