quEHRy: a question answering system to query electronic health records
JOURNAL OF THE AMERICAN MEDICAL INFORMATICS ASSOCIATION(2023)
摘要
Objective We propose a system, quEHRy, to retrieve precise, interpretable answers to natural language questions from structured data in electronic health records (EHRs). Materials and Methods We develop/synthesize the main components of quEHRy: concept normalization (MetaMap), time frame classification (new), semantic parsing (existing), visualization with question understanding (new), and query module for FHIR mapping/processing (new). We evaluate quEHRy on 2 clinical question answering (QA) datasets. We evaluate each component separately as well as holistically to gain deeper insights. We also conduct a thorough error analysis for a crucial subcomponent, medical concept normalization. Results Using gold concepts, the precision of quEHRy is 98.33% and 90.91% for the 2 datasets, while the overall accuracy was 97.41% and 87.75%. Precision was 94.03% and 87.79% even after employing an automated medical concept extraction system (MetaMap). Most incorrectly predicted medical concepts were broader in nature than gold-annotated concepts (representative of the ones present in EHRs), eg, Diabetes versus Diabetes Mellitus, Non-Insulin-Dependent. Discussion The primary performance barrier to deployment of the system is due to errors in medical concept extraction (a component not studied in this article), which affects the downstream generation of correct logical structures. This indicates the need to build QA-specific clinical concept normalizers that understand EHR context to extract the "relevant" medical concepts from questions. Conclusion We present an end-to-end QA system that allows information access from EHRs using natural language and returns an exact, verifiable answer. Our proposed system is high-precision and interpretable, checking off the requirements for clinical use.
更多查看译文
关键词
question answering,electronic health records,natural language processing,artificial intelligence,machine learning,FHIR
AI 理解论文
溯源树
样例
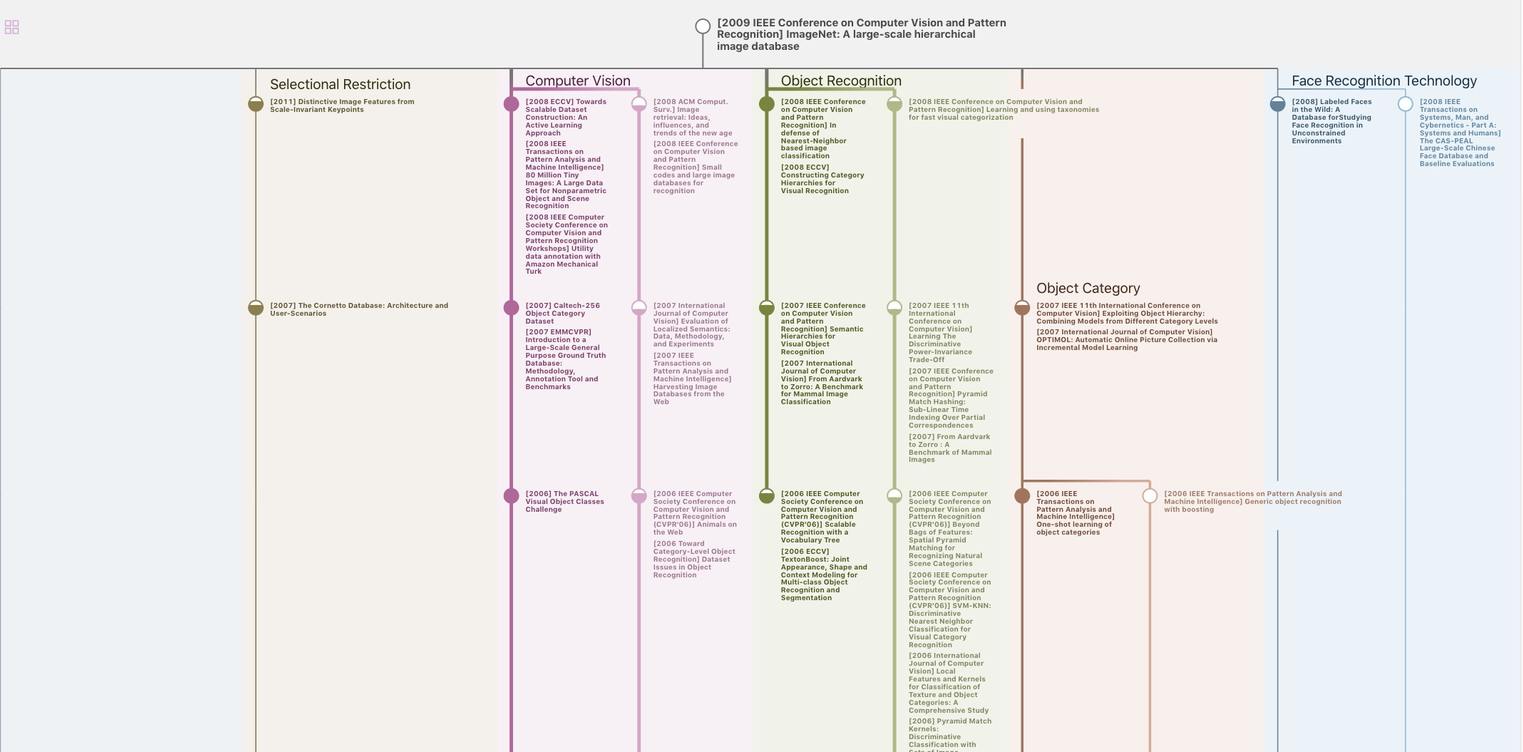
生成溯源树,研究论文发展脉络
Chat Paper
正在生成论文摘要