Medical Datasets Classification using a Hybrid Genetic Algorithm for Feature Selection based on Pearson Correlation Coefficient
2022 International Conference on Machine Learning, Computer Systems and Security (MLCSS)(2022)
摘要
The method of selecting useful features to incorporate into the creation of a predictive model is known as feature selection. In order to select the best features from datasets, this article presents a hybrid feature selection approach that combines genetic algorithm with Pearson correlation coefficient. A classifier uses the extracted features as input to determine whether or not a person has a disease. The proposed technique for diagnosing heart disease and diabetes, which have greater rates of influence on lowering quality of life globally, is developed. Heart disease, diabetes, and hepatitis are some of the selected datasets that were retrieved from the UCI repository and evaluated using the proposed methods. The experiments are carried out to analyze the performance of the genetic algorithm using the k-nearest neighbor classifier and the Pearson correlation coefficient. Ten-fold cross validation is used to attain the classification accuracy. For the hepatitis, diabetes, and heart disease datasets, the accuracy of the proposed methods is 96.87%, 89.53%, and 97.03% respectively. The outcomes indicated our proposed algorithm's higher accuracy in comparison to other available techniques in the field of pattern recognition and classification.
更多查看译文
关键词
k-nearest neighbor (kNN),Pearson Correlation coefficient(PCC),Genetic Algorithm (GA)
AI 理解论文
溯源树
样例
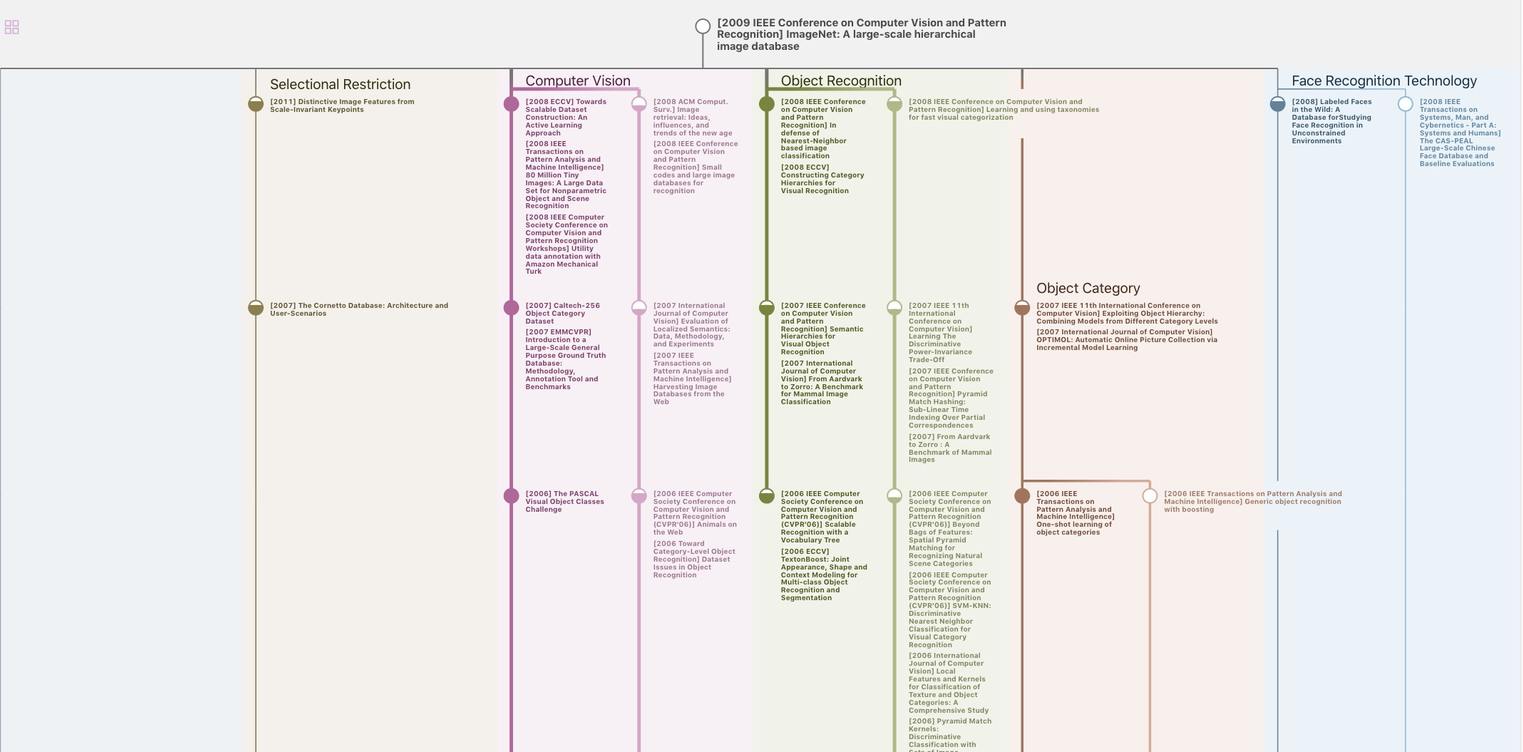
生成溯源树,研究论文发展脉络
Chat Paper
正在生成论文摘要