Shape-Adaptive Ternary-Gaussian Model: Modeling Pointing Uncertainty for Moving Targets of Arbitrary Shapes
CHI 2023(2023)
摘要
This paper presents a Shape-Adaptive Ternary-Gaussian model for describing endpoint uncertainty when pointing at moving targets of arbitrary shapes. The basic idea of the model is to combine the uncertainty related to the target shape with the uncertainty caused by the target motion. First, we proposed a model to predict endpoint distribution on static targets based on a Dual-Space Decomposition (DUDE) algorithm. Then, we linearly combined a 2D Ternary-Gaussian model with the newly proposed DUDE-based model to make the 2D Ternary-Gaussian model adaptable to moving targets with random shapes. To verify the performance of our model, we compared it with the original 2D Ternary-Gaussian model and a recent proposed Inscribed Circle model in predicting endpoint distribution. The results show that the proposed model outperformed the two baseline models while maintaining good robustness across different shapes and moving speeds.
更多查看译文
关键词
Moving Target Selection, Arbitrary Shapes, Pointing Uncertainty, Models, Endpoint Distribution
AI 理解论文
溯源树
样例
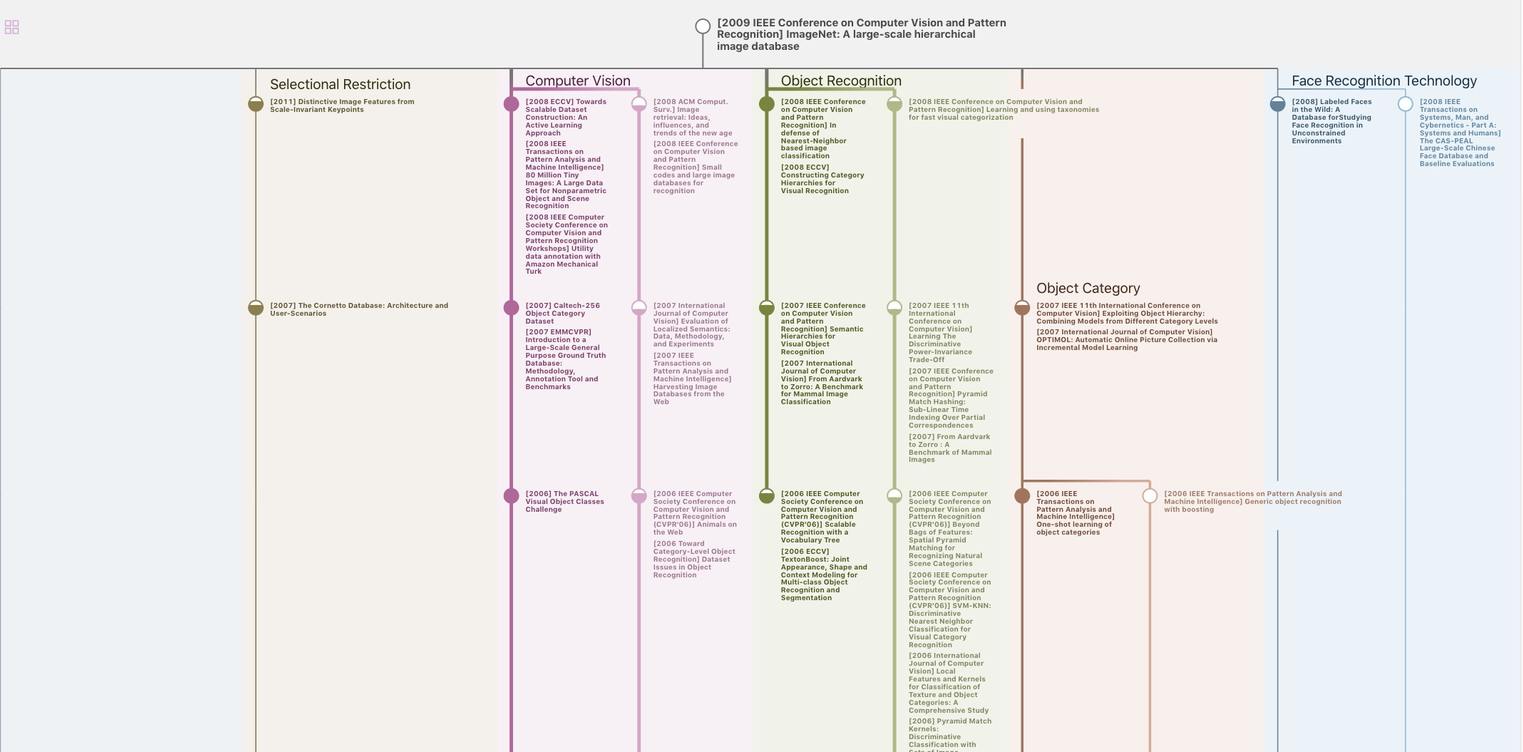
生成溯源树,研究论文发展脉络
Chat Paper
正在生成论文摘要