Expectation maximisation pseudo labels
MEDICAL IMAGE ANALYSIS(2024)
摘要
In this paper, we study pseudo -labelling. Pseudo -labelling employs raw inferences on unlabelled data as pseudolabels for self -training. We elucidate the empirical successes of pseudo -labelling by establishing a link between this technique and the Expectation Maximisation algorithm. Through this, we realise that the original pseudolabelling serves as an empirical estimation of its more comprehensive underlying formulation. Following this insight, we present a full generalisation of pseudo -labels under Bayes' theorem, termed Bayesian Pseudo Labels. Subsequently, we introduce a variational approach to generate these Bayesian Pseudo Labels, involving the learning of a threshold to automatically select high -quality pseudo labels. In the remainder of the paper, we showcase the applications of pseudo -labelling and its generalised form, Bayesian Pseudo -Labelling, in the semi -supervised segmentation of medical images. Specifically, we focus on: (1) 3D binary segmentation of lung vessels from CT volumes; (2) 2D multi -class segmentation of brain tumours from MRI volumes; (3) 3D binary segmentation of whole brain tumours from MRI volumes; and (4) 3D binary segmentation of prostate from MRI volumes. We further demonstrate that pseudo -labels can enhance the robustness of the learned representations. The code is released in the following GitHub repository: https://github.com/moucheng2017/EMSSL.
更多查看译文
关键词
Pseudo labels,Bayesian deep learning,Expectation-maximisation,Semi-supervised learning,Segmentation,Generative models,Robustness
AI 理解论文
溯源树
样例
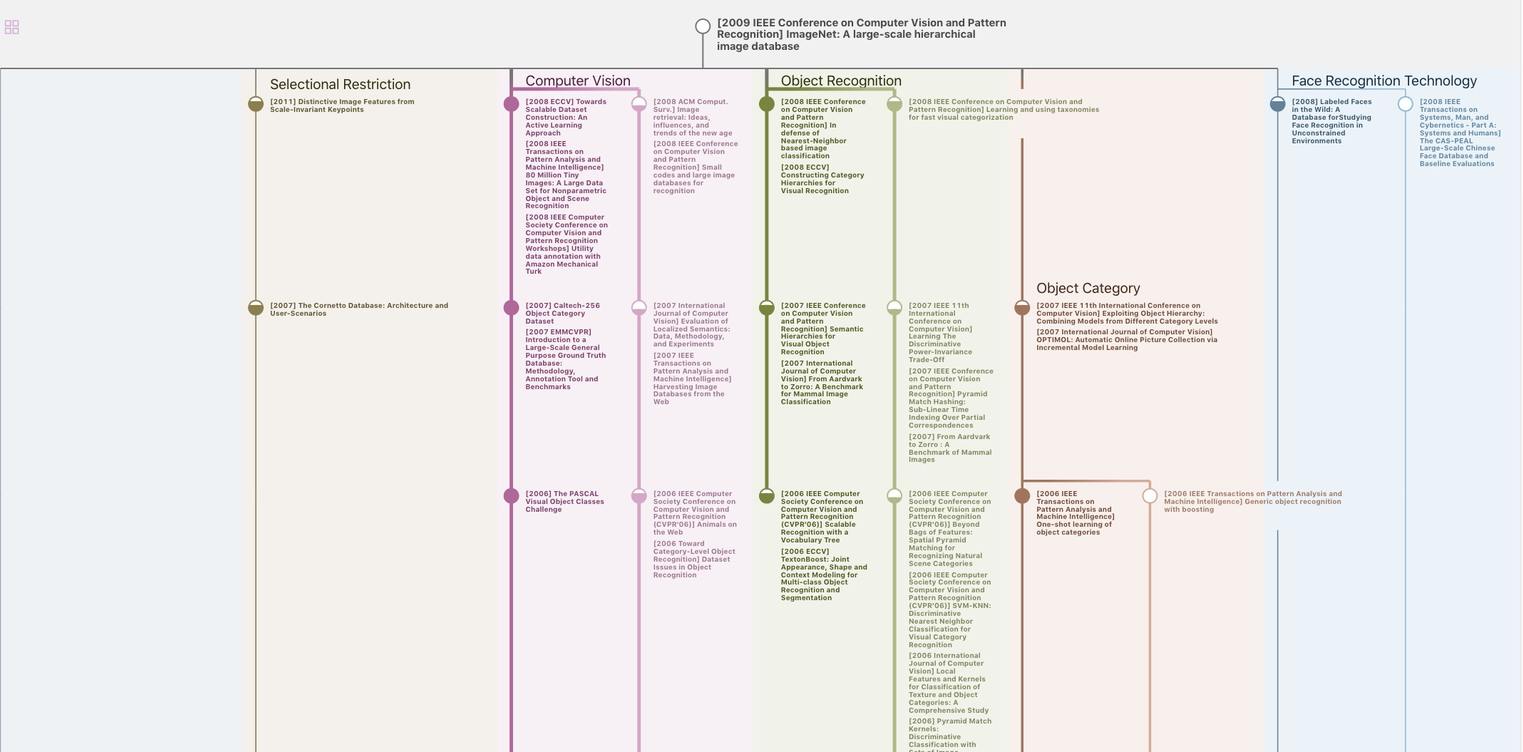
生成溯源树,研究论文发展脉络
Chat Paper
正在生成论文摘要