Reconstruction of Sparse Graph Signals from Reduced Sets of Samples
2023 27th International Conference on Information Technology (IT)(2023)
摘要
The reconstruction of sparse graph signals based on a reduced set of samples is a relevant topic, since graphs, acting as irregular signal domains, may comprise a large number of vertices, millions, or even billions. The related storage and computational issues motivate the involvement of subsampling and compressive sensing concepts applied to graphs. We investigate the fundamental differences in the conditions for the reconstruction based on a reduced set of measurements, in the general case of graph signal processing, versus the case of traditional signal processing. There are two frameworks for such reconstruction – one assuming that the positions of nonzero sparsity domain coefficients are a priori known, and the other – when those positions are unknown and must be estimated based on the available measurements. In the first framework, on which we focus in this article, the conditions are in general far less conservative than in the second framework, which involves the compressive sensing (CS) paradigm. The particular structure of the graph may significantly influence the results, therefore bringing to the fore the concept of measurements formed as a linear combination of samples.
更多查看译文
AI 理解论文
溯源树
样例
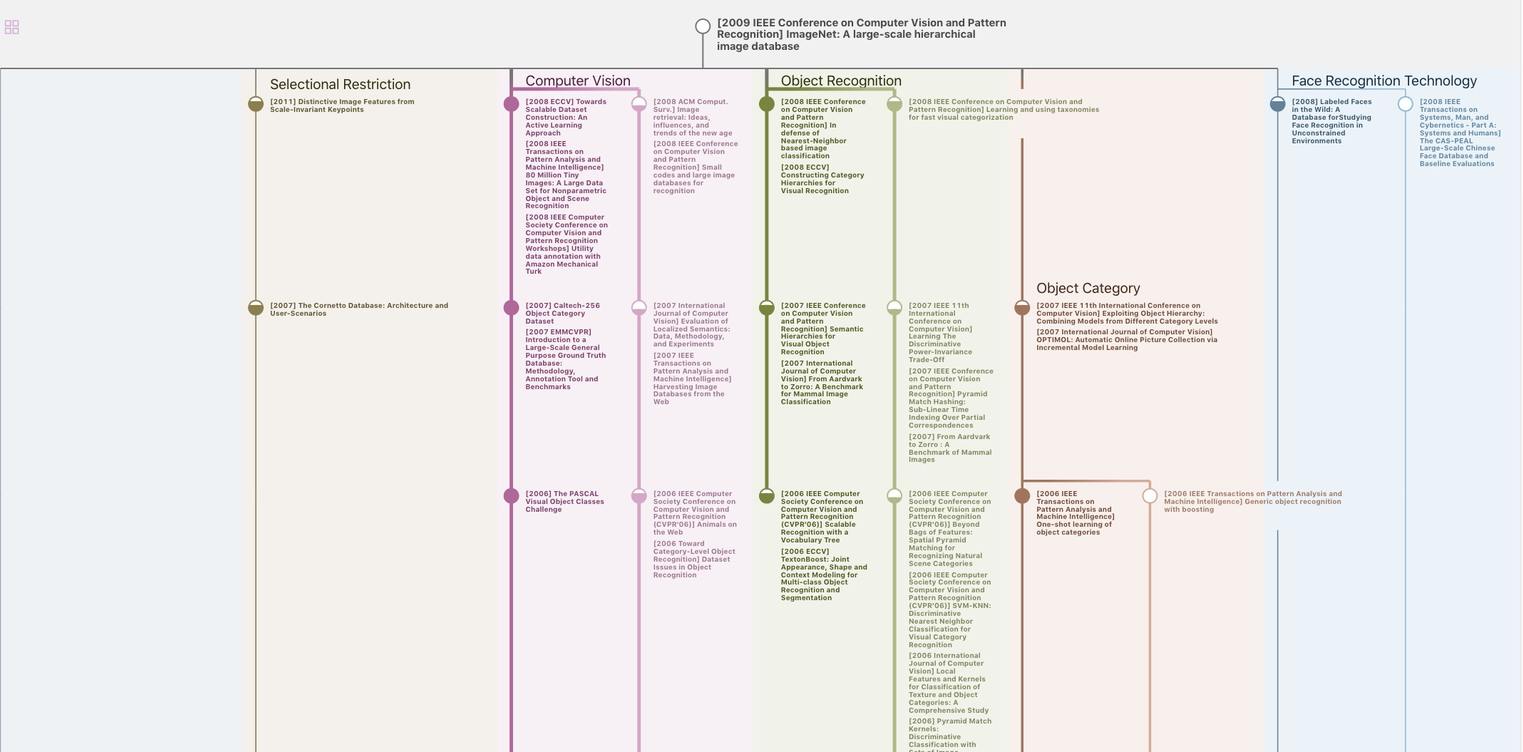
生成溯源树,研究论文发展脉络
Chat Paper
正在生成论文摘要