Learning Empirical Bregman Divergence for Uncertain Distance Representation
CoRR(2023)
摘要
Deep metric learning techniques have been used for visual representation in various supervised and unsupervised learning tasks through learning embeddings of samples with deep networks. However, classic approaches, which employ a fixed distance metric as a similarity function between two embeddings, may lead to suboptimal performance for capturing the complex data distribution. The Bregman divergence generalizes measures of various distance metrics and arises throughout many fields of deep metric learning. In this paper, we first show how deep metric learning loss can arise from the Bregman divergence. We then introduce a novel method for learning empirical Bregman divergence directly from data based on parameterizing the convex function underlying the Bregman divergence with a deep learning setting. We further experimentally show that our approach performs effectively on five popular public datasets compared to other SOTA deep metric learning methods, particularly for pattern recognition problems.
更多查看译文
关键词
Bregman divergence,distance representation,deep metric learning,visual representation
AI 理解论文
溯源树
样例
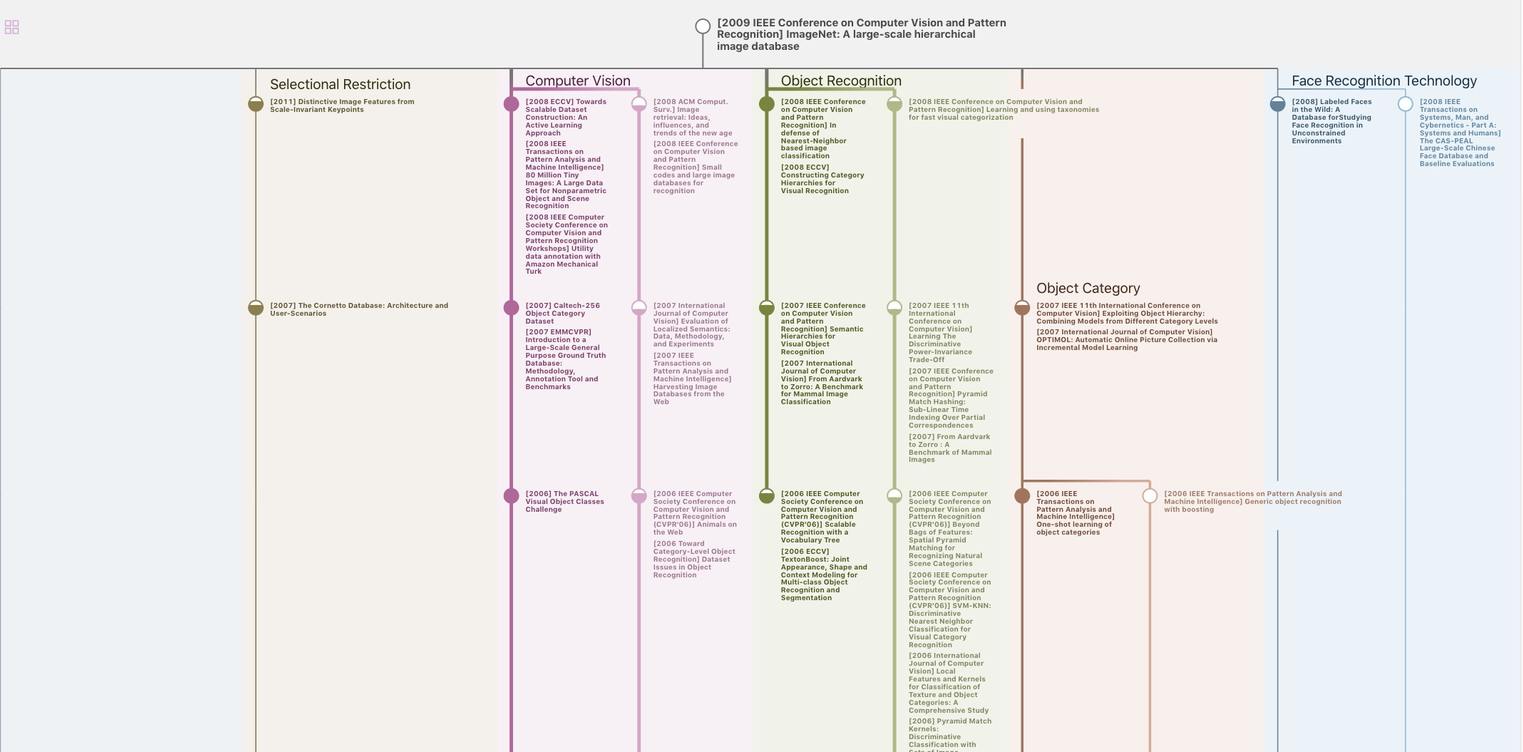
生成溯源树,研究论文发展脉络
Chat Paper
正在生成论文摘要