Oversampling Higher-Performing Minorities During Machine Learning Model Training Reduces Adverse Impact Slightly but Also Reduces Model Accuracy
CoRR(2023)
摘要
Organizations are increasingly adopting machine learning (ML) for personnel assessment. However, concerns exist about fairness in designing and implementing ML assessments. Supervised ML models are trained to model patterns in data, meaning ML models tend to yield predictions that reflect subgroup differences in applicant attributes in the training data, regardless of the underlying cause of subgroup differences. In this study, we systematically under- and oversampled minority (Black and Hispanic) applicants to manipulate adverse impact ratios in training data and investigated how training data adverse impact ratios affect ML model adverse impact and accuracy. We used self-reports and interview transcripts from job applicants (N = 2,501) to train 9,702 ML models to predict screening decisions. We found that training data adverse impact related linearly to ML model adverse impact. However, removing adverse impact from training data only slightly reduced ML model adverse impact and tended to negatively affect ML model accuracy. We observed consistent effects across self-reports and interview transcripts, whether oversampling real (i.e., bootstrapping) or synthetic observations. As our study relied on limited predictor sets from one organization, the observed effects on adverse impact may be attenuated among more accurate ML models.
更多查看译文
关键词
minorities,training,machine learning model
AI 理解论文
溯源树
样例
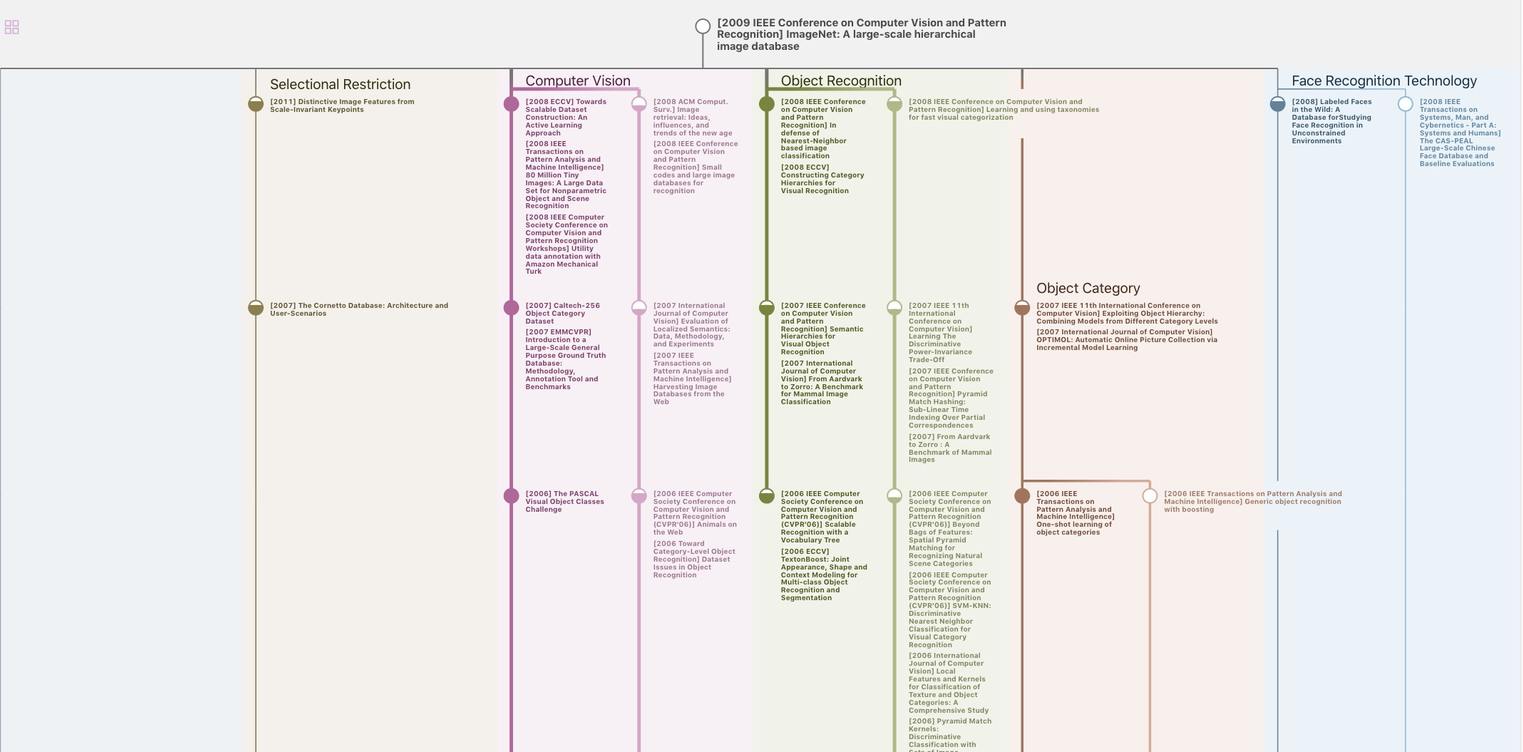
生成溯源树,研究论文发展脉络
Chat Paper
正在生成论文摘要