Dirichlet Dempster-Shafer Fusion System for Multidimensional Data Classification
2022 First International Conference on Computer Communications and Intelligent Systems (I3CIS)(2022)
摘要
In this paper, we tackle the problem of multidimensional (multi-attribute) data classification based on belief functions theory. The interest of such approaches relies on considering a more general reasoning frame, incorporating compound hypotheses in addition to conventional exclusive ones that can be perceived as classes. The proposed approach falls under this category and hinges on a general scheme: Module and sub-module extraction, evidential modeling of information, fusion of such information, and decision making. Our main contribution consists in adopting a novel evidential modeling of intra-attribute information using the Dirichlet mixture process for density estimation, in order to model information related to both simple and compound hypotheses. Our approach has then been assessed on some datasets from UCI repository. The obtained results confirm the interest of the proposed approach with respect to the existing ones.
更多查看译文
关键词
Basic belief assignment,Belief functions,Classification,Dirichlet mixture process
AI 理解论文
溯源树
样例
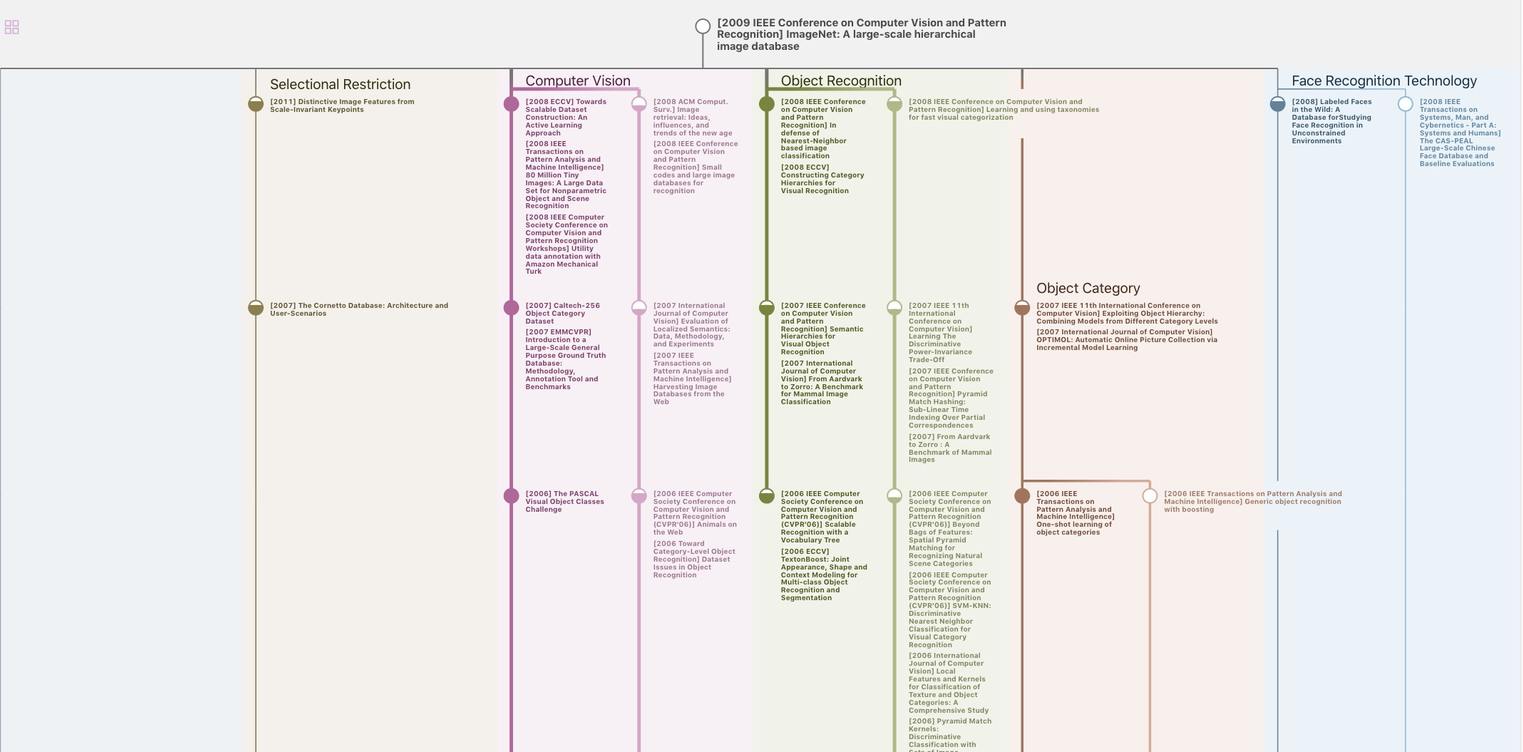
生成溯源树,研究论文发展脉络
Chat Paper
正在生成论文摘要