A Comparison of Regression Techniques for Prediction of Air Quality in Smart Cities
2023 1st International Conference on Advanced Innovations in Smart Cities (ICAISC)(2023)
摘要
The expansion of the internet of things (IoT) and Big Data are two factors that have contributed to the rise in popularity of smart cities. The ability to anticipate the quality of the air in an area with precision and efficiency is one of the fundamental building blocks of a smart city. The amount of polluted air found in smart cities throughout the world has been gradually growing. Because of this, there has been a rise in the concentration of several air pollutants in the environment, including particulate matter (PM 10), sulphur dioxide (SO2), and PM 2.5, amongst others. Because of the possibility of uncontrollable repercussions, such as an increase in the severity of asthma and cardiovascular disease, this situation poses a risk to the country and to the people who live in it. Heavy industry and vehicle exhaust have been major contributors to the growth of air pollution in smart cities such as New Delhi, Bombay, Chandigarh, and Bengaluru in India. The purpose of this investigation is to compare and contrast the efficiency of a variety of machine learning methods in order to assess the precision of the air quality index (AQI) projection of PM 2.5 in Chandigarh, India. Models for predicting AQI are trained and tested using a variety of statistical techniques like Linear regression, Lasso regression, KNN regression, and Random Forest regression This Root Mean Square Error (RMSE) found for Linear regression, Lasso regression, KNN regression, and Random Forest regression are 31.01, 29,45, 37.09 and 28.3. From all four models, random forest regression was more accurate than the other three regression models in estimating PM 2.5 levels in India’s smart city.
更多查看译文
关键词
Particulate Matter (PM2.5),Air Quality Index,Regression,Smart City,Random Forest
AI 理解论文
溯源树
样例
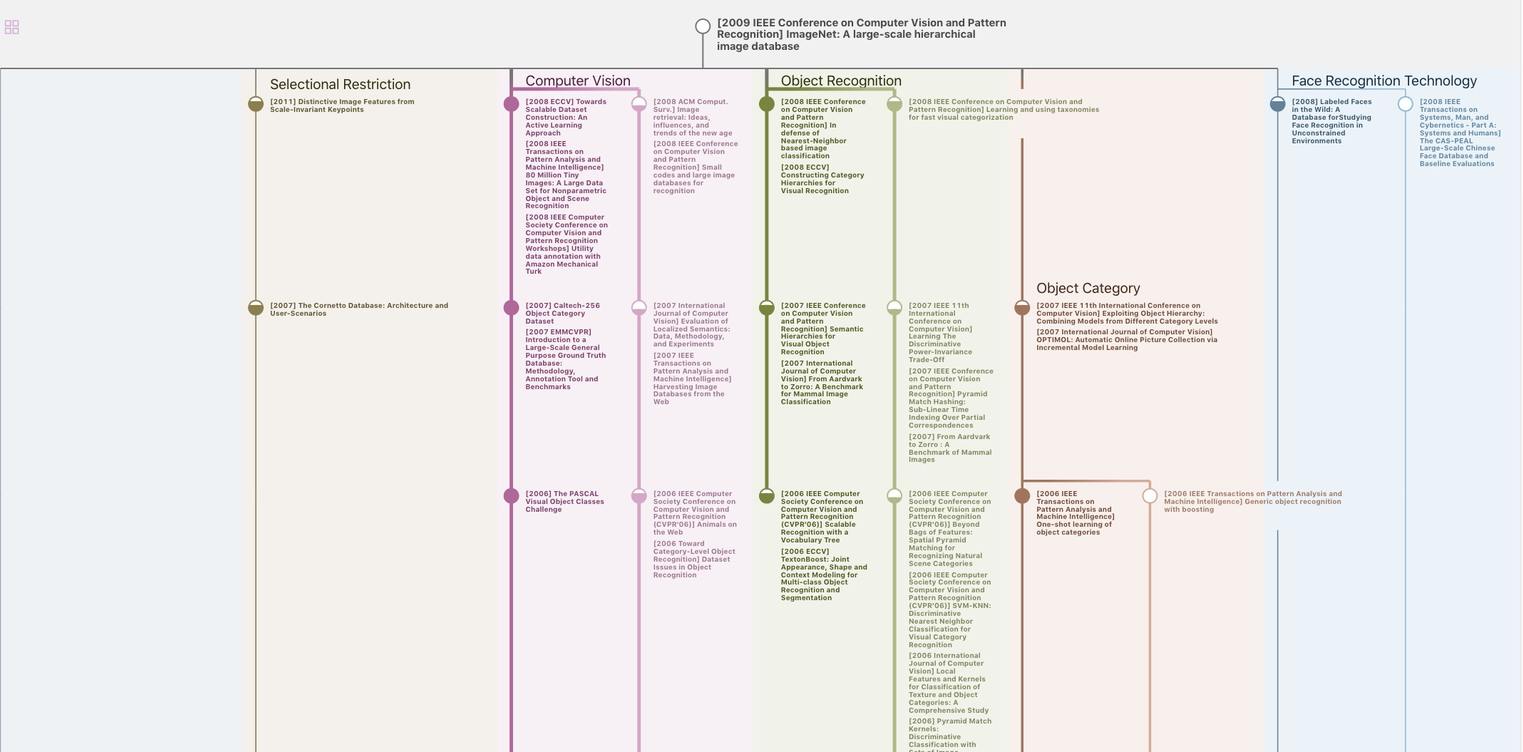
生成溯源树,研究论文发展脉络
Chat Paper
正在生成论文摘要