Reconstructing Turbulent Flows Using Physics-Aware Spatio-Temporal Dynamics and Test-Time Refinement
CoRR(2023)
摘要
Simulating turbulence is critical for many societally important applications in aerospace engineering, environmental science, the energy industry, and biomedicine. Large eddy simulation (LES) has been widely used as an alternative to direct numerical simulation (DNS) for simulating turbulent flows due to its reduced computational cost. However, LES is unable to capture all of the scales of turbulent transport accurately. Reconstructing DNS from low-resolution LES is critical for many scientific and engineering disciplines, but it poses many challenges to existing super-resolution methods due to the spatio-temporal complexity of turbulent flows. In this work, we propose a new physics-guided neural network for reconstructing the sequential DNS from low-resolution LES data. The proposed method leverages the partial differential equation that underlies the flow dynamics in the design of spatio-temporal model architecture. A degradation-based refinement method is also developed to enforce physical constraints and further reduce the accumulated reconstruction errors over long periods. The results on two different types of turbulent flow data confirm the superiority of the proposed method in reconstructing the high-resolution DNS data and preserving the physical characteristics of flow transport.
更多查看译文
关键词
turbulent flows,dynamics,refinement,physics-aware,spatio-temporal,test-time
AI 理解论文
溯源树
样例
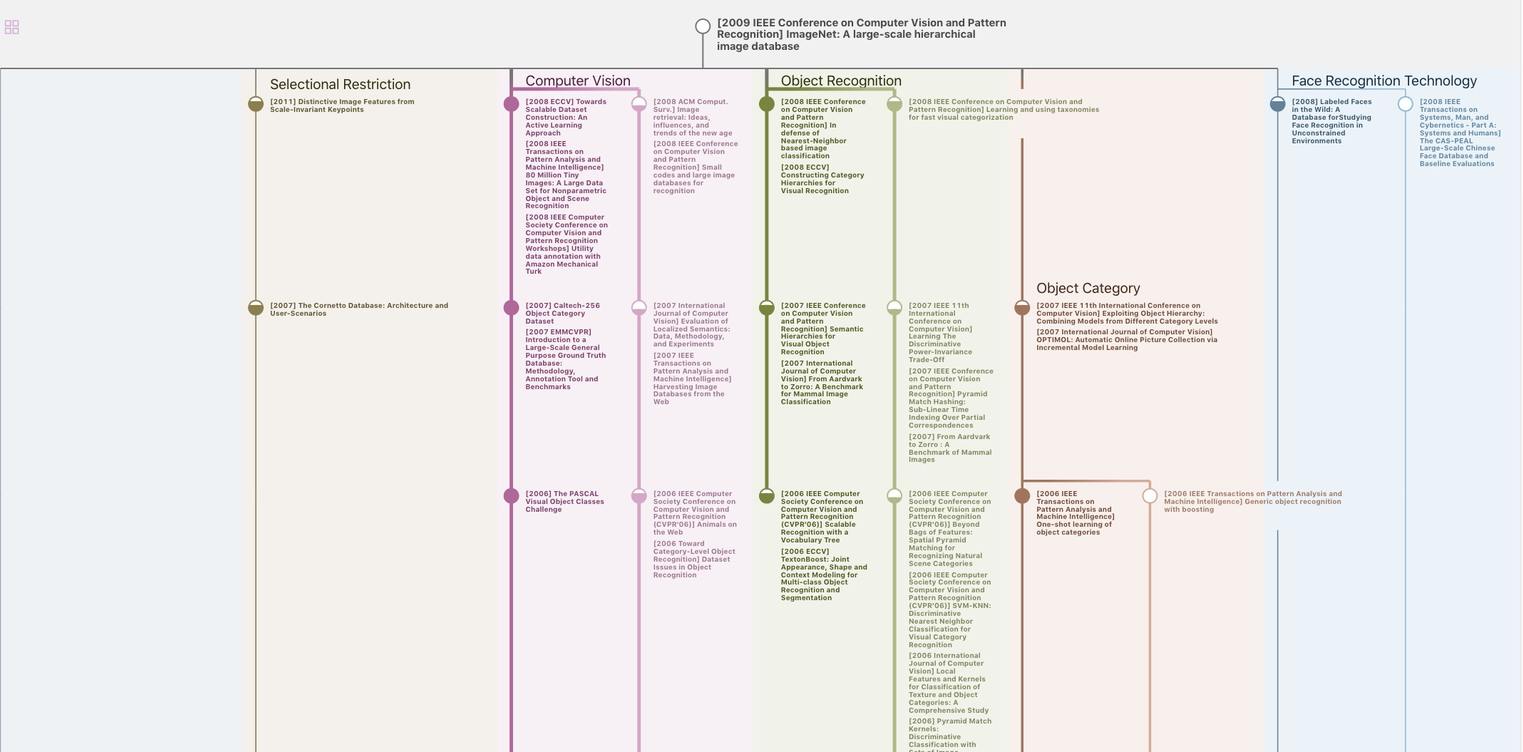
生成溯源树,研究论文发展脉络
Chat Paper
正在生成论文摘要