Triple Structural Information Modelling for Accurate, Explainable and Interactive Recommendation
PROCEEDINGS OF THE 46TH INTERNATIONAL ACM SIGIR CONFERENCE ON RESEARCH AND DEVELOPMENT IN INFORMATION RETRIEVAL, SIGIR 2023(2023)
摘要
In dynamic interaction graphs, user-item interactions usually follow heterogeneous patterns, represented by different structural information, such as user-item co-occurrence, sequential information of user interactions and the transition probabilities of item pairs. However, the existing methods cannot simultaneously leverage all three structural information, resulting in suboptimal performance. To this end, we propose TriSIM4Rec, a triple structural information modeling method for accurate, explainable and interactive recommendation on dynamic interaction graphs. Specifically, TriSIM4Rec consists of 1) a dynamic ideal low-pass graph filter to dynamically mine co-occurrence information in user-item interactions, which is implemented by incremental singular value decomposition (SVD); 2) a parameter-free attention module to capture sequential information of user interactions effectively and efficiently; and 3) an item transition matrix to store the transition probabilities of item pairs. Then, we fuse the predictions from the triple structural information sources to obtain the final recommendation results. By analyzing the relationship between the SVD-based and the recently emerging graph signal processing (GSP)-based collaborative filtering methods, we find that the essence of SVD is an ideal low-pass graph filter, so that the interest vector space in TriSIM4Rec can be extended to achieve explainable and interactive recommendation, making it possible for users to actively break through the information cocoons. Experiments on six public datasets demonstrated the effectiveness of TriSIM4Rec in accuracy, explainability and interactivity.
更多查看译文
关键词
recommendation system,singular value decomposition,graph filtering,user behavior modeling
AI 理解论文
溯源树
样例
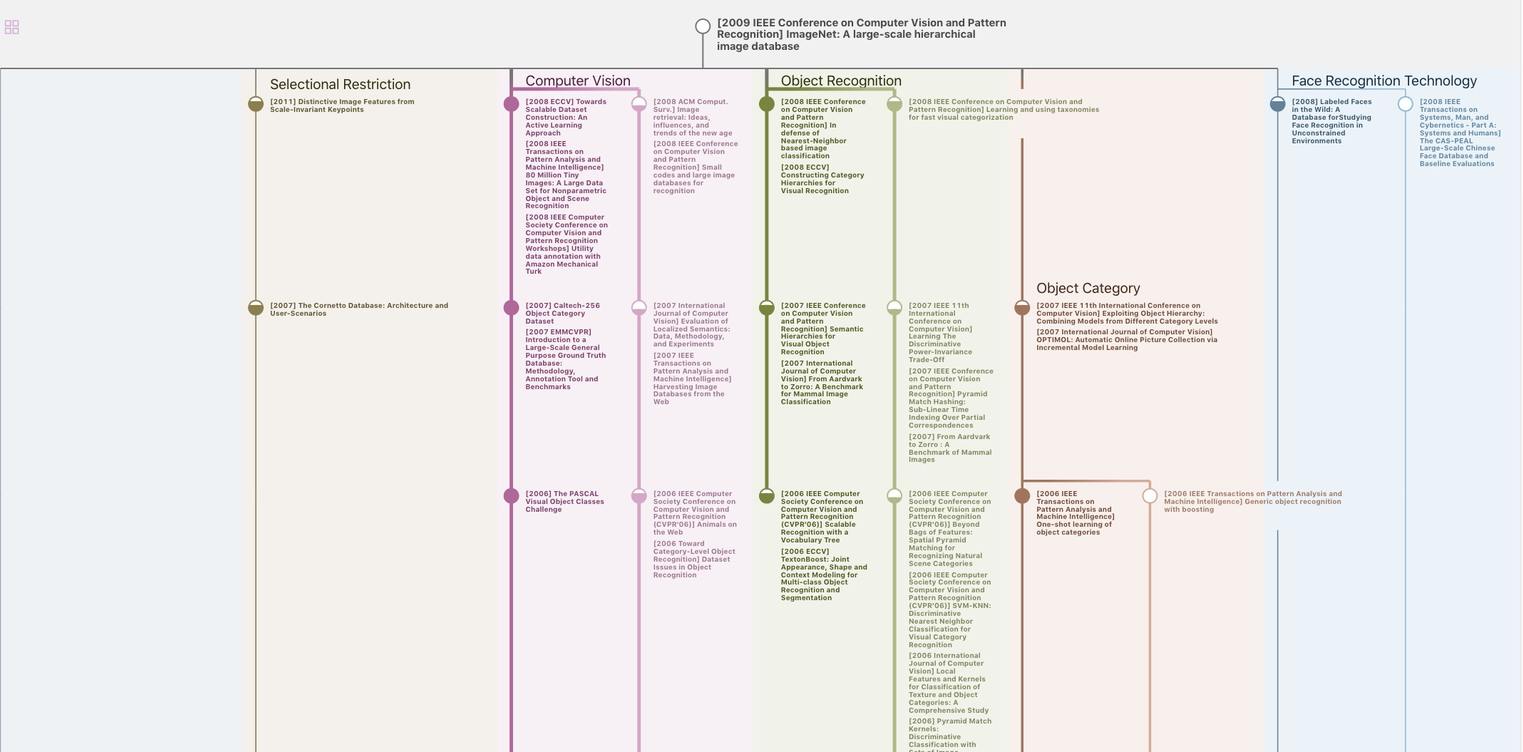
生成溯源树,研究论文发展脉络
Chat Paper
正在生成论文摘要