Methods for Detecting Epilepsy: A Comparative Analysis on Improved Deep Learning Algorithms
2022 12th International Conference on Electrical and Computer Engineering (ICECE)(2022)
摘要
Conditions affecting the brain, such as epilepsy, can be diagnosed with the help of electroencephalograms (EEGs). To analyze EEG signals, Deep Learning Algorithms have been proved very effective and efficient tools to detect epileptic seizures. In this work, we proposed a unique Recurrent Neural Network (RNN) architecture to differentiate epileptic people from the healthy counterpart. RNN is the improved version of previous ChronoNet and a ConvNet, and it consists of six convolution-max-pool blocks, followed by three standard convolution-average-pool blocks and one convolution-global_average-pool and a dense softmax classification layer. The deep Gated Recurrent Unit (GRU) layers are piled on top of each other and tightly coupled in a feed-forward fashion in proposed ChronoNet, wherein each ID convolution layer uses multiple filters of progressively increasing lengths. The proposed ChronoNet architecture utilizes six ID convolution layer of different filter size while ConvNet Model implements six ID convolution layer of the same filter size. Using the same framework, Keras, the proposed improved ChronoNet and Deep CNN (ConvNet) architectures have been implemented using the data found at Temple University Hospital EEG Corpus and it was found that the improved ChronoNet outperforms the ConvNet yielding accuracies of around 97.20% and 88.14% respectively.
更多查看译文
关键词
EEG,ChronoNet,ConvNet,epilepsy,Keras,filter size
AI 理解论文
溯源树
样例
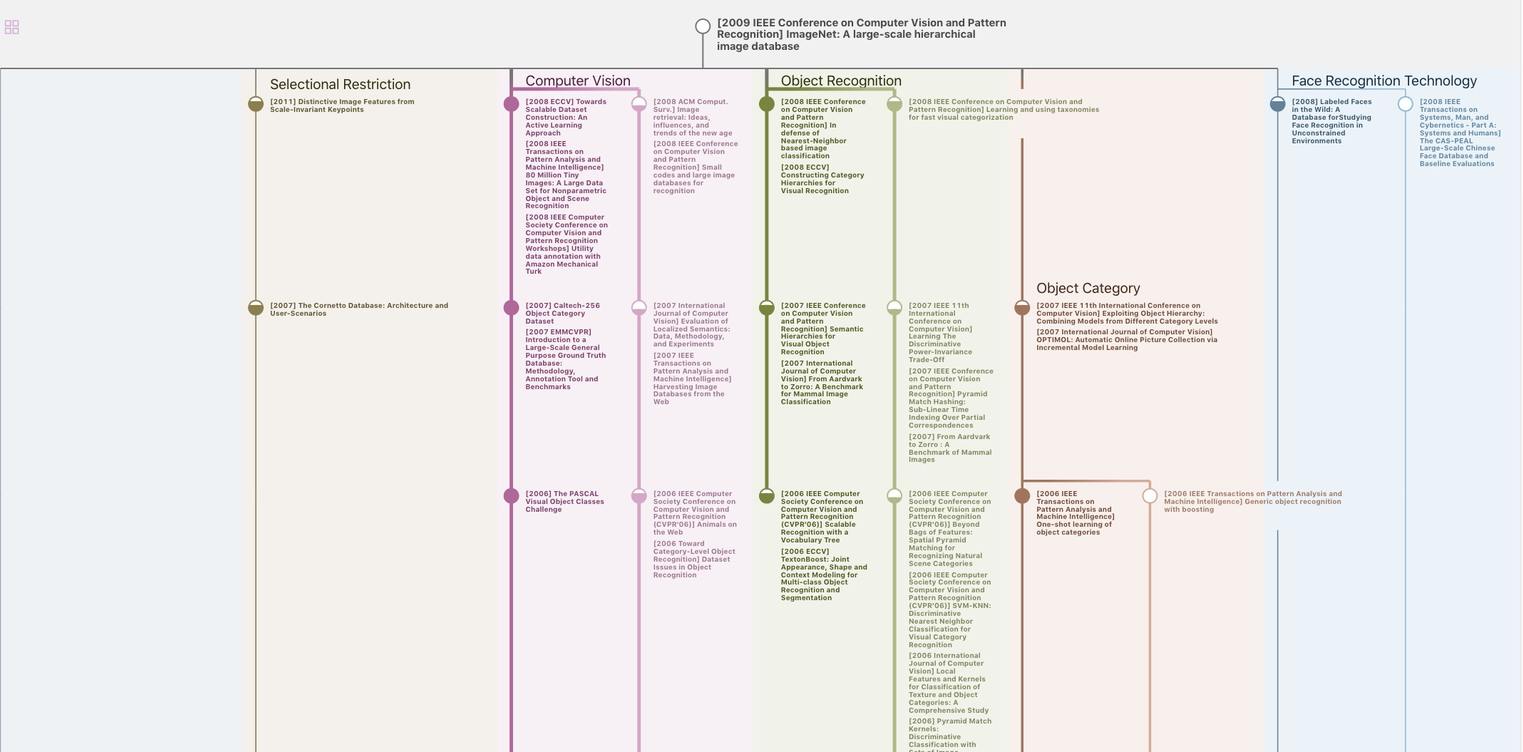
生成溯源树,研究论文发展脉络
Chat Paper
正在生成论文摘要