Minimax Signal Detection in Sparse Additive Models
arXiv (Cornell University)(2023)
摘要
Sparse additive models are an attractive choice in circumstances calling for modelling flexibility in the face of high dimensionality. We study the signal detection problem and establish the minimax separation rate for the detection of a sparse additive signal. Our result is nonasymptotic and applicable to the general case where the univariate component functions belong to a generic reproducing kernel Hilbert space. Unlike the estimation theory, the minimax separation rate reveals a nontrivial interaction between sparsity and the choice of function space. We also investigate adaptation to sparsity and establish an adaptive testing rate for a generic function space; adaptation is possible in some spaces while others impose an unavoidable cost. Finally, adaptation to both sparsity and smoothness is studied in the setting of Sobolev space, and we correct some existing claims in the literature.
更多查看译文
关键词
sparse additive models,detection
AI 理解论文
溯源树
样例
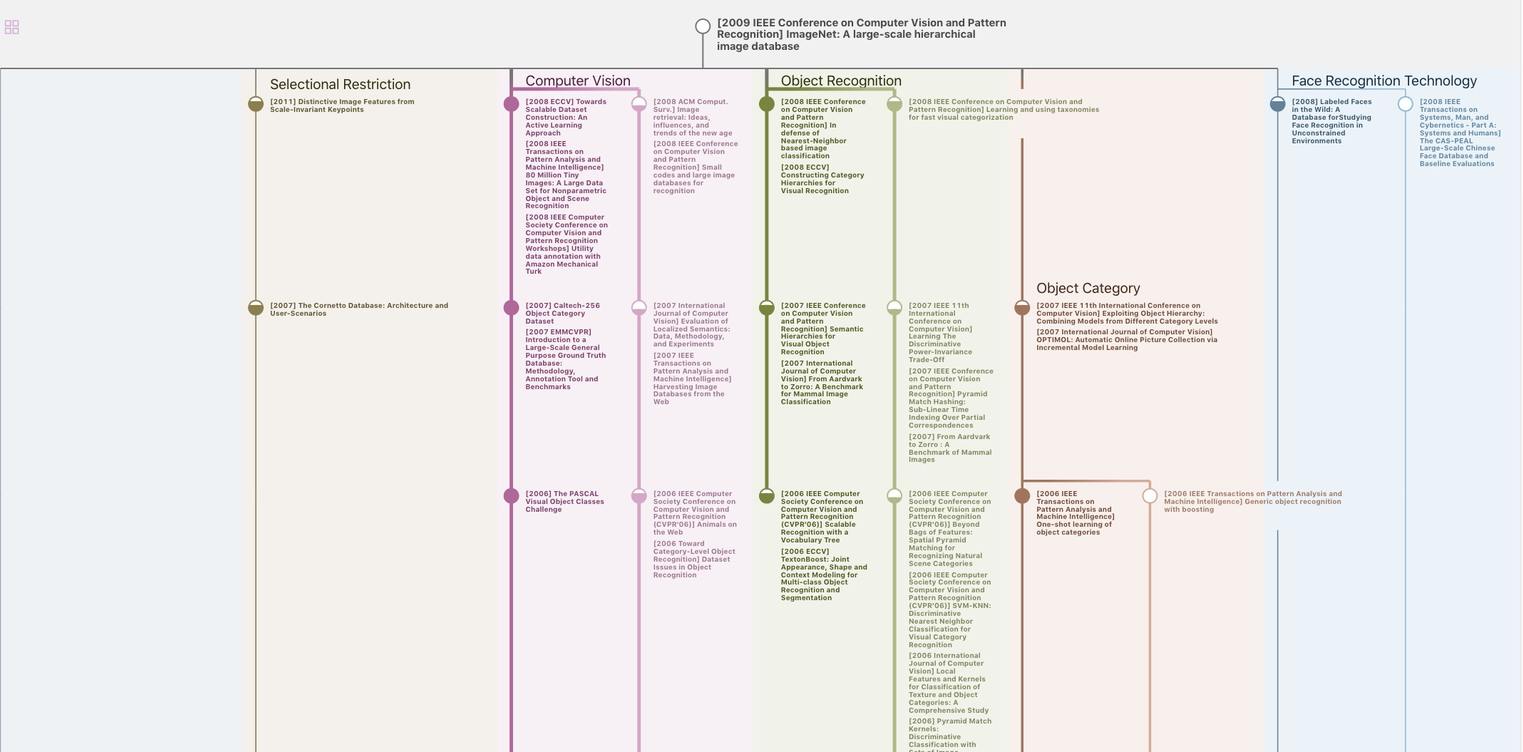
生成溯源树,研究论文发展脉络
Chat Paper
正在生成论文摘要