Weakly supervised spatial relation extraction from radiology reports
JAMIA Open(2023)
摘要
Objective Weak supervision holds significant promise to improve clinical natural language processing by leveraging domain resources and expertise instead of large manually annotated datasets alone. Here, our objective is to evaluate a weak supervision approach to extract spatial information from radiology reports. Materials and Methods Our weak supervision approach is based on data programming that uses rules (or labeling functions) relying on domain-specific dictionaries and radiology language characteristics to generate weak labels. The labels correspond to different spatial relations that are critical to understanding radiology reports. These weak labels are then used to fine-tune a pretrained Bidirectional Encoder Representations from Transformers (BERT) model. Results Our weakly supervised BERT model provided satisfactory results in extracting spatial relations without manual annotations for training (spatial trigger F1: 72.89, relation F1: 52.47). When this model is further fine-tuned on manual annotations (relation F1: 68.76), performance surpasses the fully supervised state-of-the-art. Discussion To our knowledge, this is the first work to automatically create detailed weak labels corresponding to radiological information of clinical significance. Our data programming approach is (1) adaptable as the labeling functions can be updated with relatively little manual effort to incorporate more variations in radiology language reporting formats and (2) generalizable as these functions can be applied across multiple radiology subdomains in most cases. Conclusions We demonstrate a weakly supervision model performs sufficiently well in identifying a variety of relations from radiology text without manual annotations, while exceeding state-of-the-art results when annotated data are available. Lay Summary Radiology reports contain important clinical information about patients (eg, findings, body locations). Oftentimes, this information is connected through spatial relations (eg, tumor is in the left lung). It is important to automatically capture such rich information to facilitate various clinical applications. However, developing deep learning-based natural language processing models for automatic extraction rely on manually labeled data for training that requires time and domain expertise. Such models are called fully supervised models. In this work, we proposed a weak supervision approach to automatically create training data to extract spatial information from radiology reports. This is based on data programming where we develop rules to automatically generate labels (known as weak labels). We further used this weakly labeled data to train a language model called Bidirectional Encoder Representations from Transformers (BERT) which performed sufficiently well when evaluated on an existing manually labeled dataset of 400 radiology reports. We also investigated the effect of adding some manual annotations in the training process (ie, training on weak labels followed by training on a small number of manually annotated labels) which outperformed the BERT model trained solely on manual annotations.
更多查看译文
关键词
information extraction,relation extraction,weak supervision,data programming,natural language processing,radiology report
AI 理解论文
溯源树
样例
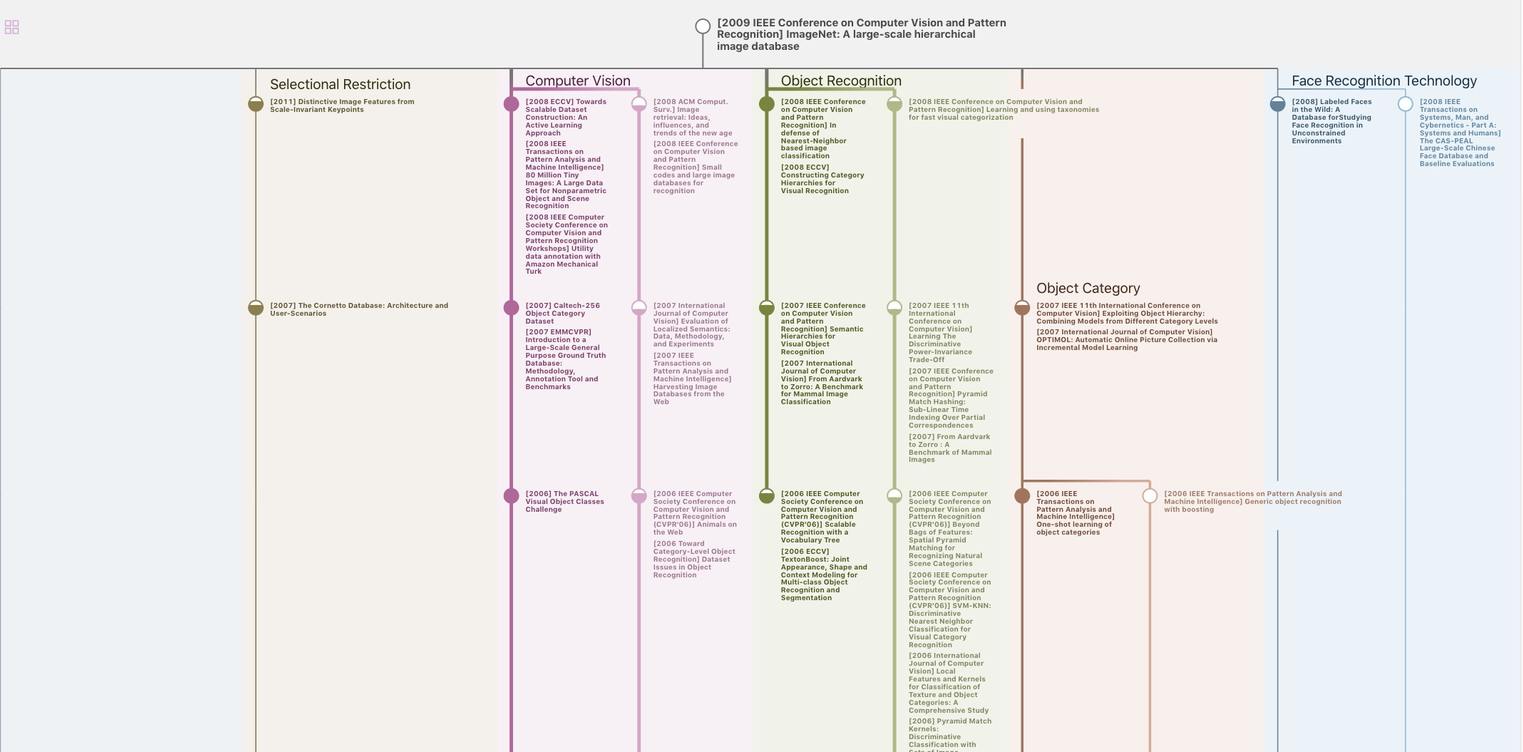
生成溯源树,研究论文发展脉络
Chat Paper
正在生成论文摘要