Continual Domain Adaptation through Pruning-aided Domain-specific Weight Modulation
CoRR(2023)
摘要
In this paper, we propose to develop a method to address unsupervised domain adaptation (UDA) in a practical setting of continual learning (CL). The goal is to update the model on continually changing domains while preserving domain-specific knowledge to prevent catastrophic forgetting of past-seen domains. To this end, we build a framework for preserving domain-specific features utilizing the inherent model capacity via pruning. We also perform effective inference using a novel batch-norm based metric to predict the final model parameters to be used accurately. Our approach achieves not only state-of-the-art performance but also prevents catastrophic forgetting of past domains significantly. Our code is made publicly available.
更多查看译文
关键词
catastrophic forgetting,CL,continual domain adaptation,continual learning,domain-specific features,domain-specific knowledge,pruning-aided domain-specific weight modulation,unsupervised domain adaptation
AI 理解论文
溯源树
样例
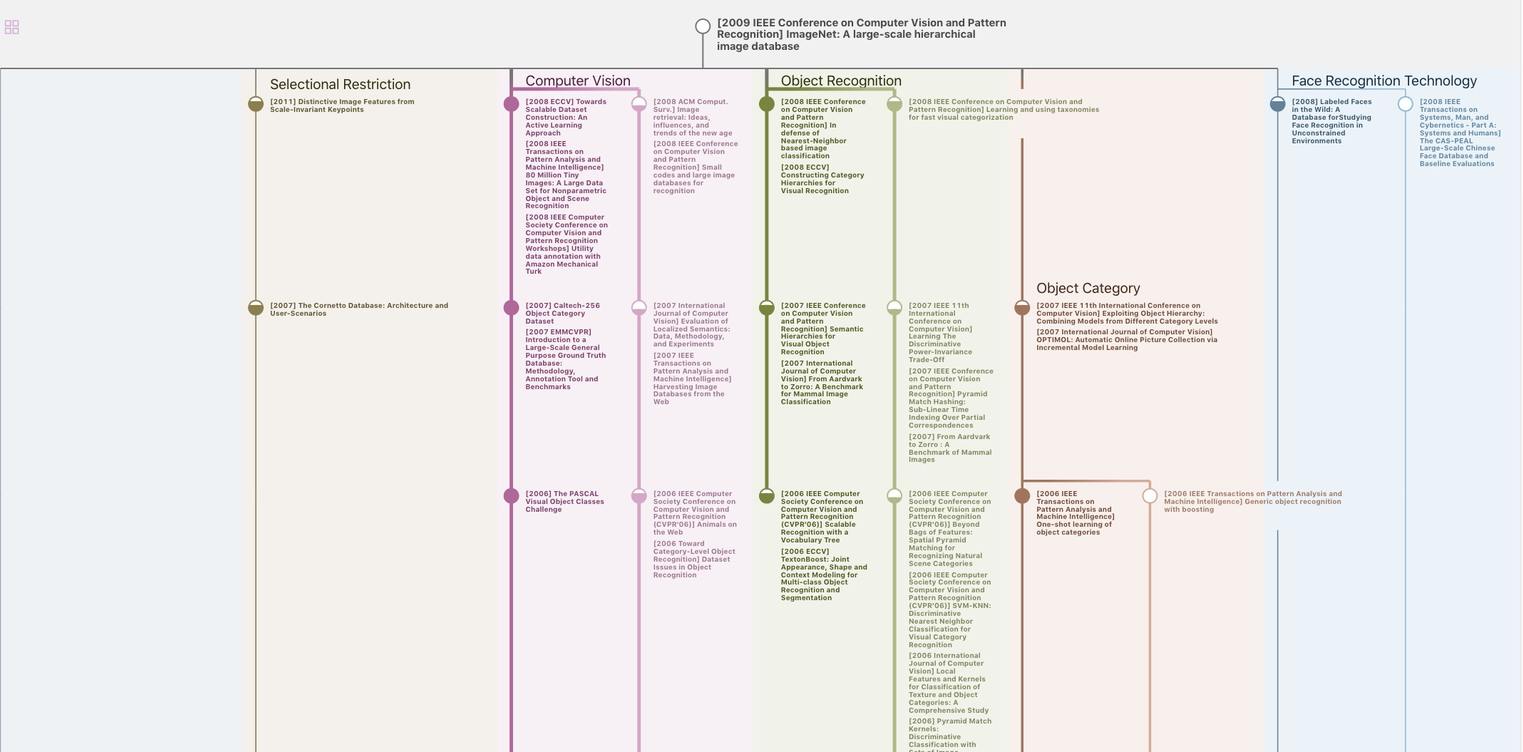
生成溯源树,研究论文发展脉络
Chat Paper
正在生成论文摘要