The Impact of Frame-Dropping on Performance and Energy Consumption for Multi-Object Tracking
CoRR(2023)
摘要
The safety of automated vehicles (AVs) relies on the representation of their environment. Consequently, state-of-the-art AVs employ potent sensor systems to achieve the best possible environment representation at all times. Although these high-performing systems achieve impressive results, they induce significant requirements for the processing capabilities of an AV's computational hardware components and their energy consumption. To enable a dynamic adaptation of such perception systems based on the situational perception requirements, we introduce a model-agnostic method for the scalable employment of single-frame object detection models using frame-dropping in tracking-by-detection systems. We evaluate our approach on the KITTI 3D Tracking Benchmark, showing that significant energy savings can be achieved at acceptable performance degradation, reaching up to 28% reduction of energy consumption at a performance decline of 6.6% in HOTA score.
更多查看译文
关键词
acceptable performance degradation,automated vehicles,AV computational hardware components,dynamic adaptation,energy consumption,environment representation,frame-dropping,KITTI 3D tracking benchmark,model-agnostic method,multiobject tracking,perception systems,performance decline,potent sensor systems,scalable employment,single-frame object detection models,situational perception requirements,state-of-the-art AV,tracking-by-detection systems
AI 理解论文
溯源树
样例
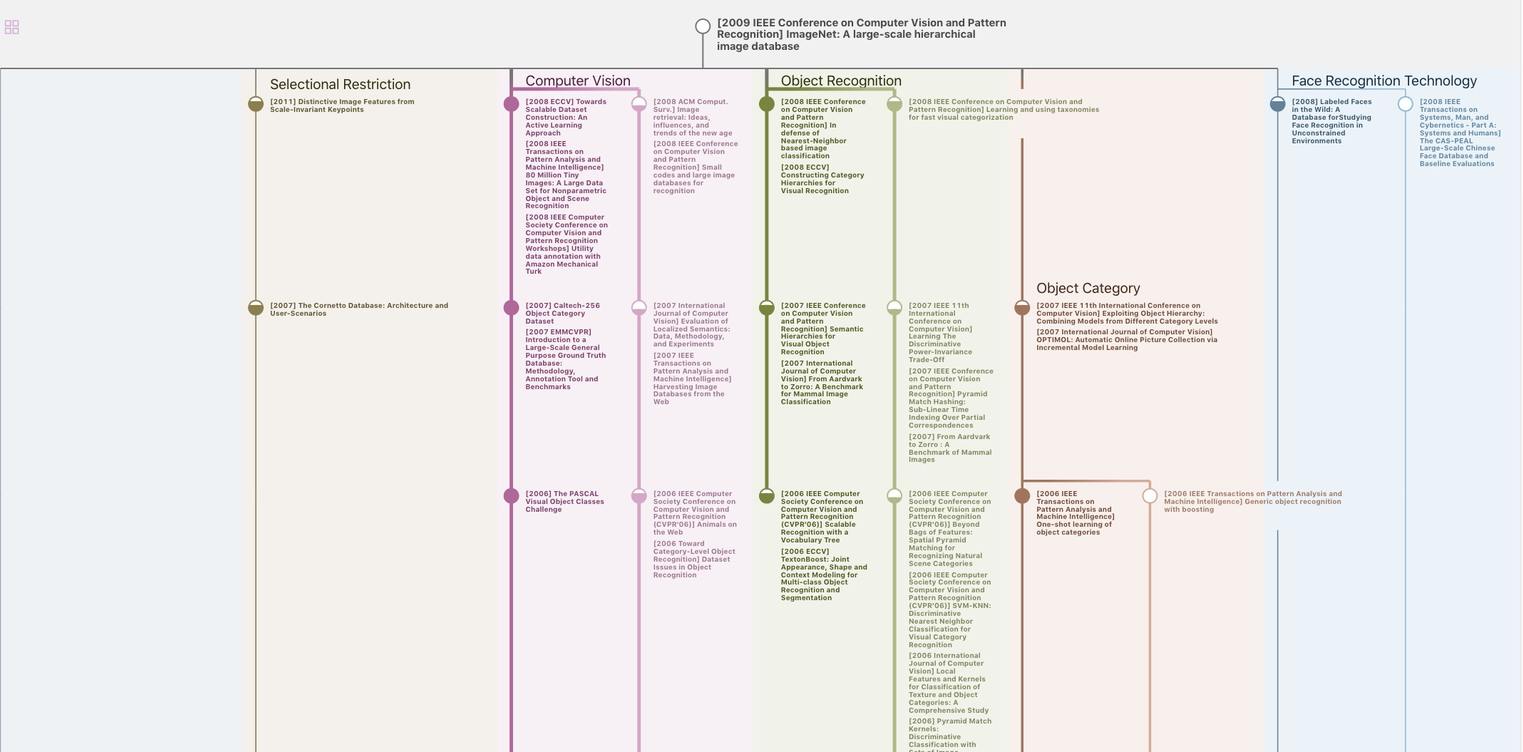
生成溯源树,研究论文发展脉络
Chat Paper
正在生成论文摘要