GlobalMind: Global multi-head interactive self-attention network for hyperspectral change detection
ISPRS Journal of Photogrammetry and Remote Sensing(2024)
摘要
High spectral resolution imagery of the Earth’s surface enables users to monitor changes over time in fine- grained scale, playing an increasingly important role in agriculture, defense, and emergency response. However, most current algorithms are still confined to describing local features and fail to incorporate a global perspective, which limits their ability to capture interactions between global features, thus usually resulting in incomplete change regions. In this paper, we proposed a Global Multi-head INteractive self-attention change Detection network (GlobalMind) to explore the implicit correlation between different surface objects and variant land cover transformations, acquiring a comprehensive understanding of the data and accurate change detection result. Firstly, a simple but effective Global Axial Segmentation (GAS) strategy is designed to expand the self-attention computation along the row space or column space of hyperspectral images, allowing the global connection with high efficiency. Secondly, with GAS, the global spatial multi-head interactive self-attention (GlobalM) module is crafted to mine the abundant spatial-spectral feature involving potential correlations between the ground objects from the entire rich and complex hyperspectral space. Moreover, to acquire the accurate and complete cross-temporal changes, we devise a global temporal interactive multi-head self-attention (GlobalD) module which incorporates the relevance and variation of bi-temporal spatial-spectral features, deriving the integrate potential same kind of changes in the local and global range with the combination of GAS. A new and challenging hyperspectral change detection dataset is designed for comparison of different approaches. We perform extensive experiments on six real hyperspectral datasets, and our method outperforms the state-of-the-art algorithms with high accuracy and efficiency.
更多查看译文
关键词
Hyperspectral change detection,Transformer,Global spatial correlation,Cross-temporal relevance,Self-attention
AI 理解论文
溯源树
样例
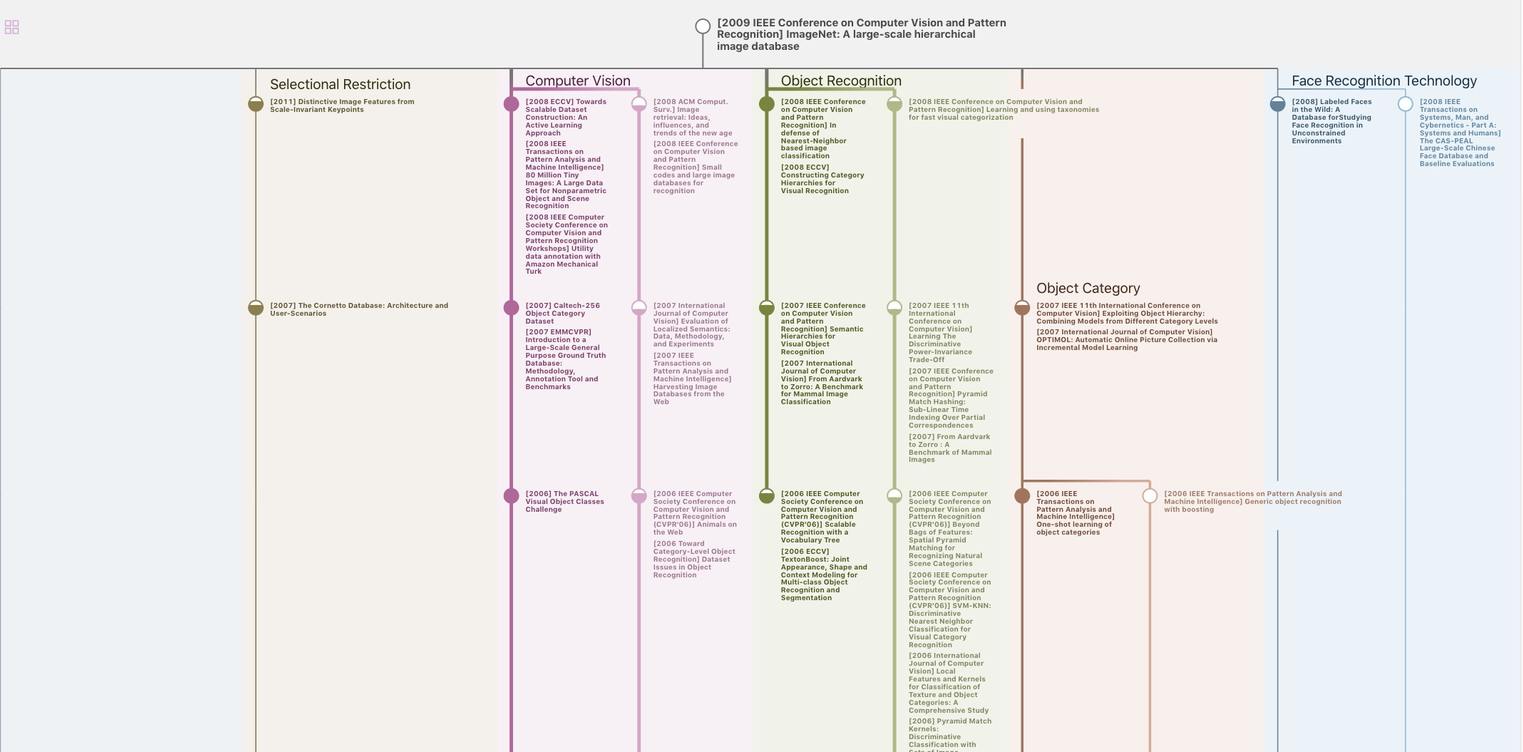
生成溯源树,研究论文发展脉络
Chat Paper
正在生成论文摘要