ViewFormer: View Set Attention for Multi-view 3D Shape Understanding
CoRR(2023)
摘要
This paper presents ViewFormer, a simple yet effective model for multi-view 3d shape recognition and retrieval. We systematically investigate the existing methods for aggregating multi-view information and propose a novel ``view set" perspective, which minimizes the relation assumption about the views and releases the representation flexibility. We devise an adaptive attention model to capture pairwise and higher-order correlations of the elements in the view set. The learned multi-view correlations are aggregated into an expressive view set descriptor for recognition and retrieval. Experiments show the proposed method unleashes surprising capabilities across different tasks and datasets. For instance, with only 2 attention blocks and 4.8M learnable parameters, ViewFormer reaches 98.8% recognition accuracy on ModelNet40 for the first time, exceeding previous best method by 1.1% . On the challenging RGBD dataset, our method achieves 98.4% recognition accuracy, which is a 4.1% absolute improvement over the strongest baseline. ViewFormer also sets new records in several evaluation dimensions of 3D shape retrieval defined on the SHREC'17 benchmark.
更多查看译文
关键词
3d,shape,attention,multi-view
AI 理解论文
溯源树
样例
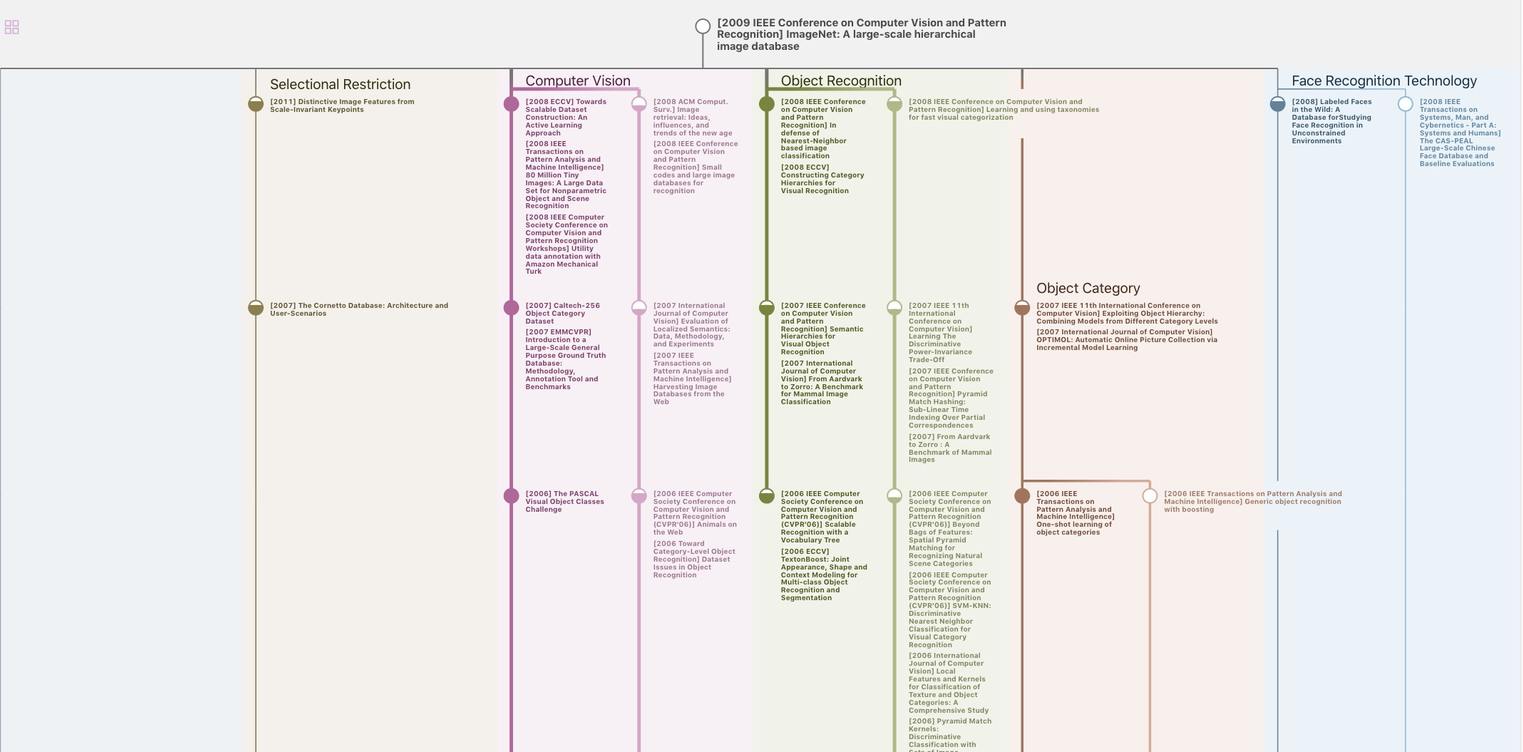
生成溯源树,研究论文发展脉络
Chat Paper
正在生成论文摘要