High-Resolution Quantitative Retrieval of Soil Moisture Based on Multisource Data Fusion with Random Forests: A Case Study in the Zoige Region of the Tibetan Plateau.
Remote. Sens.(2023)
摘要
Accurate high-resolution soil moisture mapping is critical for surface studies as well as climate change research. Currently, regional soil moisture retrieval primarily focuses on a spatial resolution of 1 km, which is not able to provide effective information for environmental science research and agricultural water resource management. In this study, we developed a quantitative retrieval framework for high-resolution (250 m) regional soil moisture inversion based on machine learning, multisource data fusion, and in situ measurement data. Specifically, we used various data sources, including the normalized vegetation index, surface temperature, surface albedo, soil properties data, precipitation data, topographic data, and soil moisture products from passive microwave data assimilation as input parameters. The soil moisture products simulated based on ground model simulation were used as supplementary data of the in situ measurements, together with the measured data from the Maqu Observation Network as the training target value. The study was conducted in the Zoige region of the Tibetan Plateau during the nonfreezing period (May-October) from 2009 to 2018, using random forests for training. The random forest model had good accuracy, with a correlation coefficient of 0.885, a root mean square error of 0.024 m(3)/m(3), and a bias of -0.004. The ground-measured soil moisture exhibited significant fluctuations, while the random forest prediction was more accurate and closely aligned with the field soil moisture compared to the soil moisture products based on ground model simulation. Our method generated results that were smoother, more stable, and with less noise, providing a more detailed spatial pattern of soil moisture. Based on the permutation importance method, we found that topographic factors such as slope and aspect, and soil properties such as silt and sand have significant impacts on soil moisture in the southeastern Tibetan Plateau. This highlights the importance of fine-scale topographic and soil property information for generating high-precision soil moisture data. From the perspective of inter-annual variation, the soil moisture in this area is generally high, showing a slow upward trend, with small spatial differences, and the annual average value fluctuates between 0.3741 m(3)/m(3) and 0.3943 m(3)/m(3). The intra-annual evolution indicates that the monthly mean average soil moisture has a large geographical variation and a small multi-year linear change rate. These findings can provide valuable insights and references for regional soil moisture research.
更多查看译文
关键词
soil moisture,multisource data fusion,tibetan plateau,random forests,high-resolution
AI 理解论文
溯源树
样例
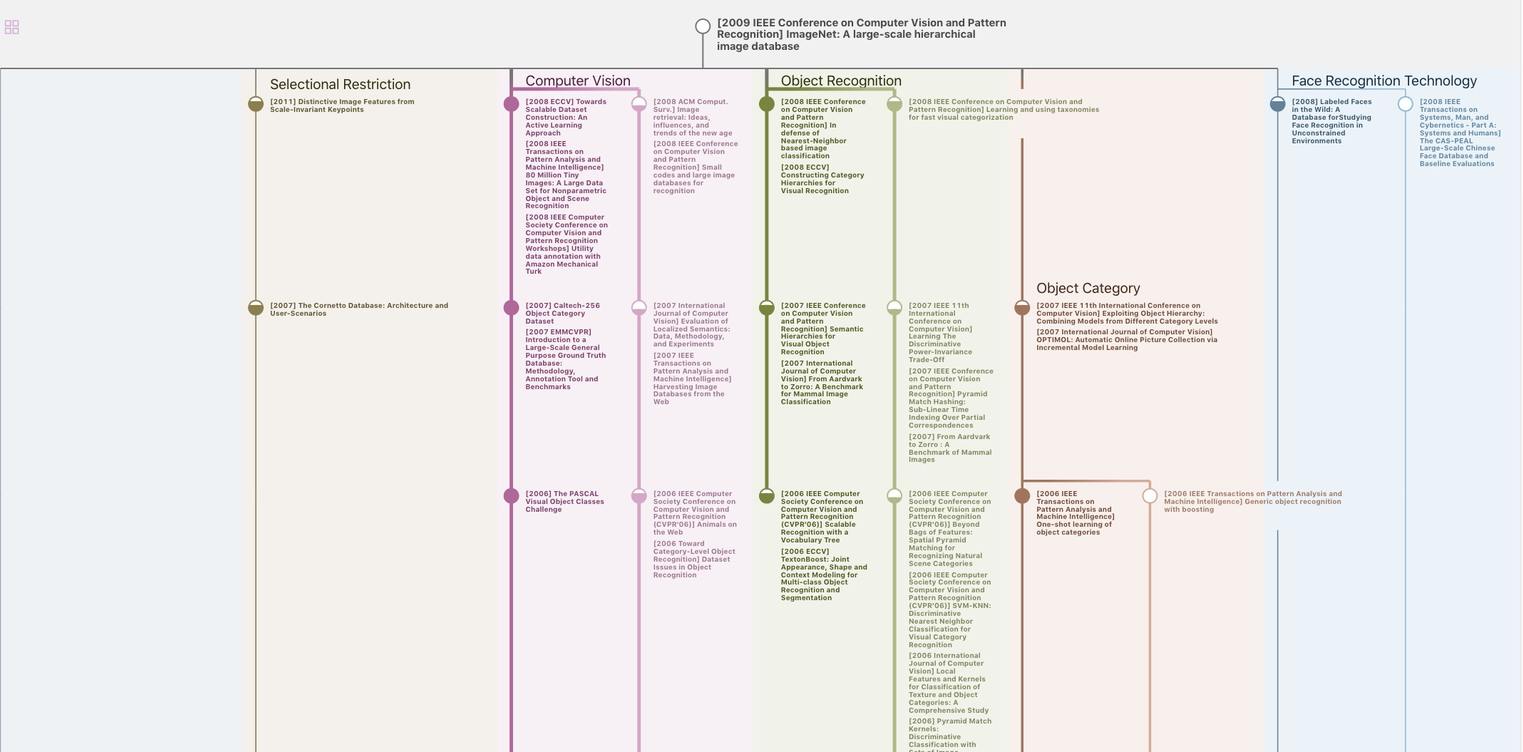
生成溯源树,研究论文发展脉络
Chat Paper
正在生成论文摘要