Multikernel Correntropy-Based Orientation Estimation of IMUs: Gradient Descent Methods
IEEE TRANSACTIONS ON INSTRUMENTATION AND MEASUREMENT(2024)
摘要
This article presents two computationally efficient algorithms for the orientation estimation of inertial measurement units (IMUs): the multikernel correntropy-based gradient descent (CGD) and the multikernel correntropy-based decoupled orientation estimation (CDOE). Traditional methods, such as gradient descent (GD) and decoupled orientation estimation (DOE), rely on the least square (LS) criterion in algorithm derivation, making them vulnerable to external acceleration and magnetic interference. To address this issue, we first demonstrate that the multikernel correntropy loss (MKCL) is an optimal objective function under the maximum likelihood estimation (MLE) framework when the noise follows a specific type of heavy-tailed distribution. Then, we provide some important properties of the MKCL as a cost function. By replacing the LS cost with the MKCL, we develop the CGD and CDOE algorithms. We evaluate the effectiveness of the proposed methods by comparing them with existing algorithms in various situations. The experimental results indicate that our proposed approaches (CGD and CDOE) outperform their conventional counterparts (GD and DOE), especially when faced with external acceleration and magnetic disturbances. Meanwhile, the new algorithms exhibit significantly lower computational complexity than Kalman filter (KF)-based approaches, making them suitable for applications with low-cost microprocessors.
更多查看译文
关键词
Gradient descent (GD),heavy-tailed noise,inertial measurement units (IMUs),maximum likelihood estimation (MLE),multikernel correntropy (MKC),orientation estimation
AI 理解论文
溯源树
样例
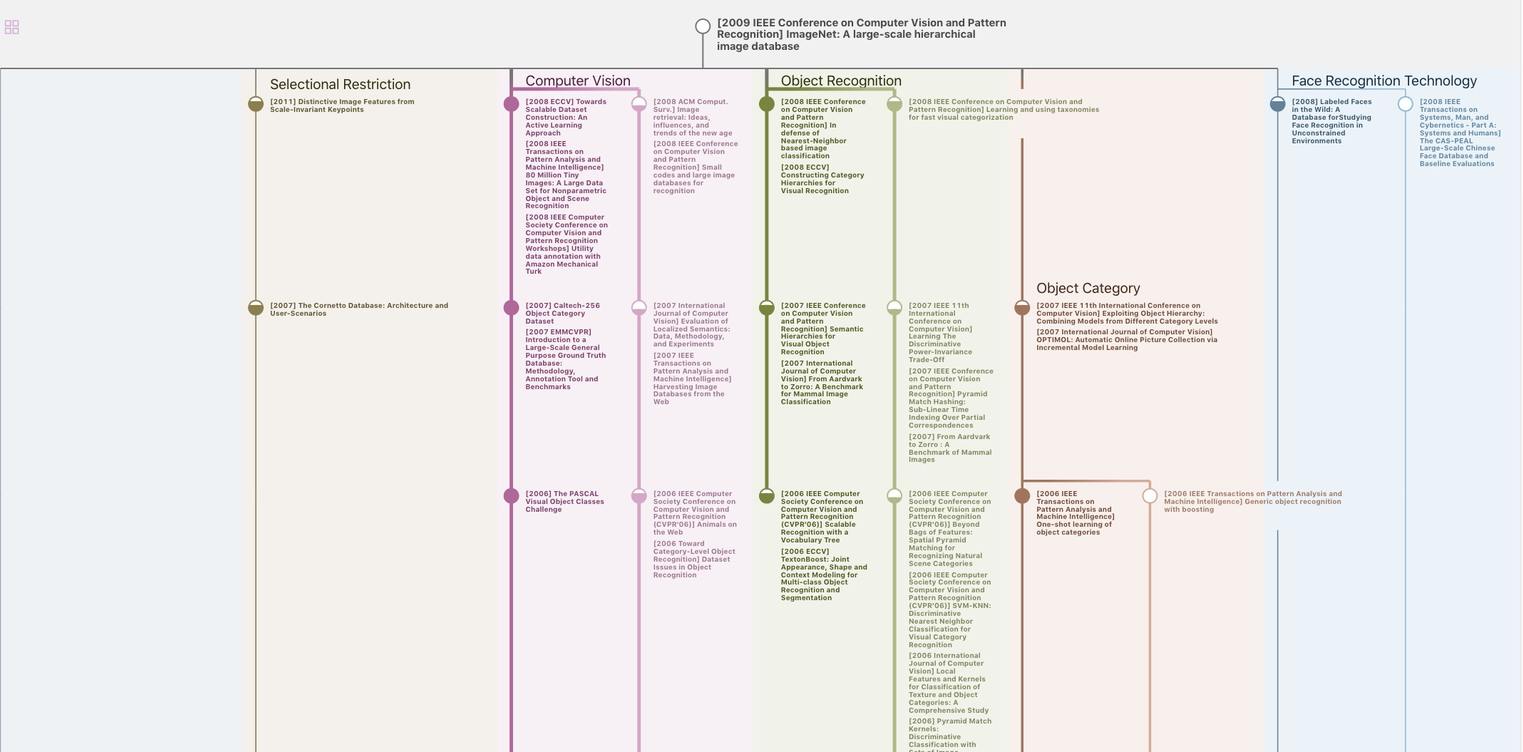
生成溯源树,研究论文发展脉络
Chat Paper
正在生成论文摘要