Data-driven remanufacturing planning with parameter uncertainty
Eur. J. Oper. Res.(2023)
摘要
We consider the problem of remanufacturing planning in the presence of statistical estimation errors. Determining the optimal remanufacturing timing, first and foremost, requires modeling of the state tran-sitions of a system. The estimation of these probabilities, however, often suffers from data inadequacy and is far from accurate, resulting in serious degradation in performance. To mitigate the impacts of the uncertainty in transition probabilities, we develop a novel data-driven modeling framework for reman-ufacturing planning in which decision makers can remain robust with respect to statistical estimation errors. We model the remanufacturing planning problem as a robust Markov decision process, and con-struct ambiguity sets that contain the true transition probabilities with high confidence. We further es-tablish structural properties of optimal robust policies and provide insights for remanufacturing planning. A computational study on the NASA turbofan engine shows that our data-driven robust decision frame-work consistently yields better out-of-sample reward and higher reliability of the performance guarantee, compared to the nominal model that uses the maximum likelihood estimates of the transition probabili-ties without considering parameter uncertainty. (c) 2023 Elsevier B.V. All rights reserved.
更多查看译文
关键词
Maintenance,Remanufacturing planning,Robust Markov decision process,Control-limit policy
AI 理解论文
溯源树
样例
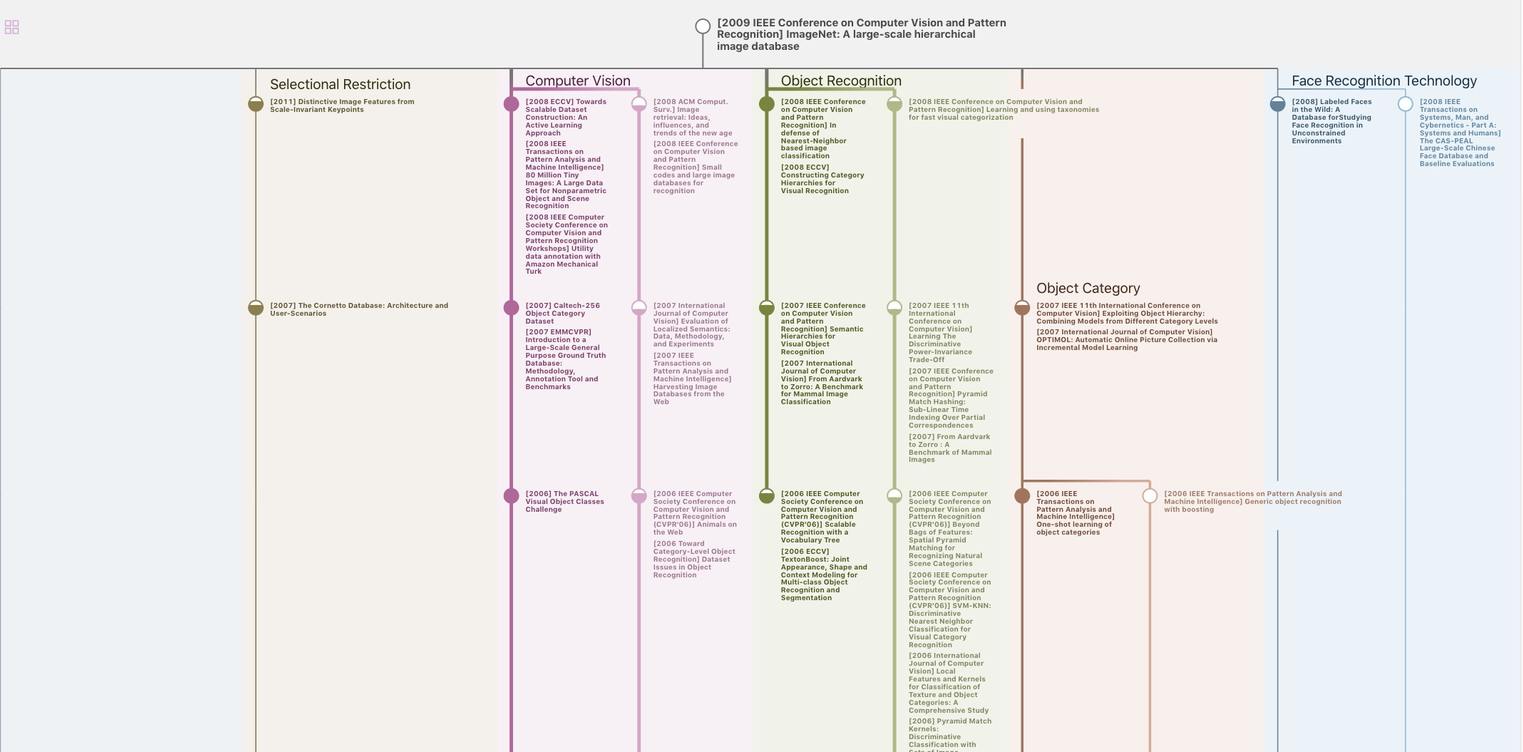
生成溯源树,研究论文发展脉络
Chat Paper
正在生成论文摘要