OPDN: Omnidirectional Position-aware Deformable Network for Omnidirectional Image Super-Resolution
CoRR(2023)
摘要
360{\deg} omnidirectional images have gained research attention due to their immersive and interactive experience, particularly in AR/VR applications. However, they suffer from lower angular resolution due to being captured by fisheye lenses with the same sensor size for capturing planar images. To solve the above issues, we propose a two-stage framework for 360{\deg} omnidirectional image superresolution. The first stage employs two branches: model A, which incorporates omnidirectional position-aware deformable blocks (OPDB) and Fourier upsampling, and model B, which adds a spatial frequency fusion module (SFF) to model A. Model A aims to enhance the feature extraction ability of 360{\deg} image positional information, while Model B further focuses on the high-frequency information of 360{\deg} images. The second stage performs same-resolution enhancement based on the structure of model A with a pixel unshuffle operation. In addition, we collected data from YouTube to improve the fitting ability of the transformer, and created pseudo low-resolution images using a degradation network. Our proposed method achieves superior performance and wins the NTIRE 2023 challenge of 360{\deg} omnidirectional image super-resolution.
更多查看译文
关键词
network,position-aware,super-resolution
AI 理解论文
溯源树
样例
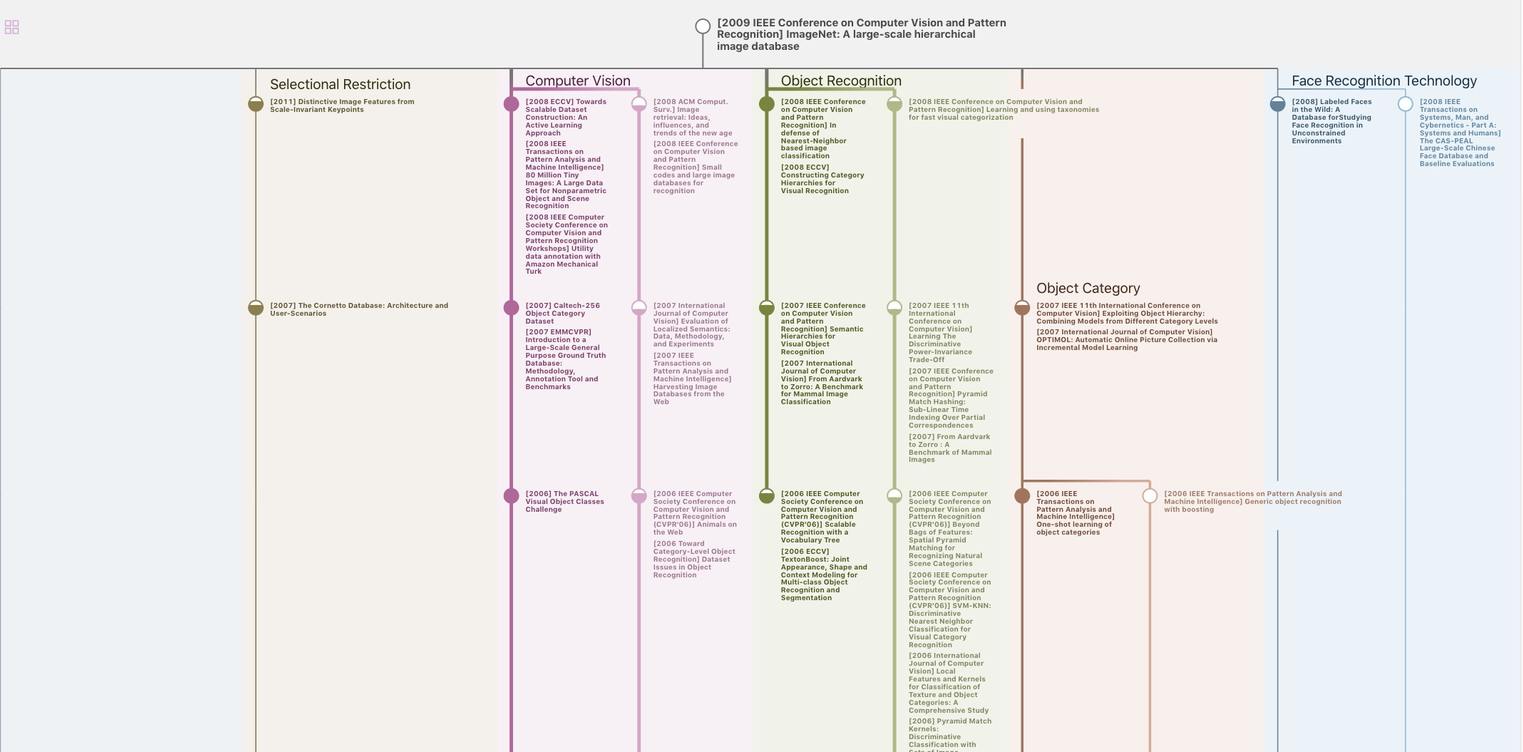
生成溯源树,研究论文发展脉络
Chat Paper
正在生成论文摘要