Structured Sparsity Optimization With Non-Convex Surrogates of $\ell _{2,0}$ ℓ 2 , 0 -Norm: A Unified Algorithmic Framework
IEEE Transactions on Pattern Analysis and Machine Intelligence(2023)
摘要
In this article, we present a general optimization framework that leverages structured sparsity to achieve superior recovery results. The traditional method for solving the structured sparse objectives based on
$\ell _{2,0}$
-norm is to use the
$\ell _{2,1}$
-norm as a convex surrogate. However, such an approximation often yields a large performance gap. To tackle this issue, we first provide a framework that allows for a wide range of surrogate functions (including non-convex surrogates), which exhibits better performance in harnessing structured sparsity. Moreover, we develop a fixed point algorithm that solves a key underlying non-convex structured sparse recovery optimization problem to global optimality with a guaranteed super-linear convergence rate. Building on this, we consider three specific applications, i.e., outlier pursuit, supervised feature selection, and structured dictionary learning, which can benefit from the proposed structured sparsity optimization framework. In each application, how the optimization problem can be formulated and thus be relaxed under a generic surrogate function is explained in detail. We conduct extensive experiments on both synthetic and real-world data and demonstrate the effectiveness and efficiency of the proposed framework.
更多查看译文
关键词
Structured sparsity,non-convex surrogate,fixed-point algorithm
AI 理解论文
溯源树
样例
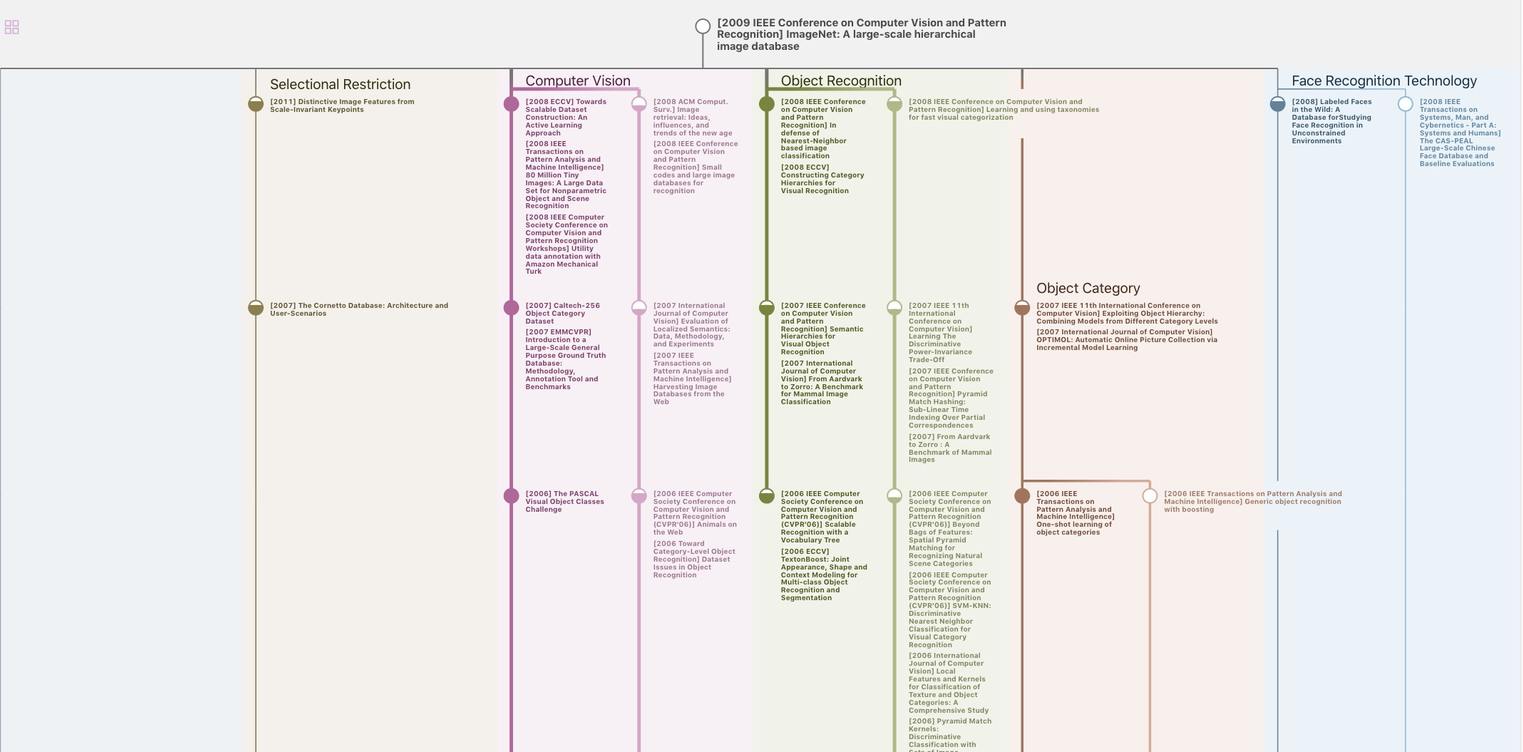
生成溯源树,研究论文发展脉络
Chat Paper
正在生成论文摘要