Deep Learning Aided Multi-Level Transmit Power Recognition in Cognitive Radio Networks
IEEE Transactions on Cognitive Communications and Networking(2023)
摘要
According to the regulations of the hybrid access strategy in cognitive radio network, the secondary user (SU) needs to identify the primary user’s (PU) specific transmit power level to avoid unacceptable interference with the PU. However, the conventional transmit power recognition methods cannot accurately identify the transmit power in conditions with low signal-to-noise ratio, fading channels and the existence of noise uncertainty, since those methods are based on a fixed statistical theory to model the dynamic electromagnetic environment mathematically. To address these issues, a ResNet-based multi-level transmission power recognition (MTPR) architecture is presented in this paper. Furthermore, the proposed architecture is implemented in two cases with different observation data. In the first case, the received signal’s covariance matrix (CM) containing rich energy information is used as the observation data of CM-MTPR scheme. To further improve the identification accuracy, in the second case, the in-phase and quadrature-phase (IQ) data sampled from the received signal that preserves more original information is configured as the observation data of IQ-MTPR scheme. The IQ-MTPR scheme, however, consumes additional computing resources which forms a trade-off between identification performance and computational consumption with the CM-MTPR scheme. Simulation results demonstrate the identification performance of the proposed schemes.
更多查看译文
关键词
Cognitive radio,convolutional neural network,multiple transmit power levels recognition
AI 理解论文
溯源树
样例
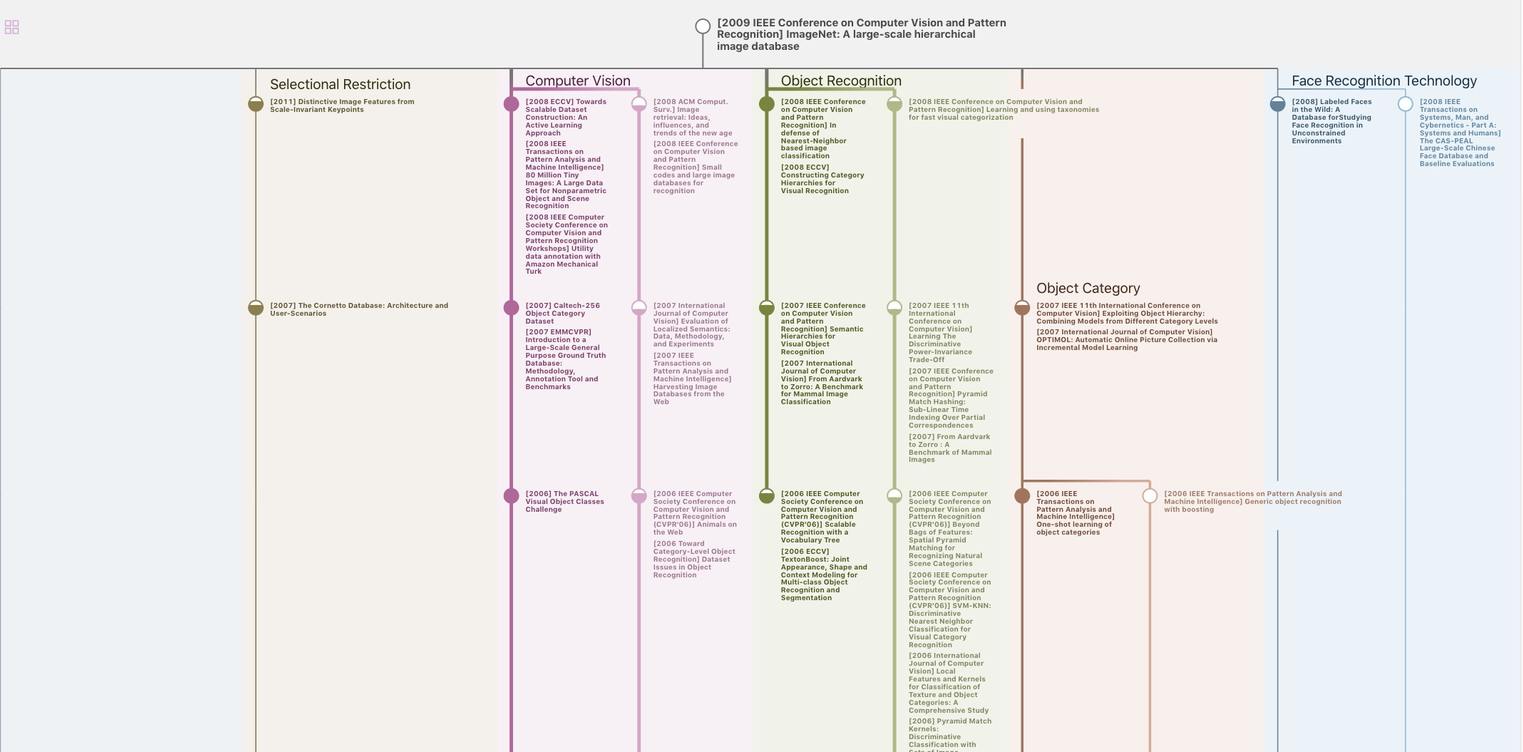
生成溯源树,研究论文发展脉络
Chat Paper
正在生成论文摘要