Text-Conditional Contextualized Avatars For Zero-Shot Personalization
CoRR(2023)
摘要
Recent large-scale text-to-image generation models have made significant improvements in the quality, realism, and diversity of the synthesized images and enable users to control the created content through language. However, the personalization aspect of these generative models is still challenging and under-explored. In this work, we propose a pipeline that enables personalization of image generation with avatars capturing a user's identity in a delightful way. Our pipeline is zero-shot, avatar texture and style agnostic, and does not require training on the avatar at all - it is scalable to millions of users who can generate a scene with their avatar. To render the avatar in a pose faithful to the given text prompt, we propose a novel text-to-3D pose diffusion model trained on a curated large-scale dataset of in-the-wild human poses improving the performance of the SOTA text-to-motion models significantly. We show, for the first time, how to leverage large-scale image datasets to learn human 3D pose parameters and overcome the limitations of motion capture datasets.
更多查看译文
关键词
text-conditional,zero-shot
AI 理解论文
溯源树
样例
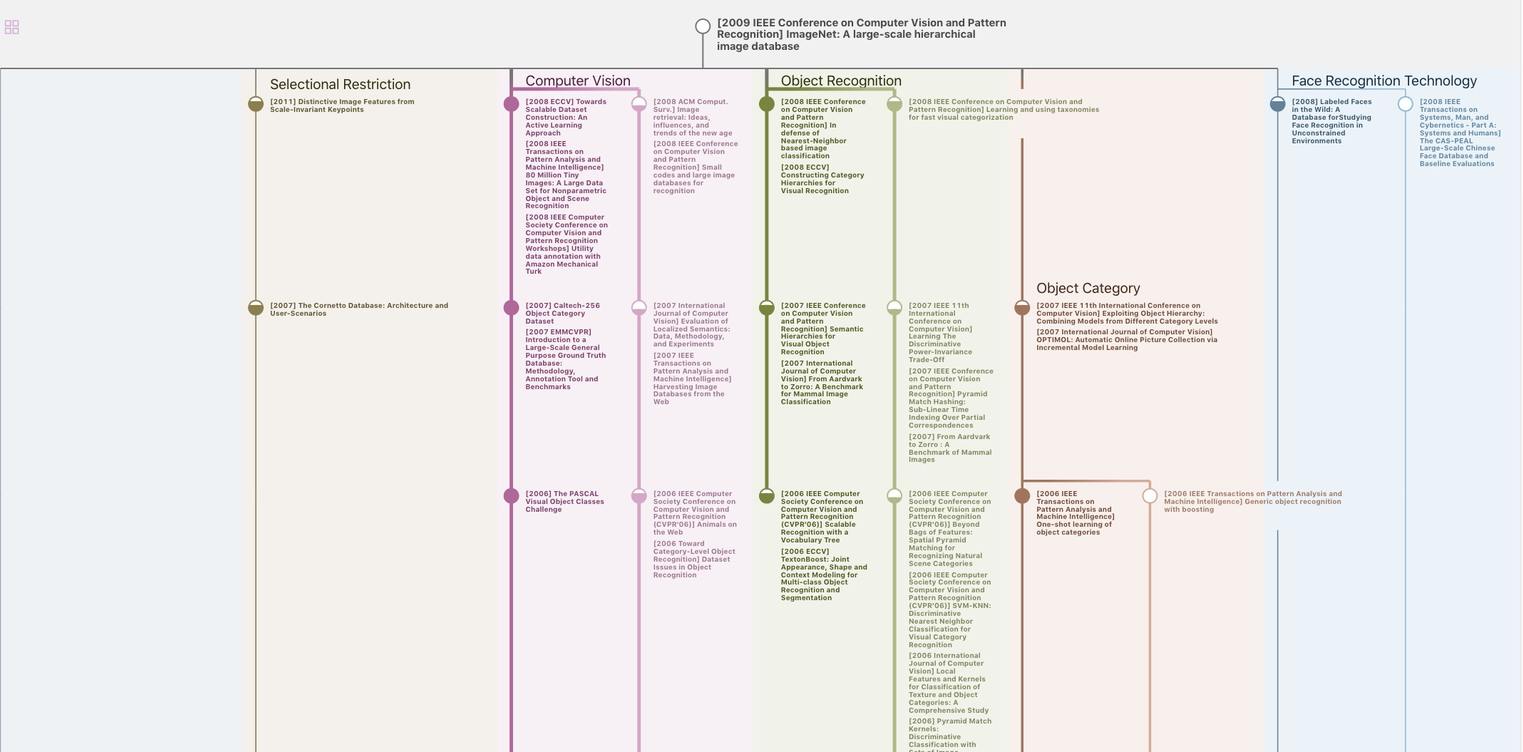
生成溯源树,研究论文发展脉络
Chat Paper
正在生成论文摘要