Dissecting Psychiatric Heterogeneity and Comorbidity with Core Region-Based Machine Learning
Neuroscience bulletin(2023)
摘要
Machine learning approaches are increasingly being applied to neuroimaging data from patients with psychiatric disorders to extract brain-based features for diagnosis and prognosis. The goal of this review is to discuss recent practices for evaluating machine learning applications to obsessive-compulsive and related disorders and to advance a novel strategy of building machine learning models based on a set of core brain regions for better performance, interpretability, and generalizability. Specifically, we argue that a core set of co-altered brain regions (namely ‘core regions’) comprising areas central to the underlying psychopathology enables the efficient construction of a predictive model to identify distinct symptom dimensions/clusters in individual patients. Hypothesis-driven and data-driven approaches are further introduced showing how core regions are identified from the entire brain. We demonstrate a broadly applicable roadmap for leveraging this core set-based strategy to accelerate the pursuit of neuroimaging-based markers for diagnosis and prognosis in a variety of psychiatric disorders.
更多查看译文
关键词
Core region,Machine learning,Magnetic resonance imaging,Neuroimaging-based diagnosis,Obsessive-compulsive disorder,Psychiatric disorders
AI 理解论文
溯源树
样例
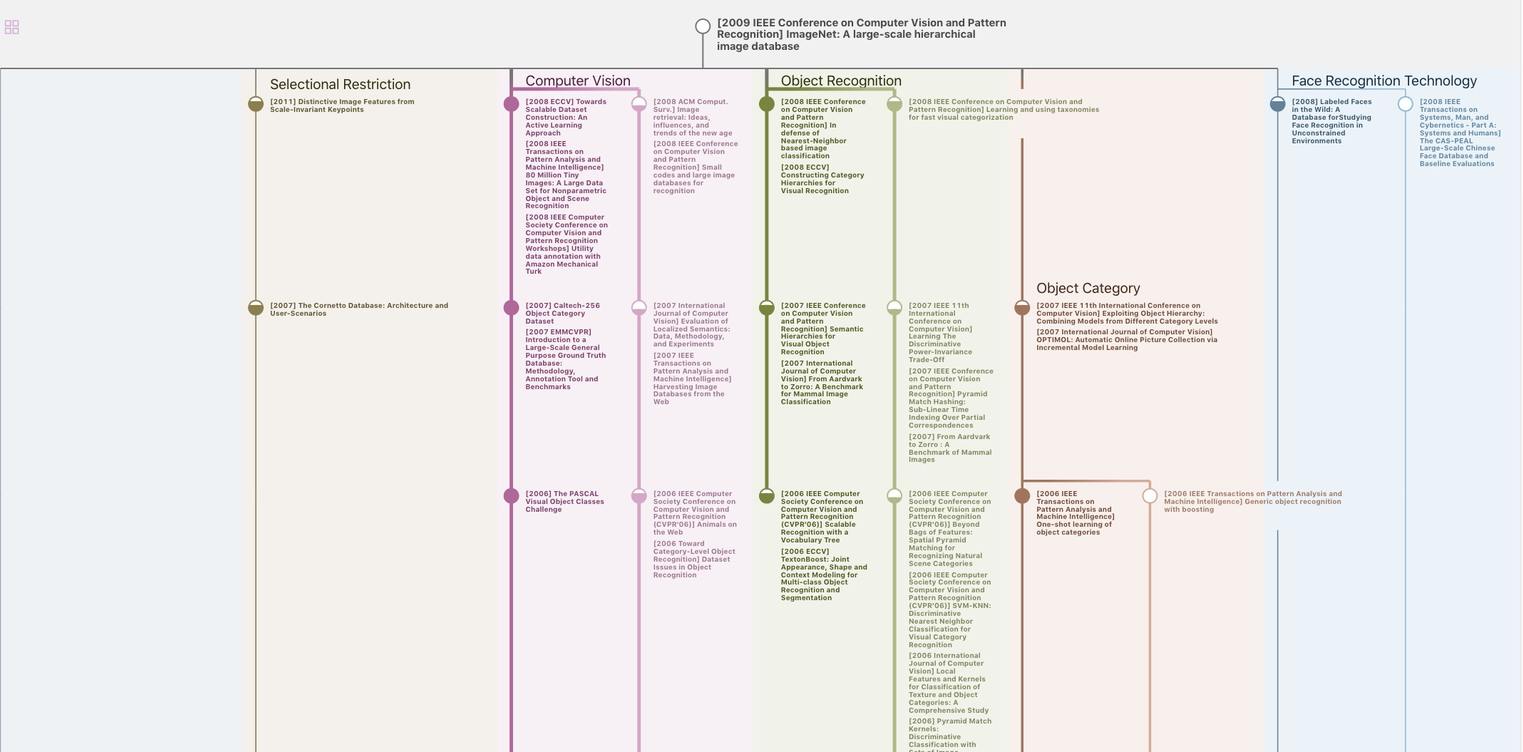
生成溯源树,研究论文发展脉络
Chat Paper
正在生成论文摘要