PreNAS: Preferred One-Shot Learning Towards Efficient Neural Architecture Search
CoRR(2023)
摘要
The wide application of pre-trained models is driving the trend of once-for-all training in one-shot neural architecture search (NAS). However, training within a huge sample space damages the performance of individual subnets and requires much computation to search for an optimal model. In this paper, we present PreNAS, a search-free NAS approach that accentuates target models in one-shot training. Specifically, the sample space is dramatically reduced in advance by a zero-cost selector, and weight-sharing one-shot training is performed on the preferred architectures to alleviate update conflicts. Extensive experiments have demonstrated that PreNAS consistently outperforms state-of-the-art one-shot NAS competitors for both Vision Transformer and convolutional architectures, and importantly, enables instant specialization with zero search cost. The code will be released upon acceptance.
更多查看译文
关键词
architecture search,efficient neural
AI 理解论文
溯源树
样例
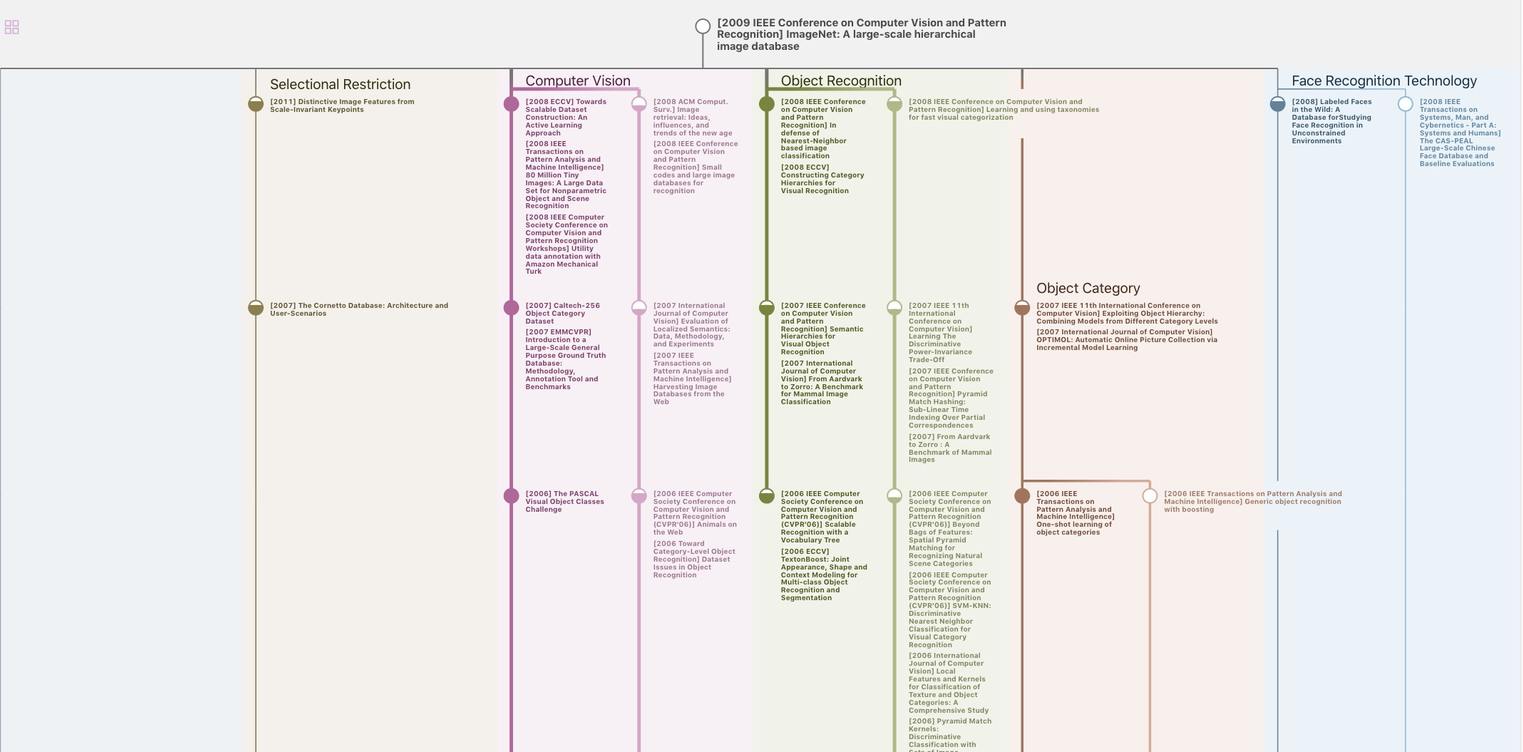
生成溯源树,研究论文发展脉络
Chat Paper
正在生成论文摘要