NELoRa-Bench: A Benchmark for Neural-enhanced LoRa Demodulation
CoRR(2023)
摘要
Low-Power Wide-Area Networks (LPWANs) are an emerging Internet-of-Things (IoT) paradigm marked by low-power and long-distance communication. Among them, LoRa is widely deployed for its unique characteristics and open-source technology. By adopting the Chirp Spread Spectrum (CSS) modulation, LoRa enables low signal-to-noise ratio (SNR) communication. The standard LoRa demodulation method accumulates the chirp power of the whole chirp into an energy peak in the frequency domain. In this way, it can support communication even when SNR is lower than -15 dB. Beyond that, we proposed NELoRa, a neural-enhanced decoder that exploits multi-dimensional information to achieve significant SNR gain. This paper presents the dataset used to train/test NELoRa, which includes 27,329 LoRa symbols with spreading factors from 7 to 10, for further improvement of neural-enhanced LoRa demodulation. The dataset shows that NELoRa can achieve 1.84-2.35 dB SNR gain over the standard LoRa decoder. The dataset and codes can be found at https://github.com/daibiaoxuwu/NeLoRa_Dataset.
更多查看译文
关键词
nelora-bench demodulation,benchmark,neural-enhanced
AI 理解论文
溯源树
样例
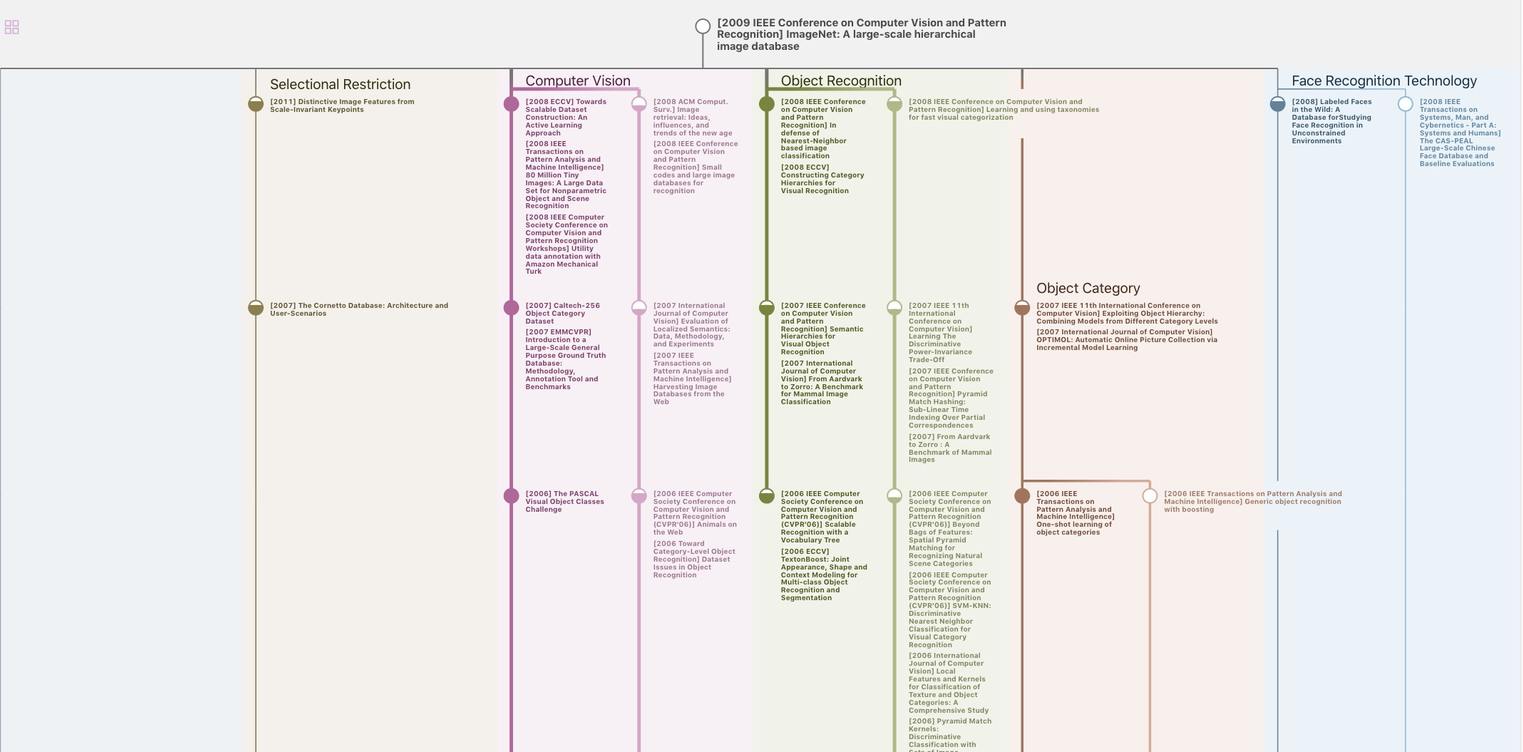
生成溯源树,研究论文发展脉络
Chat Paper
正在生成论文摘要