GTRNet: a graph-based table reconstructed network
2022 IEEE 34th International Conference on Tools with Artificial Intelligence (ICTAI)(2022)
摘要
Tabular data, with an exceedingly effective data structure, can give us a more intuitive visual display. To under-stand well and make use of the spatial and logic dependencies of it, we propose an end-to-end, graph-based table reconstructed network, namely GTRNet, in this paper. Our model works differently from most existing models which treat tables as either a markup sequence problem or a graph structure of rows and columns. It can utilize a table as input and extract its features in the text, image and position coordinate to predict the dependencies of the text instances and well distinguish the spatial relationship to infer whether multiple text segments belong to the same merged cell. Optimized along with this network, we can then restore the structure of this table. Moreover, we also create a new Chinese benchmark dataset GraphTable for this task to tackle complex challenges on the table. The competitive results on ICDAR-2013, GraphTable, SciTSR and FinTab benchmarks further confirm the great effectiveness of GTRN et.
更多查看译文
关键词
table recognition,spatial dependency,logic dependency,graph neural network,Chinese dataset
AI 理解论文
溯源树
样例
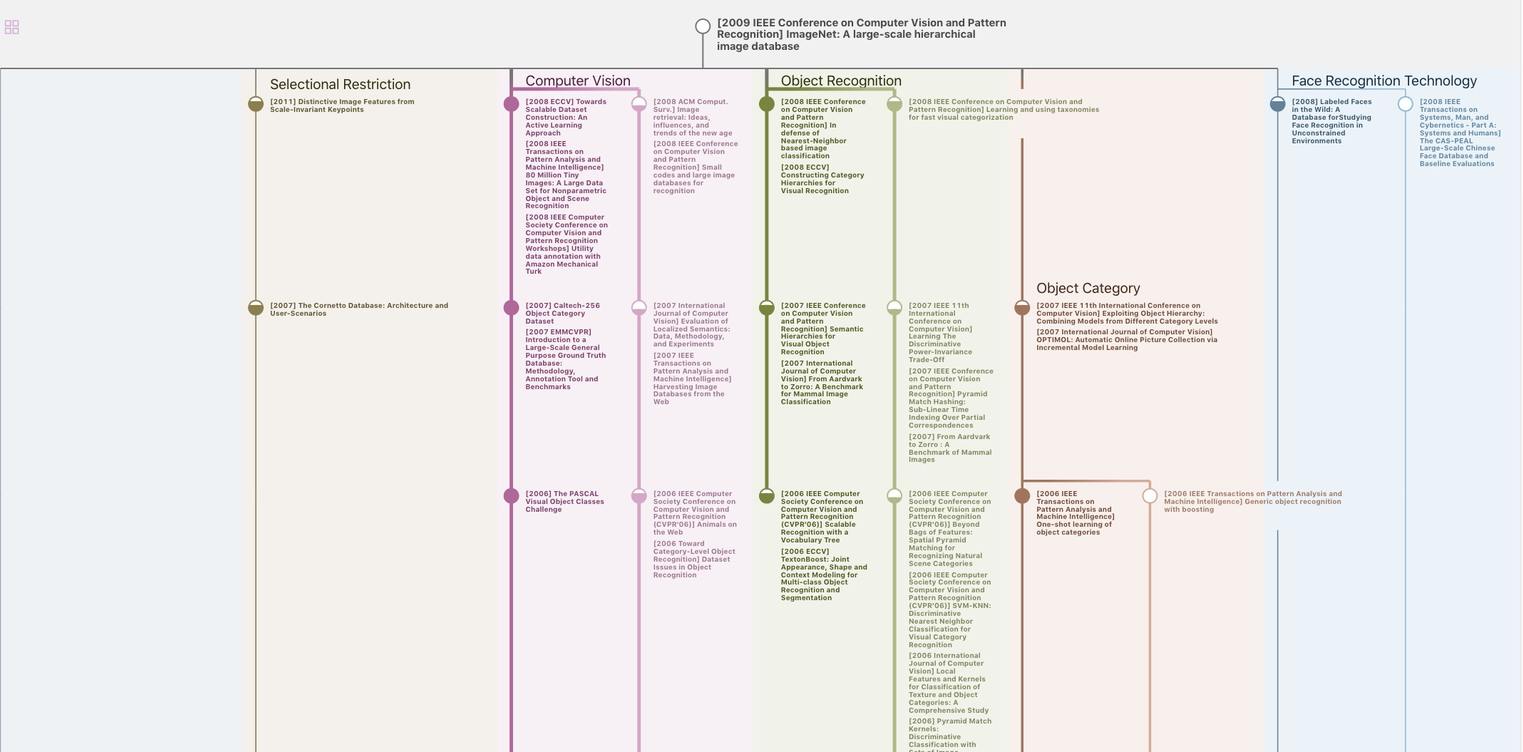
生成溯源树,研究论文发展脉络
Chat Paper
正在生成论文摘要