Non-exemplar Class-incremental Learning by Random Auxiliary Classes Augmentation and Mixed Features
arXiv (Cornell University)(2023)
摘要
Non-exemplar class-incremental learning refers to classifying new and old classes without storing samples of old classes. Since only new class samples are available for optimization, it often occurs catastrophic forgetting of old knowledge. To alleviate this problem, many new methods are proposed such as model distillation, class augmentation. In this paper, we propose an effective non-exemplar method called RAMF consisting of Random Auxiliary classes augmentation and Mixed Feature. On the one hand, we design a novel random auxiliary classes augmentation method, where one augmentation is randomly selected from three augmentations and applied on the input to generate augmented samples and extra class labels. By extending data and label space, it allows the model to learn more diverse representations, which can prevent the model from being biased towards learning task-specific features. When learning new tasks, it will reduce the change of feature space and improve model generalization. On the other hand, we employ mixed feature to replace the new features since only using new feature to optimize the model will affect the representation that was previously embedded in the feature space. Instead, by mixing new and old features, old knowledge can be retained without increasing the computational complexity. Extensive experiments on three benchmarks demonstrate the superiority of our approach, which outperforms the state-of-the-art non-exemplar methods and is comparable to high-performance replay-based methods.
更多查看译文
关键词
random auxiliary classes,learning,augmentation,non-exemplar,class-incremental
AI 理解论文
溯源树
样例
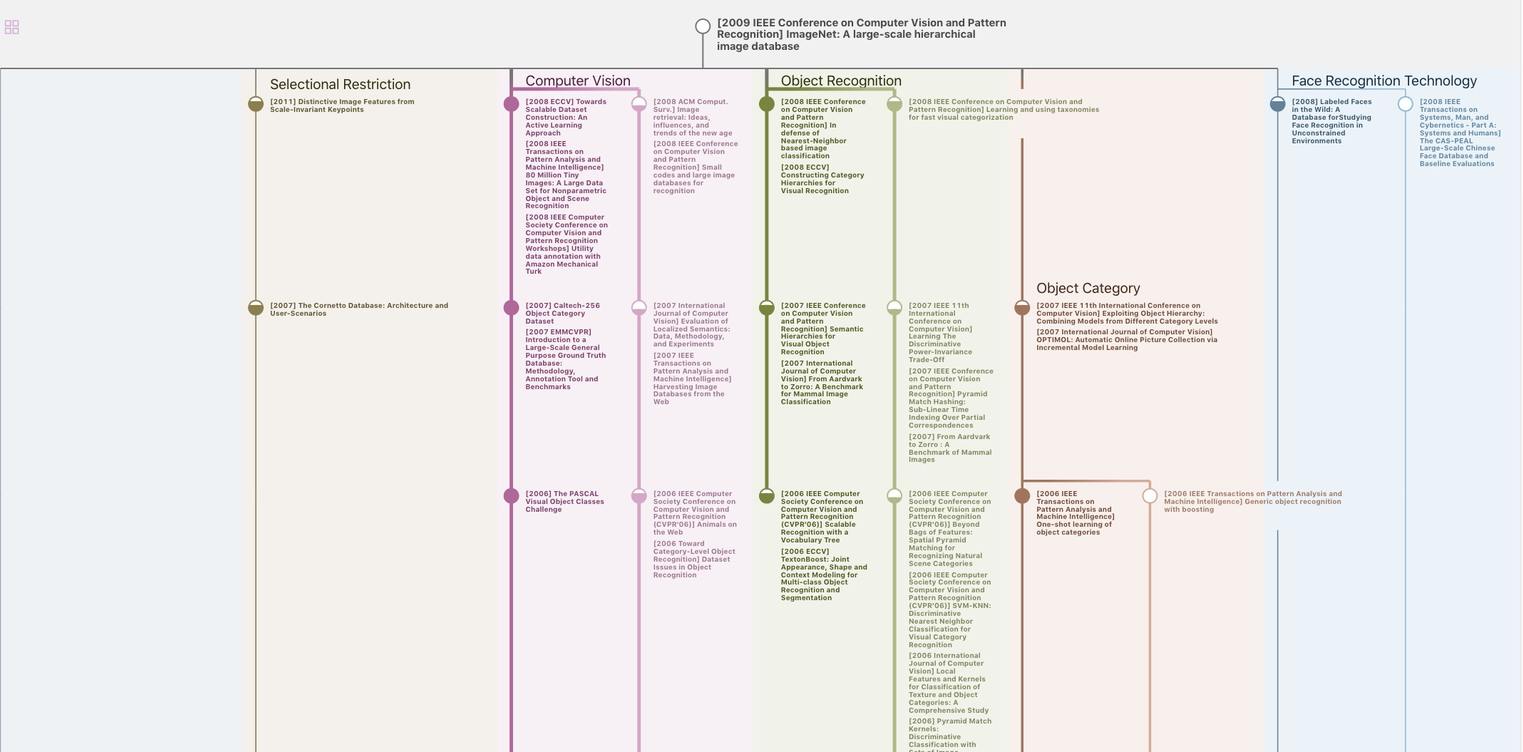
生成溯源树,研究论文发展脉络
Chat Paper
正在生成论文摘要