The role of hyper-parameters in relational topic models: Prediction capabilities vs topic quality
Information Sciences(2023)
摘要
In this paper, we investigate the impact of optimal hyper-parameter configuration in relational topic models. The main goal is to validate the hypothesis that single-objective Bayesian Optimization (BO) can discover a hyper-parameter setting that leads a set of relational topic models to simultaneously ensure good prediction capabilities and significant topics from a qualitative perspective. Our research, as a result of a comparative analysis performed on 7 state-of-the-art models, 5 performance measures and 3 datasets, has highlighted three main findings: (1) the majority of relational topic models are not able to offer a good trade-off between classification capabilities and topic interpretability; (2) single-objective optimization of hyper-parameters, targeted on maximizing the F1-Measure, is able to create topics that are also optimal with respect to the Kullback Leibler divergence measure; (3) the Pareto frontiers across several performance metrics reveals that the most promising trade-off between the performance metrics can be obtained by Constrained Relational Topic Models.
更多查看译文
关键词
Topic modelling,Hyper-parameter tuning,Evaluation
AI 理解论文
溯源树
样例
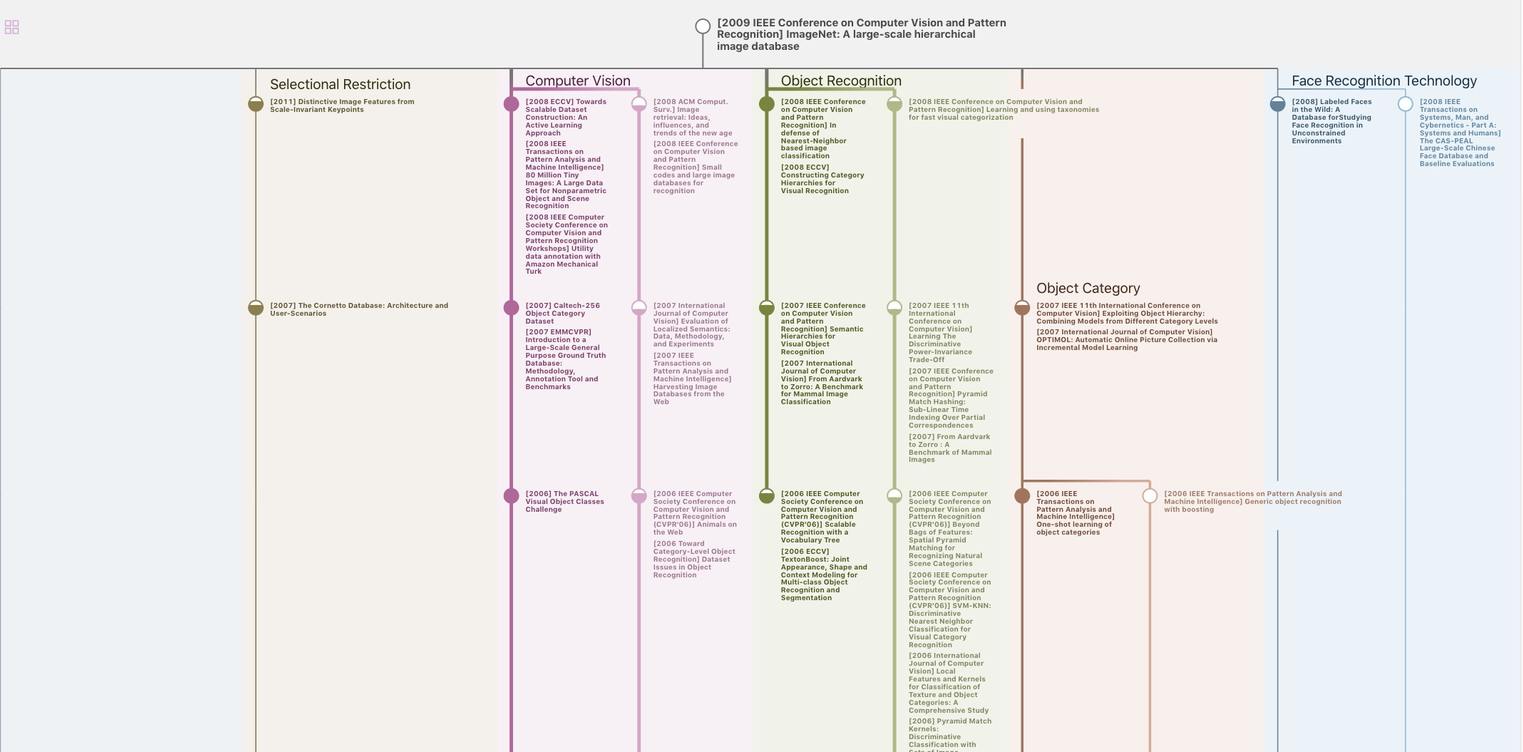
生成溯源树,研究论文发展脉络
Chat Paper
正在生成论文摘要