Prediction of PM2.S concentration in Changchun based on ensemble learning model
2022 18th International Conference on Computational Intelligence and Security (CIS)(2022)
摘要
With the continuous development of society, the air quality condition is declining day by day. This study focuses on the concentration of PM2.5. Taking Changchun City as the research object, using the data from 2014 to 2020 and decision tree, random forest, Adaboost, GBDT, KNN, XGBoost, LightgBM, CatBoost, SVR, Stacking, Blending, and multiple linear regression model were established respectively. The experimental results show that the concentration of PM2.5 in Changchun is high in spring and winter, while low in summer and autumn. PM2.5 concentration has a strong correlation with air quality data, but a weak correlation with meteorological data. The prediction model based on ensemble learning has a significant prediction effect on PM2.5 concentration, and the goodness of fit of all ensemble learning models on the test set reaches more than 0.92. The best prediction effect is based on the random forest model, LightGBM model and gradient lifting model in the first layer, and the Stacking model based on KNN algorithm in the second layer, The goodness of fit on the test set is above 0.94. From the aspect of feature importance, PMI0, CO, N02, SO2 and other air quality factors have a great influence on the PM2.5 prediction results in Changchun.
更多查看译文
关键词
PM2.5,Ensemble learning,Relevance,Forecast,Feature importance
AI 理解论文
溯源树
样例
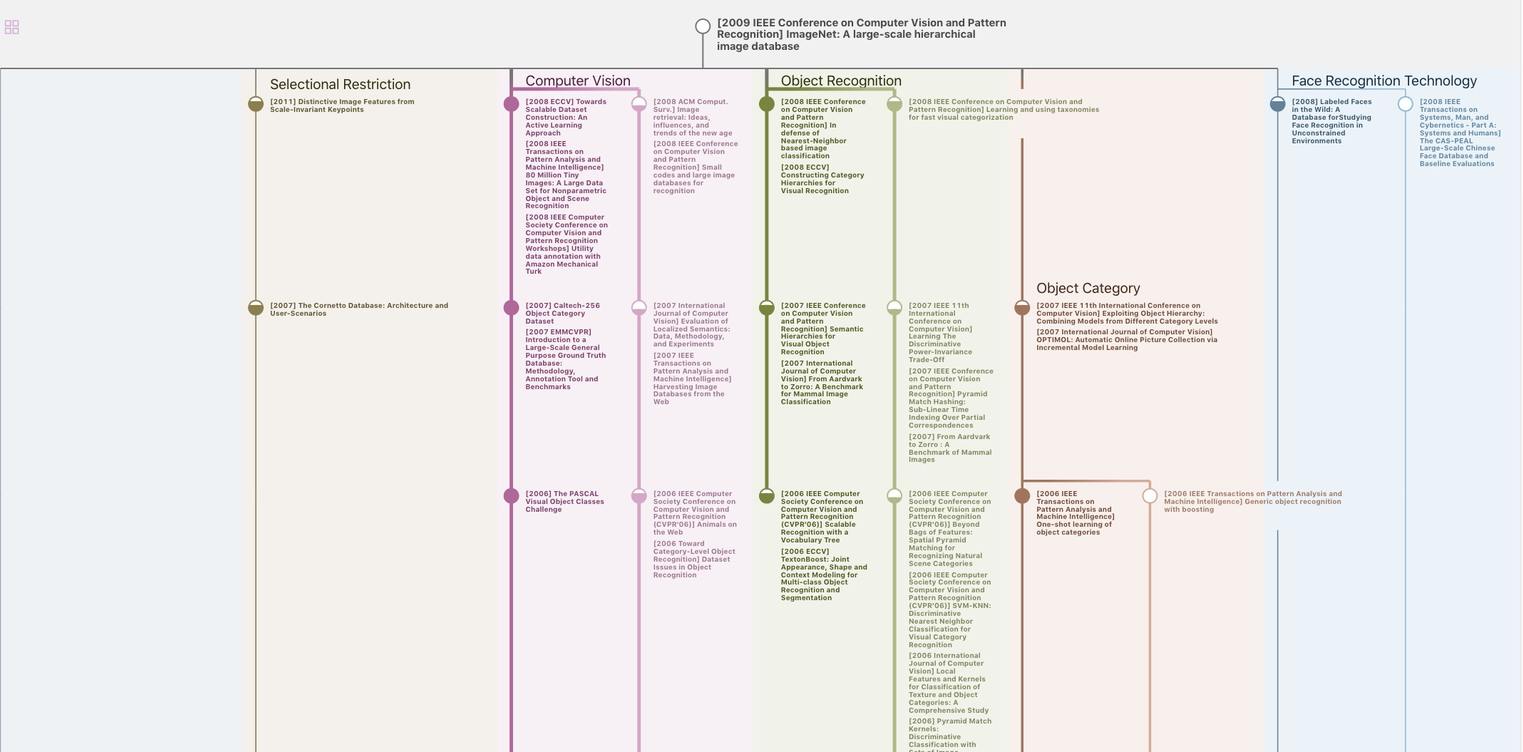
生成溯源树,研究论文发展脉络
Chat Paper
正在生成论文摘要