Multi-view representation model based on graph autoencoder
Information Sciences(2023)
摘要
Graph representation learning is a hot topic in non-Euclidean data in various domains, such as social networks, biological networks, etc. When some data labels are missing, graph autoencoder and graph variational autoencoder can perform outstanding abilities on node clustering or link prediction tasks. However, most existing graph representation learning ignores data's multi-modal features and takes the node features and graph structure features as one view. Besides, most graph autoencoders only reconstruct the node feature matrix or adjacency matrix, which does not fully use the hidden representation information. In this paper, we propose a multi-view representation model based on graph autoencoder, which can employ the global structure topology, latent local topology, and feature relative information. Meanwhile, we add another decoder to reconstruct the node feature matrix as an auxiliary task. In this way, the proposed framework can utilize the learned representation sufficiently. We validate the effectiveness of our framework on four datasets, and the experimental results demonstrate superior performance compared with other advanced frameworks.
更多查看译文
关键词
Graph representation learning,Graph autoencoder,Unsupervised learning,Multi-view learning
AI 理解论文
溯源树
样例
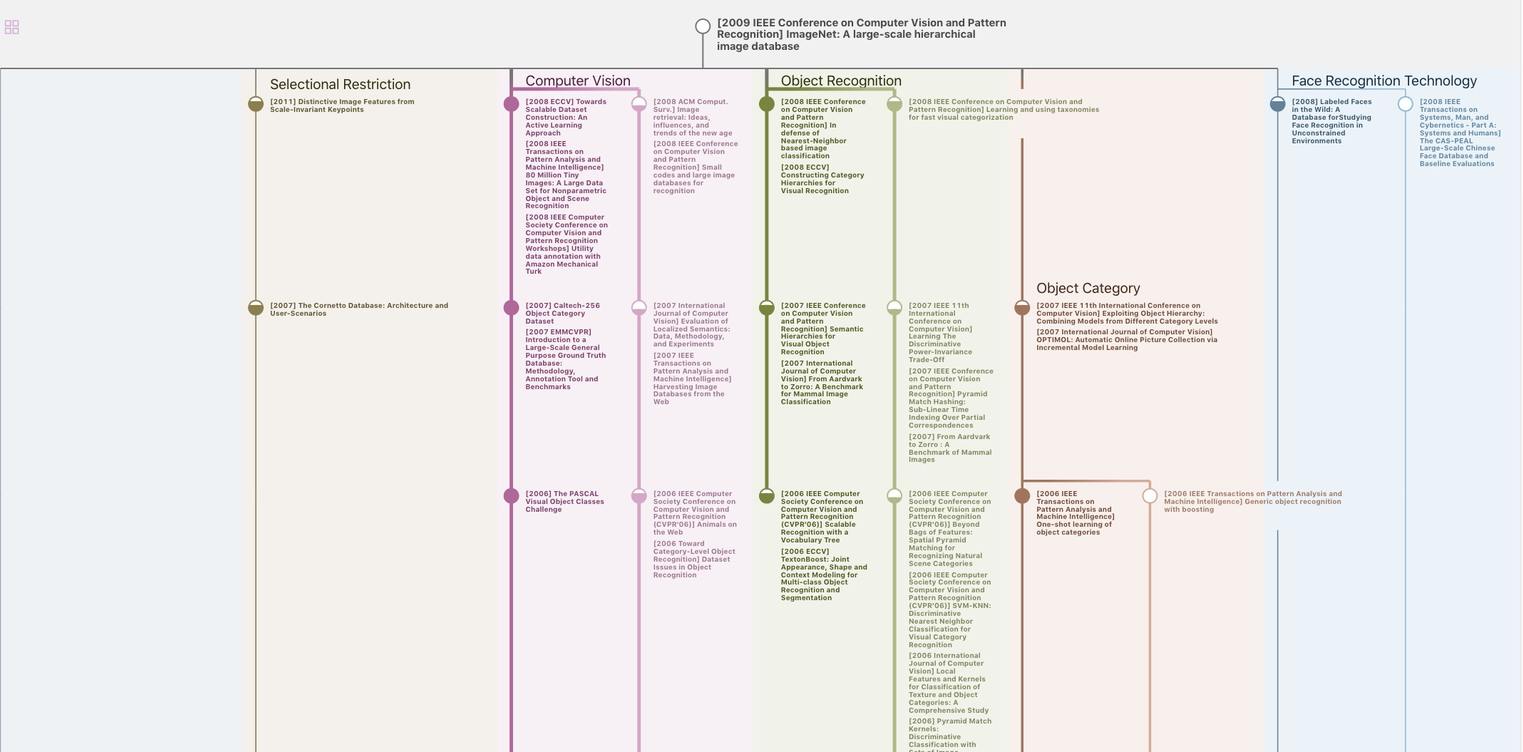
生成溯源树,研究论文发展脉络
Chat Paper
正在生成论文摘要