Fog-DeepStream: A new approach combining LSTM and Concept Drift for data stream analytics on Fog computing
Internet of Things(2023)
摘要
Applications and infrastructures designed to support Fog Computing in IoT (Internet of Things) environments generate large volumes of data, usually characterized as open-ended streams, thus making unfeasible the adoption of traditional modeling approaches. Namely, the main limitations with such approaches are: (i) the adoption of offline models might be out of date when new data are available; (ii) the need for high computational power to store and process all data from IoT devices; and (iii) the transmission of all monitored data might overload the network connections from devices to servers. Such limitations are accentuated in complex IoT environments that combine different devices and servers configured in Fog and Cloud Computing. Aiming at dealing with such limitations, we propose a challenging approach, referred to as Fog-DeepStream, which combines three important research areas: Signal Processing, Concept Drift, and Deep Neural Networks. Initially, our approach uses Wavelet Transform to undersample data streams without losing details about their main behavior. Next, we use Concept Drift algorithms to only transmit the data in the environment when a new behavior is detected. These two steps reduce the amount of processed and exchanged data that Deep Neural Networks will later model. Our results emphasize that the contributions of Fog-DeepStream are twofold: we provide an incremental approach to efficiently model data streams in Fog Computing and reduce data transmission between computational resources, thus making it possible to save energy in IoT scenarios.
更多查看译文
关键词
Internet of Things,Data stream,Fog computing,Concept Drift,Signal processing,Deep neural networks,Big data streams
AI 理解论文
溯源树
样例
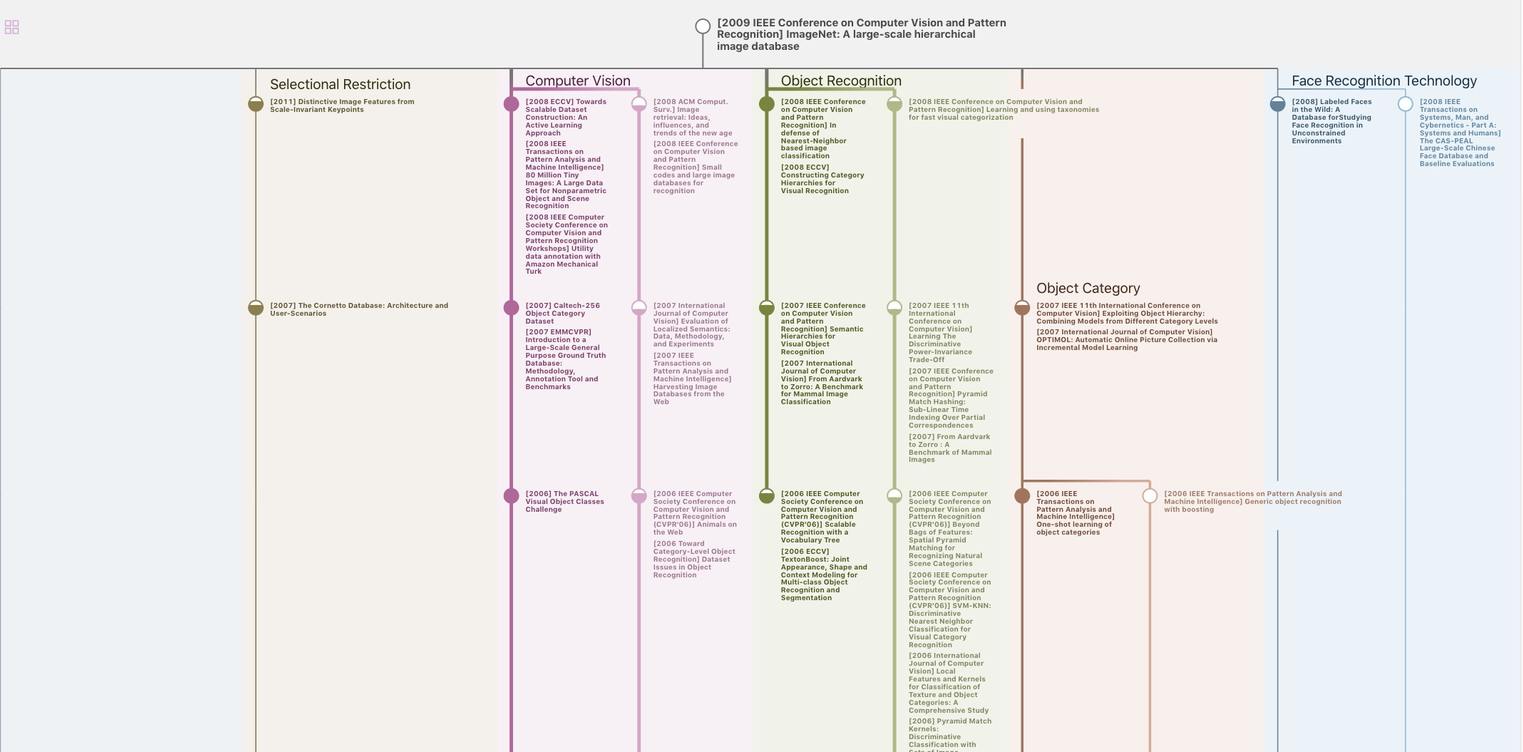
生成溯源树,研究论文发展脉络
Chat Paper
正在生成论文摘要