Reinforcement Learning with Knowledge Representation and Reasoning: A Brief Survey
CoRR(2023)
摘要
Reinforcement Learning(RL) has achieved tremendous development in recent years, but still faces significant obstacles in addressing complex real-life problems due to the issues of poor system generalization, low sample efficiency as well as safety and interpretability concerns. The core reason underlying such dilemmas can be attributed to the fact that most of the work has focused on the computational aspect of value functions or policies using a representational model to describe atomic components of rewards, states and actions etc, thus neglecting the rich high-level declarative domain knowledge of facts, relations and rules that can be either provided a priori or acquired through reasoning over time. Recently, there has been a rapidly growing interest in the use of Knowledge Representation and Reasoning(KRR) methods, usually using logical languages, to enable more abstract representation and efficient learning in RL. In this survey, we provide a preliminary overview on these endeavors that leverage the strengths of KRR to help solving various problems in RL, and discuss the challenging open problems and possible directions for future work in this area.
更多查看译文
关键词
knowledge representation,reinforcement,learning,reasoning
AI 理解论文
溯源树
样例
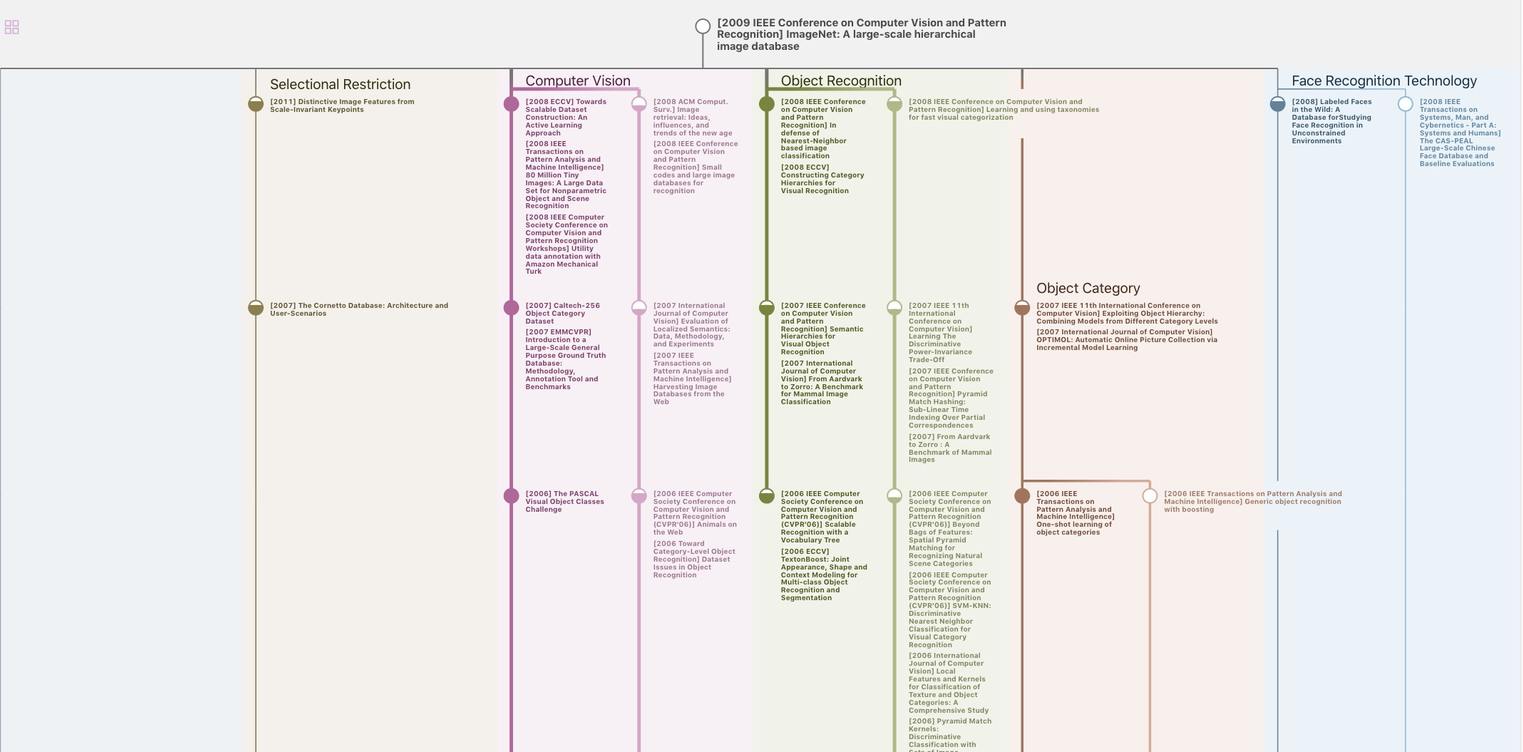
生成溯源树,研究论文发展脉络
Chat Paper
正在生成论文摘要