A Dehazing Method for Remote Sensing Image Under Nonuniform Hazy Weather Based on Deep Learning Network
IEEE Transactions on Geoscience and Remote Sensing(2023)
摘要
Different from the ground image with uniform haze, the haze in remote sensing (RS) image has the characteristics of irregular shape and uneven concentration in hazy weather. It brings a great challenge to the application of RS image data in advanced image processing tasks. A novel dehazing network for nonuniform hazy RS images, named KFA-Net, is proposed to solve the aforementioned issues. The designed asymmetric size feature cascade (ASFC), k-means pixel attention (KPA), and FFT channel attention (FCA) in KFA-Net all show excellent effects. Compared with symmetrically linked typical Unet, ASFC can more easily extract shallow features for feature reconstruction. Furthermore, different from the commonly used pixel attention that compresses feature maps directly, KPA introduces the k-means clustering algorithm in machine learning into the attention mechanism, which facilitates network training to focus on the thick hazy region. Compared to a typical squeeze-and-excitation block, FCA uses the low-frequency region feature of the spectrogram to obtain the attention weight coefficient in the frequency domain, making network training pay more attention to the feature of the image low-frequency region. Extensive comparison experiments verify that the proposed KFA-Net has great superiority. Peak signal to noise ratio (PSNR) and structural similarity (SSIM) of KFA-Net are 31.0952% and 6.6401% higher than DCP with the highest citation in traditional dehazing methods, respectively. PSNR and SSIM of KFA-Net are 2.2049% and 0.4966% higher than the recently proposed 4KDehazing with the best performance among all comparison dehazing methods, respectively. The KFA-Net proposed in this research can greatly enhance the temporal and spatial scopes of RS image application in hazy weather conditions.
更多查看译文
关键词
Convolutional neural networks,deep learning (DL),feature extraction,image restoration
AI 理解论文
溯源树
样例
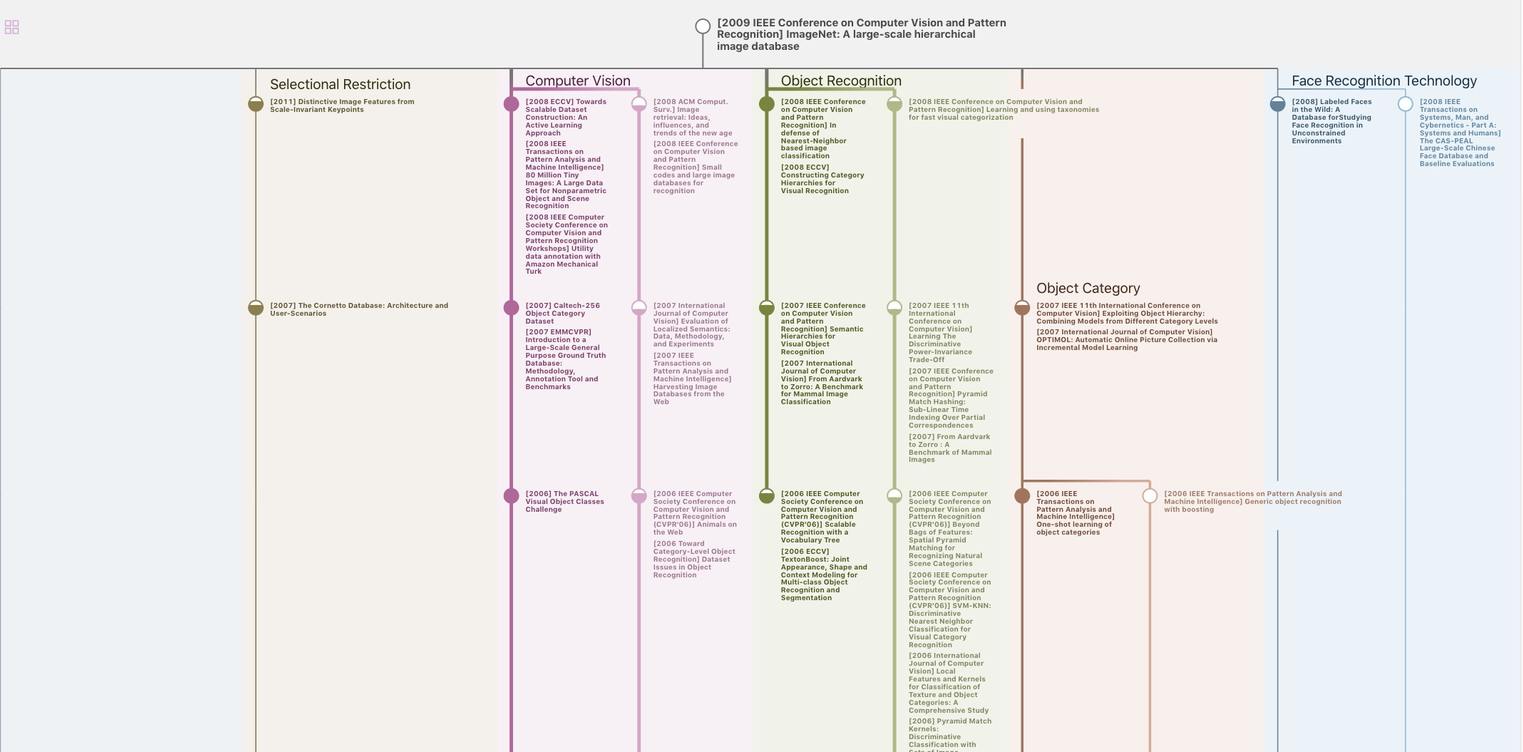
生成溯源树,研究论文发展脉络
Chat Paper
正在生成论文摘要