Learning Self-Supervised Representations for Label Efficient Cross-Domain Knowledge Transfer on Diabetic Retinopathy Fundus Images
CoRR(2023)
摘要
This work presents a novel label-efficient selfsupervised representation learning-based approach for classifying diabetic retinopathy (DR) images in cross-domain settings. Most of the existing DR image classification methods are based on supervised learning which requires a lot of time-consuming and expensive medical domain experts-annotated data for training. The proposed approach uses the prior learning from the source DR image dataset to classify images drawn from the target datasets. The image representations learned from the unlabeled source domain dataset through contrastive learning are used to classify DR images from the target domain dataset. Moreover, the proposed approach requires a few labeled images to perform successfully on DR image classification tasks in cross-domain settings. The proposed work experiments with four publicly available datasets: EyePACS, APTOS 2019, MESSIDOR-I, and Fundus Images for self-supervised representation learning-based DR image classification in cross-domain settings. The proposed method achieves state-of-the-art results on binary and multiclassification of DR images, even in cross-domain settings. The proposed method outperforms the existing DR image binary and multi-class classification methods proposed in the literature. The proposed method is also validated qualitatively using class activation maps, revealing that the method can learn explainable image representations. The source code and trained models are published on GitHub.
更多查看译文
关键词
Self-supervised representation learning,domain adaptation
AI 理解论文
溯源树
样例
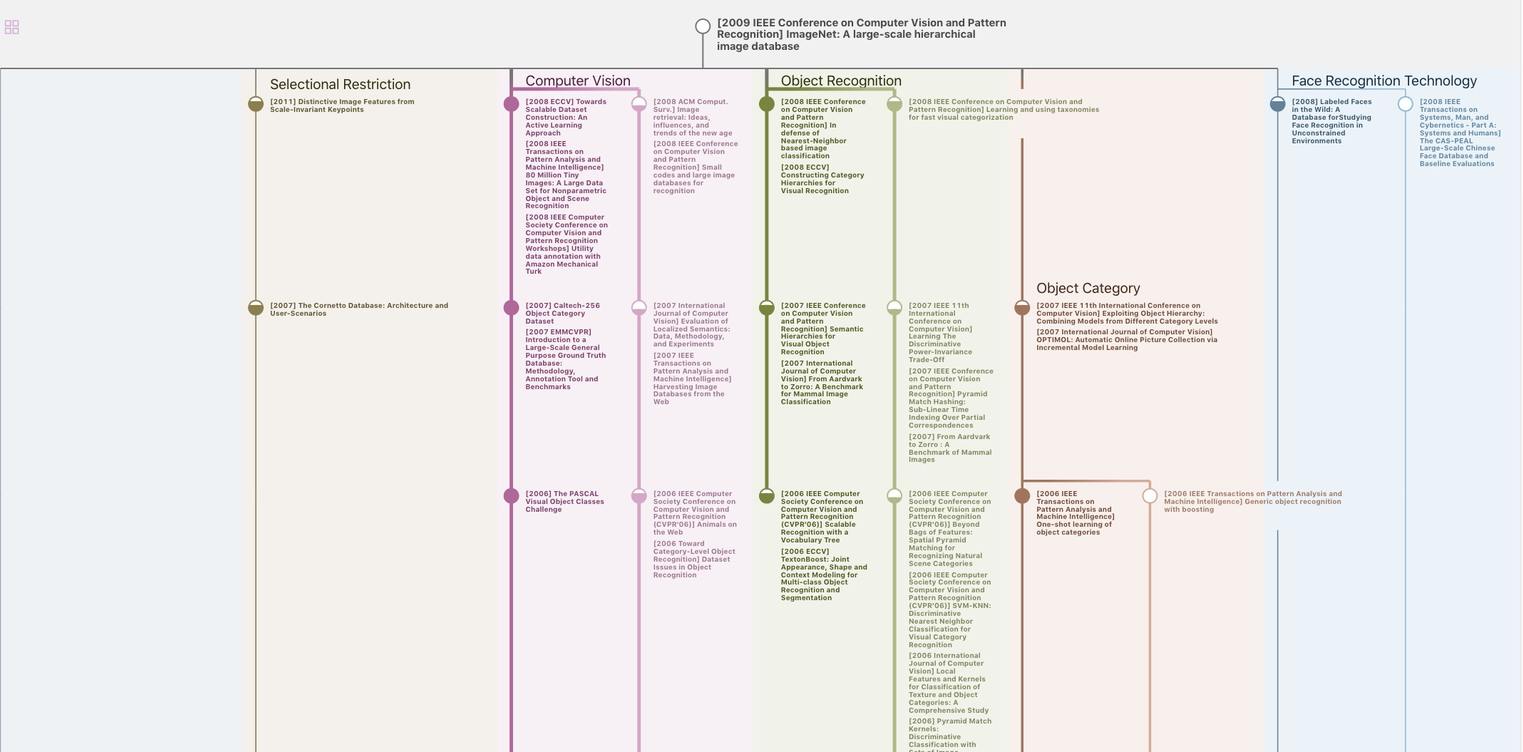
生成溯源树,研究论文发展脉络
Chat Paper
正在生成论文摘要