Nested physics-informed neural network for analysis of transient flows in natural gas pipelines
Engineering Applications of Artificial Intelligence(2023)
摘要
Natural gas pipeline systems are commonly designed under the assumption of constant supply and demand flow conditions. This is while gas flows are transient because of the compression stations, presence of gas storage facilities and fluctuating supply and consumer demands. Analyzing such transient flows substantially benefits design, control, and monitoring of natural gas pipeline systems. The nonlinear partial differential equations describing the physics of transient flows in pipelines are solved using conventional methods, which are computationally demanding especially for uncertainty quantification purposes where many simulations are required. In this study, we propose an alternative physics-informed neural network (PINN) framework for the transient analysis of pipeline networks that can perform transient flow analysis in natural gas pipelines that the original PINNs cannot solve due to the high complexity of the problem. We propose a nested structure for the PINNs with a loss model that greatly reduces the number of tasks in the emergent complex multi-task learning process. We also integrate an adaptive weights approach that tackles the imbalanced gradients caused by the extremely large coefficients in the equations of the natural gas pipeline problem. The proposed framework, for the first time, can produce accurate results for the complex natural gas pipeline network problem using PINNs. Furthermore, we investigate the parameterization of the nested PINNs as a surrogate model for the natural gas pipeline system. With merely 26% more training costs, the surrogate model can perform the transient flow analysis given thousands of realizations of parameters in a split millisecond, while the costs of performing the same simulations using conventional methods can be prohibitively expensive. This can greatly boost the efficiency of complex many-query analyses such as sensitivity analysis, uncertainty propagation and design optimization.
更多查看译文
关键词
Reliability analysis,Surrogate modeling,Adaptive kriging,Gaussian process,Error-based learning function
AI 理解论文
溯源树
样例
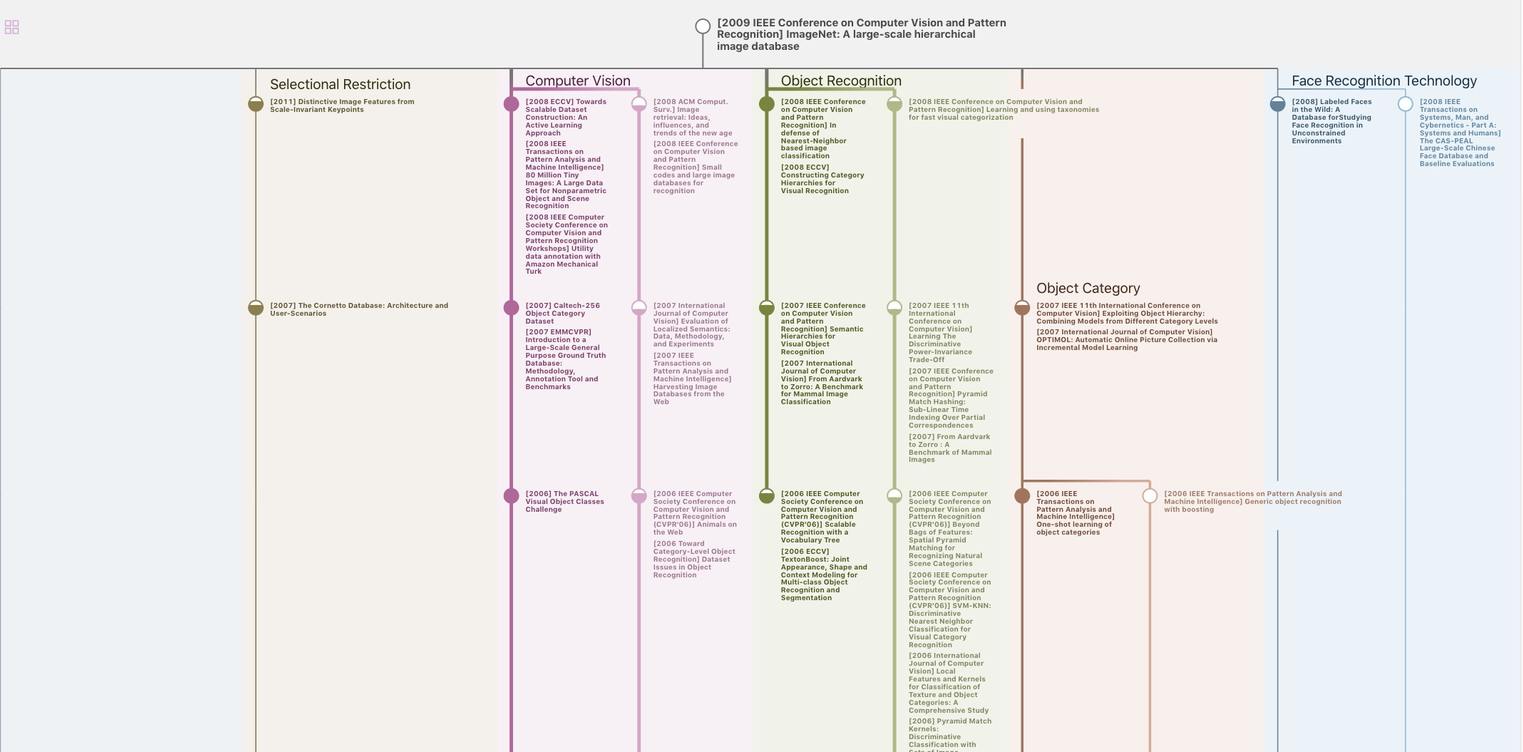
生成溯源树,研究论文发展脉络
Chat Paper
正在生成论文摘要