TT-Net: Tensorized Transformer Network for 3D medical image segmentation.
Computerized medical imaging and graphics : the official journal of the Computerized Medical Imaging Society(2023)
摘要
Accurate segmentation of organs, tissues and lesions is essential for computer-assisted diagnosis. Previous works have achieved success in the field of automatic segmentation. However, there exists two limitations. (1) They are remain challenged by complex conditions, such as segmentation target is variable in location, size and shape, especially for different imaging modalities. (2) Existing transformer-based networks suffer from a high parametric complexity. To solve these limitations, we propose a new Tensorized Transformer Network (TT-Net). In this paper, (1) Multi-scale transformer with layers-fusion is proposed to faithfully capture context interaction information. (2) Cross Shared Attention (CSA) module that based on pHash similarity fusion (pSF) is well-designed to extract the global multi-variate dependency features. (3) Tensorized Self-Attention (TSA) module is proposed to deal with the large number of parameters, which can also be easily embedded into other models. In addition, TT-Net gains a good explainability through visualizing the transformer layers. The proposed method is evaluated on three widely accepted public datasets and one clinical dataset, which contains different imaging modalities. Comprehensive results show that TT-Net outperforms other state-of-the-art methods for the four different segmentation tasks. Besides, the compression module which can be easily embedded into other transformer-based methods achieves lower computation with comparable segmentation performance.
更多查看译文
关键词
Automatic segmentation,Explainability,Multi-scale,Tensorized Transformer
AI 理解论文
溯源树
样例
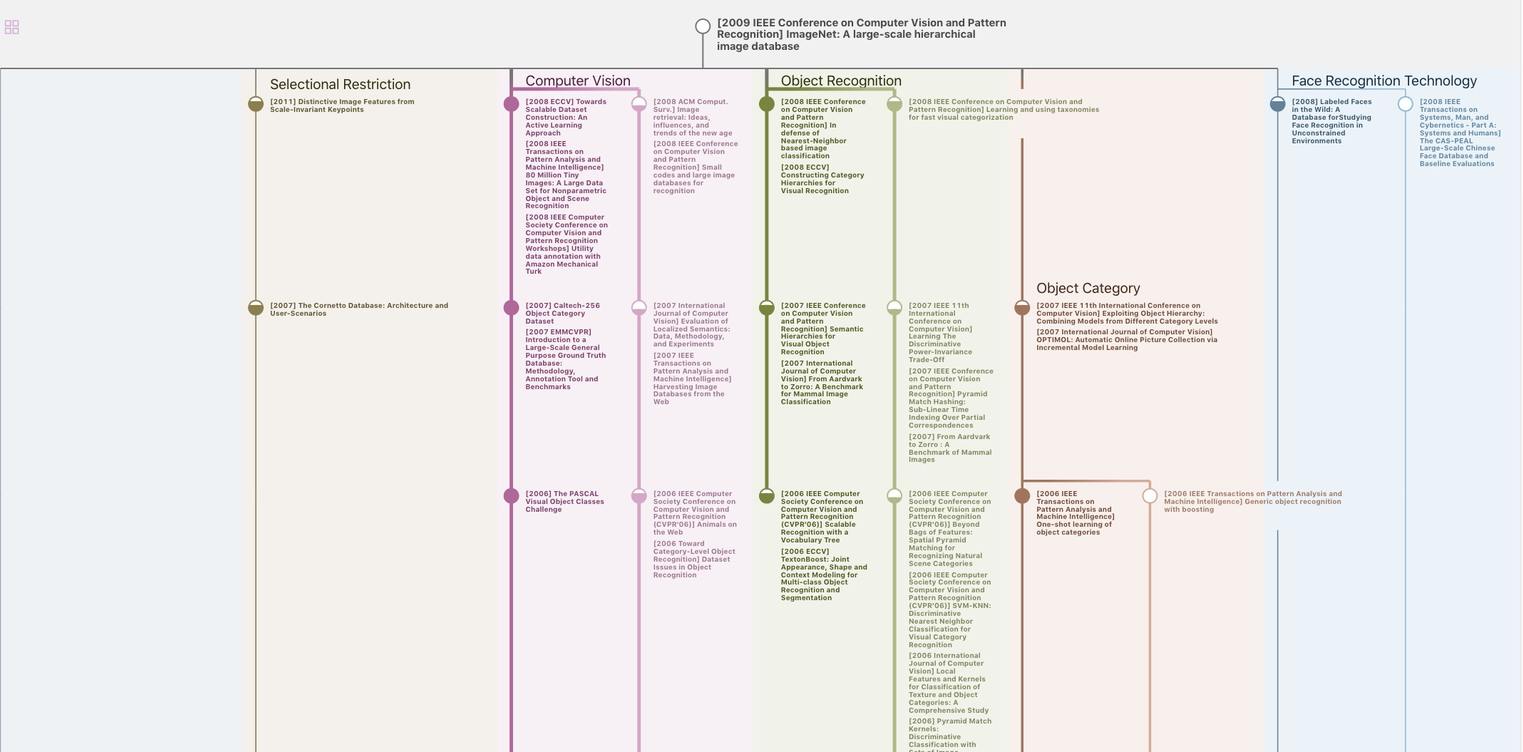
生成溯源树,研究论文发展脉络
Chat Paper
正在生成论文摘要