Differentiable Sensor Layouts for End-to-End Learning of Task-Specific Camera Parameters
CoRR(2023)
摘要
The success of deep learning is frequently described as the ability to train all parameters of a network on a specific application in an end-to-end fashion. Yet, several design choices on the camera level, including the pixel layout of the sensor, are considered as pre-defined and fixed, and high resolution, regular pixel layouts are considered to be the most generic ones in computer vision and graphics, treating all regions of an image as equally important. While several works have considered non-uniform, \eg, hexagonal or foveated, pixel layouts in hardware and image processing, the layout has not been integrated into the end-to-end learning paradigm so far. In this work, we present the first truly end-to-end trained imaging pipeline that optimizes the size and distribution of pixels on the imaging sensor jointly with the parameters of a given neural network on a specific task. We derive an analytic, differentiable approach for the sensor layout parameterization that allows for task-specific, local varying pixel resolutions. We present two pixel layout parameterization functions: rectangular and curvilinear grid shapes that retain a regular topology. We provide a drop-in module that approximates sensor simulation given existing high-resolution images to directly connect our method with existing deep learning models. We show that network predictions benefit from learnable pixel layouts for two different downstream tasks, classification and semantic segmentation.
更多查看译文
关键词
differentiable sensor layouts,camera,learning,end-to-end,task-specific
AI 理解论文
溯源树
样例
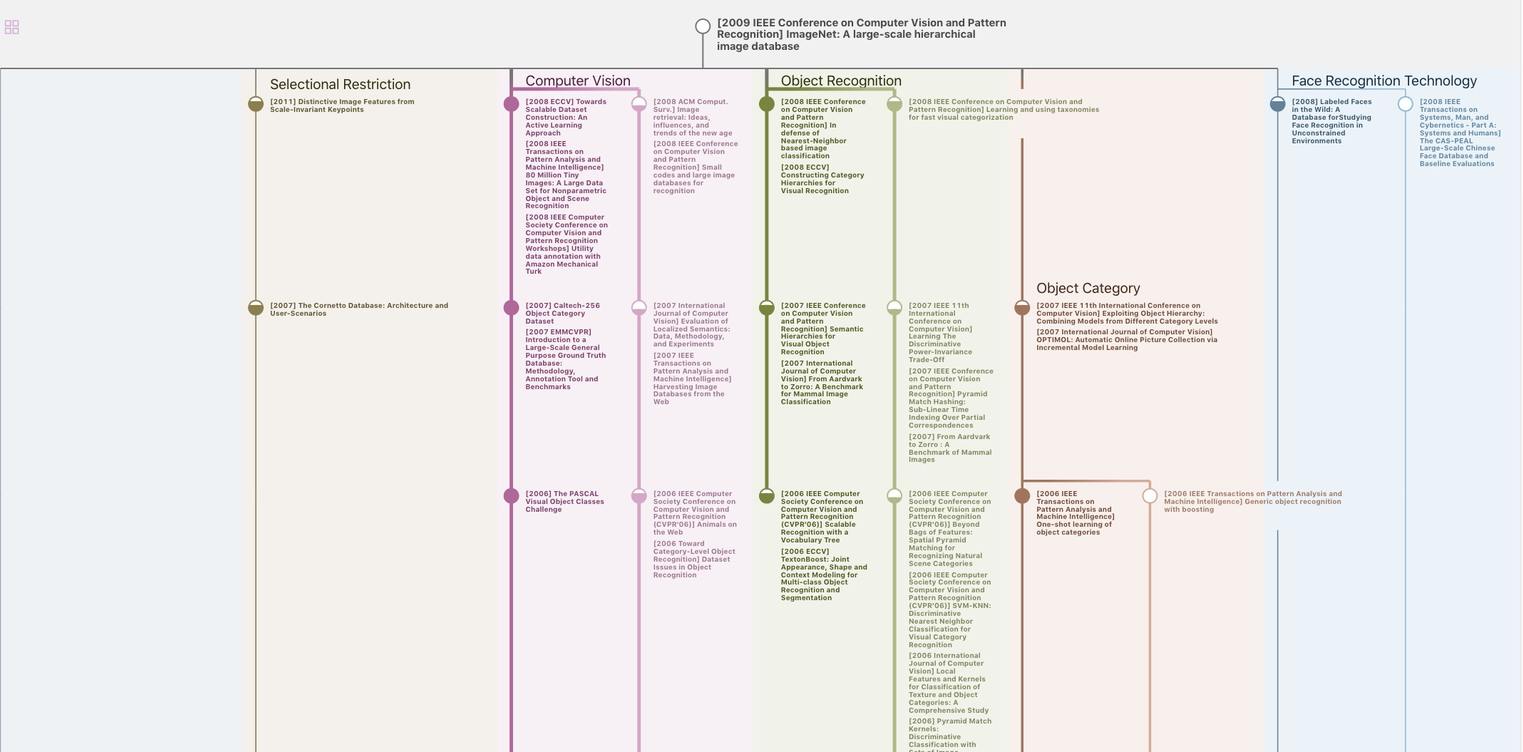
生成溯源树,研究论文发展脉络
Chat Paper
正在生成论文摘要