Torque-Based Deep Reinforcement Learning for Task-and-Robot Agnostic Learning on Bipedal Robots Using Sim-to-Real Transfer
IEEE ROBOTICS AND AUTOMATION LETTERS(2023)
摘要
In this letter, we review the question of which action space is best suited for controlling a real biped robot in combination with Sim2Real training. Position control has been popular as it has been shown to be more sample efficient and intuitive to combine with other planning algorithms. However, for position control, gain tuning is required to achieve the best possible policy performance. We show that, instead, using a torque-based action space enables task-and-robot agnostic learning with less parameter tuning and mitigates the sim-to-reality gap by taking advantage of torque control's inherent compliance. Also, we accelerate the torque-based-policy training process by pre-training the policy to remain upright by compensating for gravity. The letter showcases the first successful sim-to-real transfer of a torque-based deep reinforcement learning policy on a real human-sized biped robot.
更多查看译文
关键词
Reinforcement learning,humanoid and bipedal locomotion,torque-based control
AI 理解论文
溯源树
样例
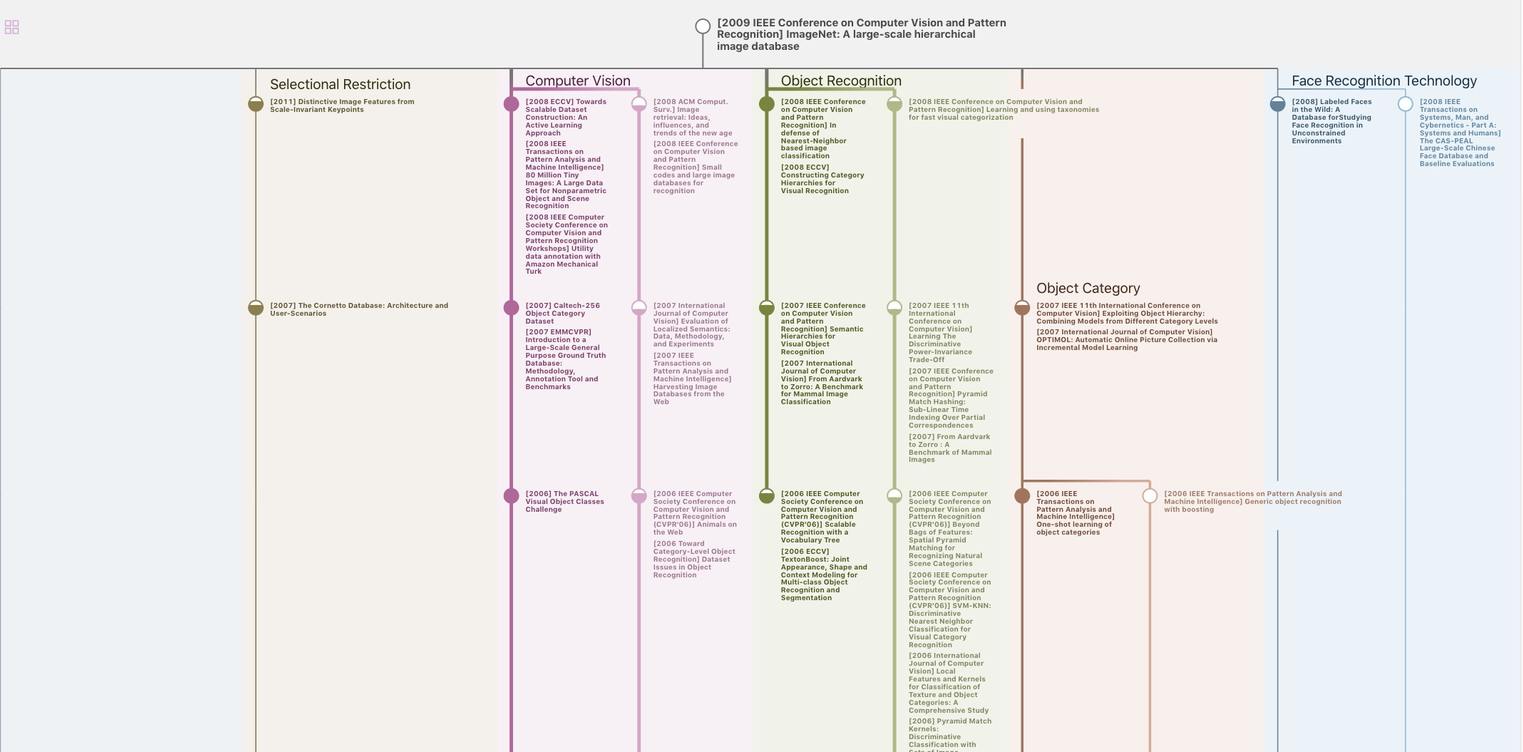
生成溯源树,研究论文发展脉络
Chat Paper
正在生成论文摘要