Multi-task Federated Learning Medical Analysis Algorithm Integrated Into Adapter
2023 IEEE 8th International Conference on Big Data Analytics (ICBDA)(2023)
摘要
Recently, the accumulated large amount of medical data has laid a solid foundation for the development of medical analysis. More and more data analysis methods have been proposed. But in this era, data security and data analysis capabilities have encountered significant challenges. First, medical data contains much information about the patient’s health status, disease progression, treatment, etc., and also involves much basic knowledge of patients. The adverse impact will be immeasurable if these data are attacked and leaked. Second, Data analysis capabilities are limited by security rules and cannot be improved. We propose a multi-task personalized clinical evidence-based analysis algorithm integrated into the task adapter, built with the federated learning architecture as the underlying architecture. We conduct extensive experiments on public datasets. The effect gap between our proposed model and the traditional central training model can be controlled by 5% for all medical tasks, which indicates the clinical evidence-based analysis algorithm based on the federated learning framework can achieve the same effect of approaching centralized training without data leakage. It will make Multi-task medical joint training possible.
更多查看译文
关键词
federated learning,adapter,multi-task,medical analysis
AI 理解论文
溯源树
样例
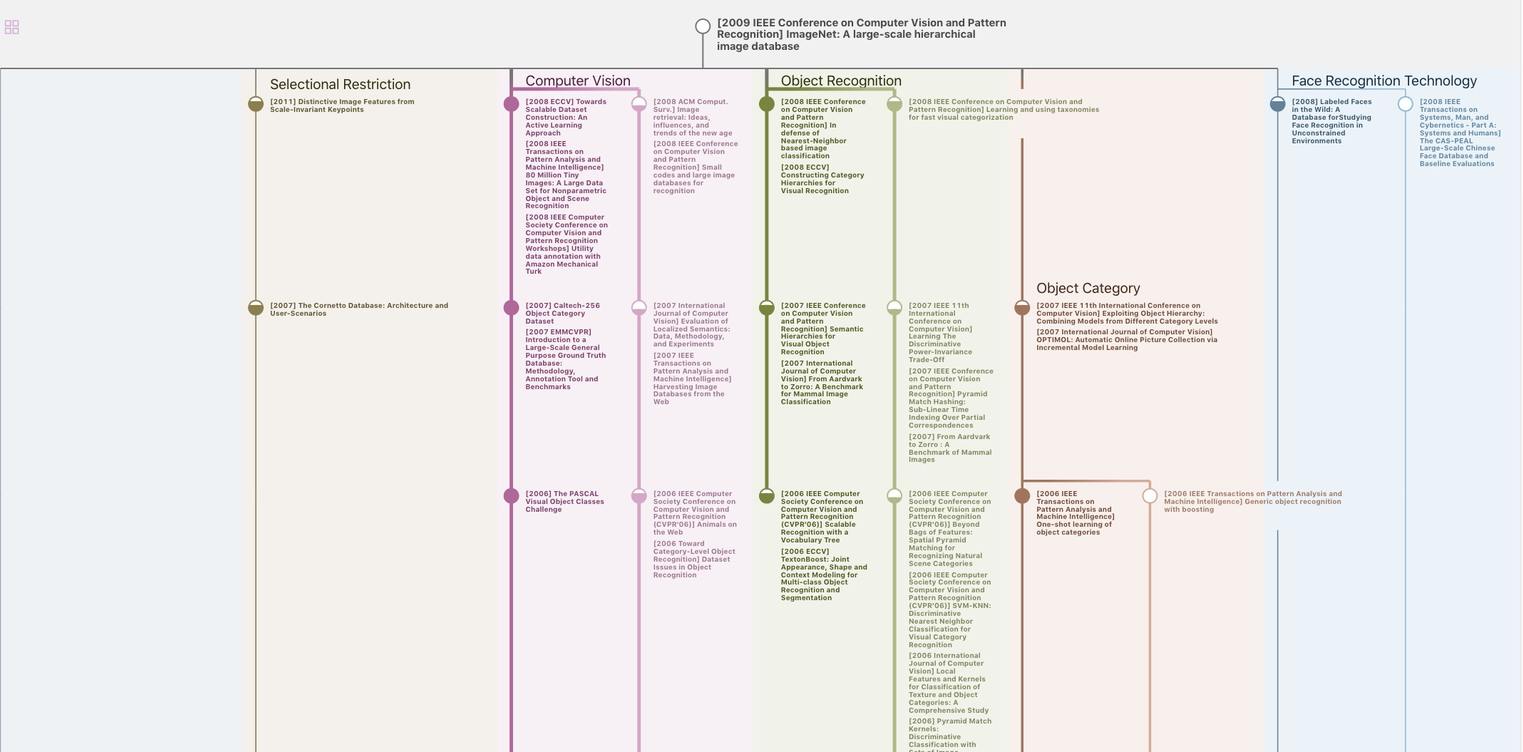
生成溯源树,研究论文发展脉络
Chat Paper
正在生成论文摘要