Kernel Learning by quantum annealer
arXiv (Cornell University)(2023)
摘要
The Boltzmann machine is one of the various applications using quantum annealer. We propose an application of the Boltzmann machine to the kernel matrix used in various machine-learning techniques. We focus on the fact that shift-invariant kernel functions can be expressed in terms of the expected value of a spectral distribution by the Fourier transformation. Using this transformation, random Fourier feature (RFF) samples the frequencies and approximates the kernel function. In this paper, furthermore, we propose a method to obtain a spectral distribution suitable for the data using a Boltzmann machine. As a result, we show that the prediction accuracy is comparable to that of the method using the Gaussian distribution. We also show that it is possible to create a spectral distribution that could not be feasible with the Gaussian distribution.
更多查看译文
关键词
quantum annealer,learning
AI 理解论文
溯源树
样例
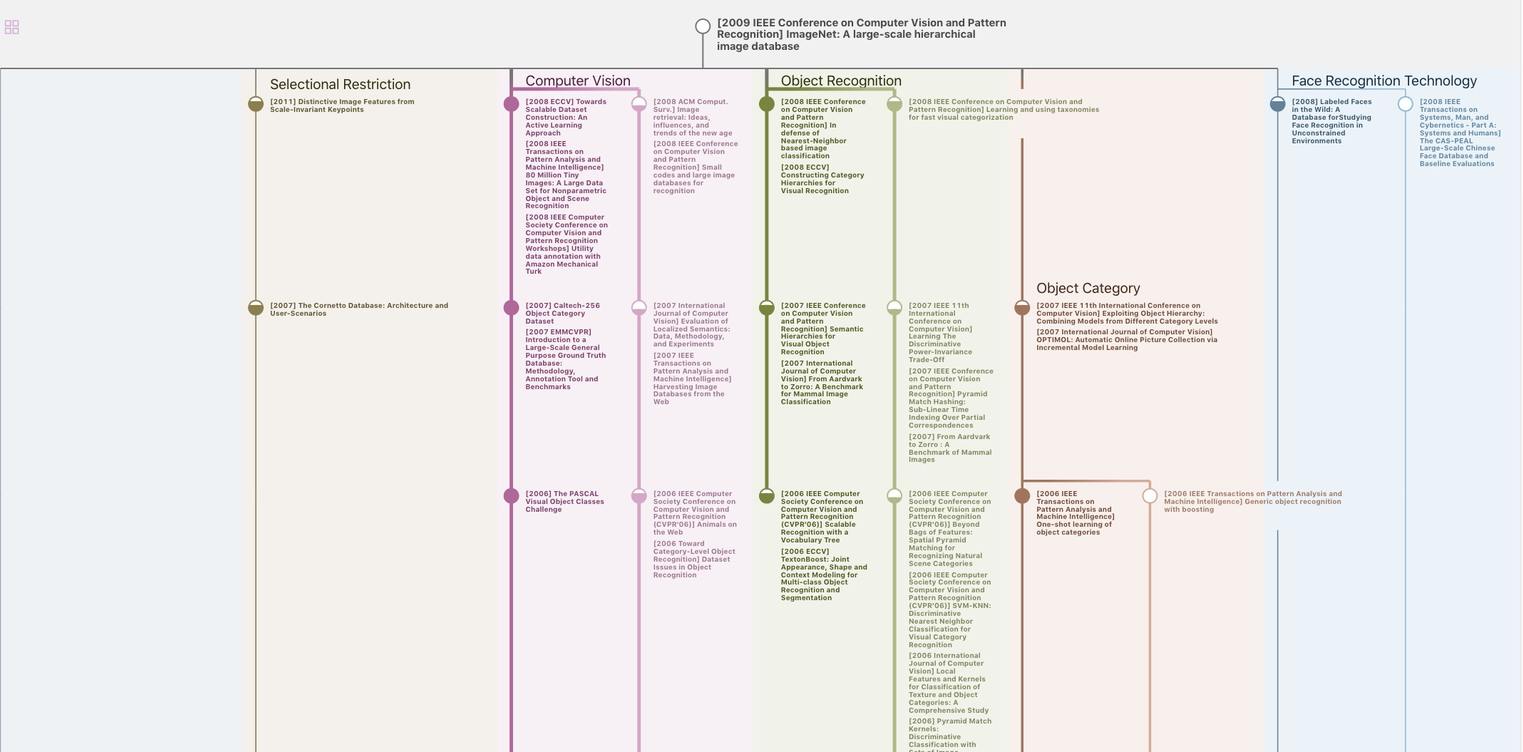
生成溯源树,研究论文发展脉络
Chat Paper
正在生成论文摘要