Multi-Kernel Correntropy Regression: Robustness, Optimality, and Application on Magnetometer Calibration
IEEE TRANSACTIONS ON AUTOMATION SCIENCE AND ENGINEERING(2023)
摘要
This paper investigates the robustness and optimality of the multi-kernel correntropy (MKC) on linear regression. We first derive an upper error bound for a scalar regression problem in the presence of arbitrarily large outliers. Then, we find that the proposed MKC is related to a specific heavy-tail distribution, where its head shape is consistent with the Gaussian distribution while its tail shape is heavy-tailed and the extent of heavy-tail is controlled by the kernel bandwidth. Interestingly, when the bandwidth is infinite, the MKC-induced distribution becomes a Gaussian distribution, enabling the MKC to address both Gaussian and non-Gaussian problems by appropriately selecting correntropy parameters. To automatically tune these parameters, an expectation-maximization-like (EM) algorithm is developed to estimate the parameter vectors and the correntropy parameters in an alternating manner. The results show that our algorithm can achieve equivalent performance compared with the traditional linear regression under Gaussian noise, and significantly outperforms the conventional method under heavy-tailed noise. Both numerical simulations and experiments on a magnetometer calibration application verify the effectiveness of the proposed method. Note to Practitioners-The goal of this paper is to enhance the accuracy of conventional linear regression in handling outliers while maintaining its optimality under Gaussian situations. Our algorithm is formulated under the maximum likelihood estimation (MLE) framework, assuming the regression residuals follow a type of heavy-tailed noise distribution with an extreme case of Gaussian. The degree of the heavy tail is explored alternatingly using an Expectation-Maximization (EM) algorithm which converges very quickly. The robustness and optimality of the proposed approach are investigated and compared with the traditional approaches. Both theoretical analysis and experiments on magnetometer calibration demonstrate the superiority of the proposed method over the conventional methods. In the future, we will extend the proposed method to more general cases (such as nonlinear regression and classification) and derive new algorithms to accommodate more complex applications (such as with equality or inequality constraints or with prior knowledge of parameter vectors).
更多查看译文
关键词
Linear regression,multi-kernel correntropy,robustness and optimality,maximum likelihood estimation,expectation-maximization,magnetometer calibration
AI 理解论文
溯源树
样例
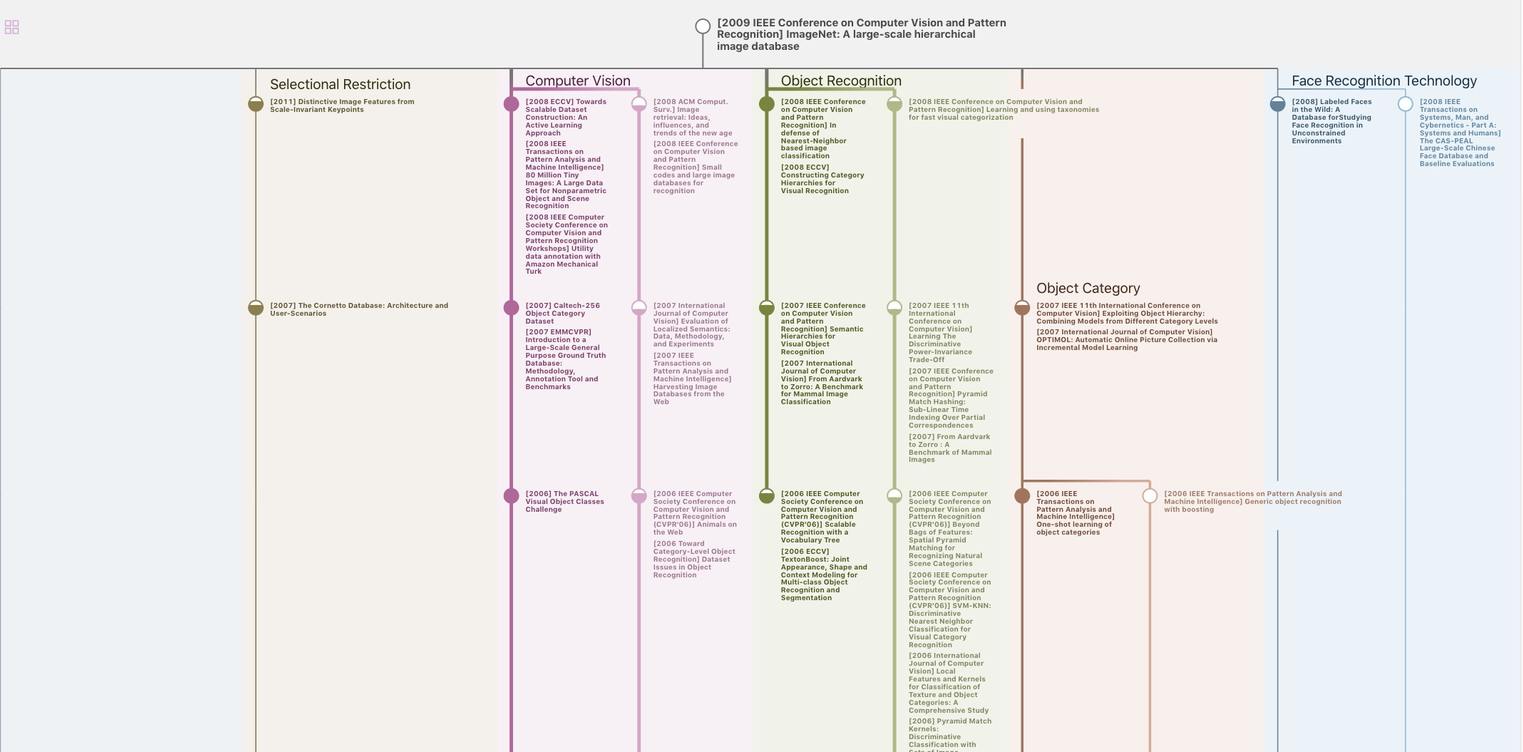
生成溯源树,研究论文发展脉络
Chat Paper
正在生成论文摘要