The Pipeline System of ASR and NLU with MLM-based data Augmentation Toward Stop Low-Resource Challenge
CoRR(2023)
摘要
This paper describes our system for the low-resource domain adaptation track (Track 3) in Spoken Language Understanding Grand Challenge, which is a part of ICASSP Signal Processing Grand Challenge 2023. In the track, we adopt a pipeline approach of ASR and NLU. For ASR, we fine-tune Whisper for each domain with upsampling. For NLU, we fine-tune BART on all the Track3 data and then on low-resource domain data. We apply masked LM (MLM) -based data augmentation, where some of input tokens and corresponding target labels are replaced using MLM. We also apply a retrieval-based approach, where model input is augmented with similar training samples. As a result, we achieved exact match (EM) accuracy 63.3/75.0 (average: 69.15) for reminder/weather domain, and won the 1st place at the challenge.
更多查看译文
关键词
ASR,BART,ICASSP Signal Processing Grand Challenge 2023,low-resource domain adaptation track,masked LM based data augmentation,MLM-based data augmentation,NLU,pipeline system,retrieval-based approach,Spoken Language Understanding Grand Challenge,STOP low-resource challenge,Track3 data,Whisper
AI 理解论文
溯源树
样例
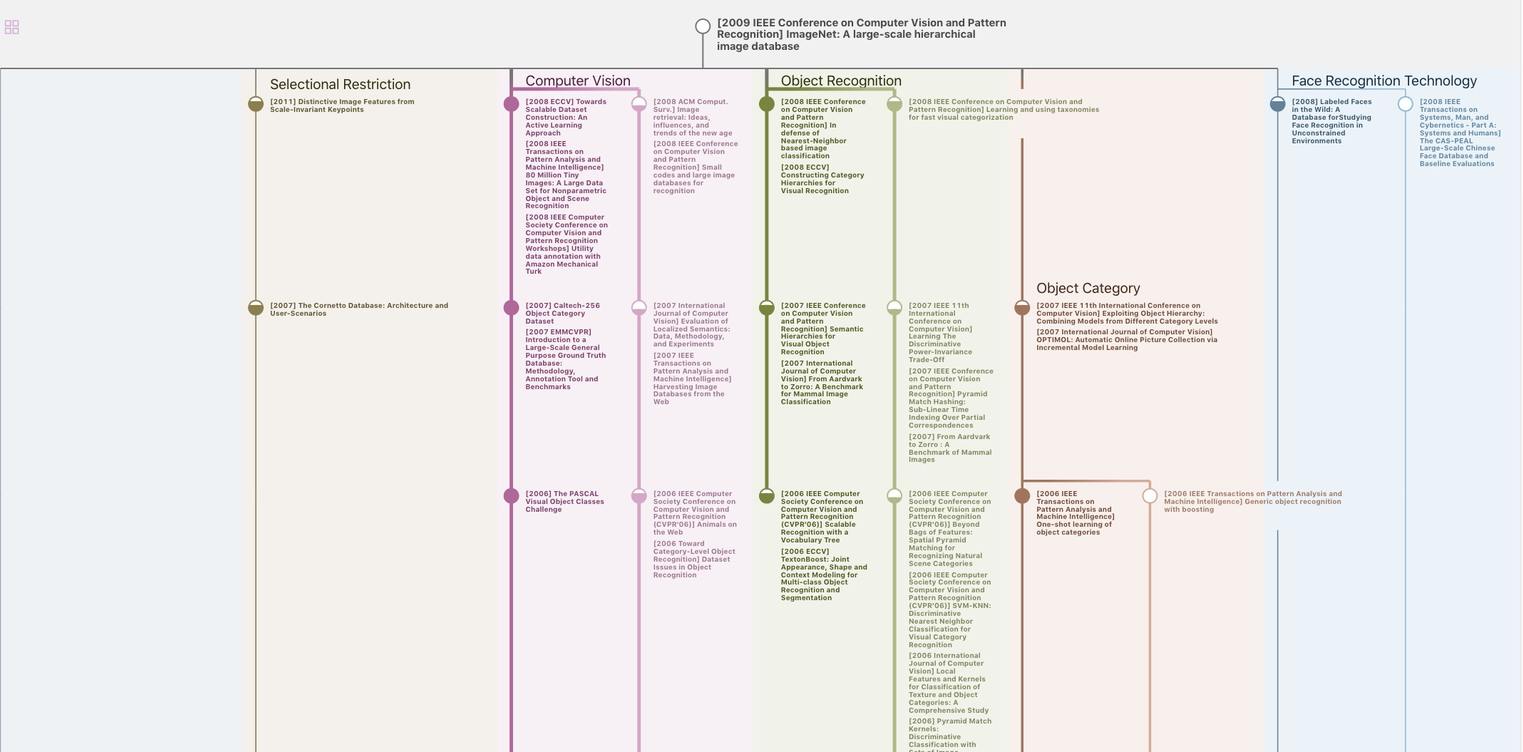
生成溯源树,研究论文发展脉络
Chat Paper
正在生成论文摘要