Comparative Analysis of three machine learning models for Early Prediction of Skeletal Class-III Malocclusion from Profile Photos
medrxiv(2022)
摘要
The pre-adolescent growth period is the best time for the skeletal Class-III malocclusion treatment. Diagnosis and treatment during this period continue to be a complex orthodontic problem. Class-III malocclusion is complicated to treat with braces frequently requiring surgical intervention after a pubertal growth spurt. In addition, delayed recognition of the problem will yield significant functional, aesthetic, and psychological concerns. This study presents the first fully automated machine learning method to accurately diagnose Class-III malocclusion applied across mobile images, to the best of our knowledge. For this purpose, we comparatively evaluated three machine learning approaches: a deep learning algorithm, a machine learning algorithm, and a rule-based algorithm. We collected a novel profile image data set for this analysis along with their formal diagnosis from 435 orthodontics patients. The most successful method among the three was the machine learning method, with an accuracy of %76.
### Competing Interest Statement
The authors have declared no competing interest.
### Funding Statement
This study was funded by TUBITAK-TEYDEB
### Author Declarations
I confirm all relevant ethical guidelines have been followed, and any necessaxry IRB and/or ethics committee approvals have been obtained.
Yes
The details of the IRB/oversight body that provided approval or exemption for the research described are given below:
IRB# 54022451-050.05.04 Orthodontics at Bezmialem Vakif University Faculty of Dentistry. IRB approval (IRB# 54022451-050.05.04) was obtained from Bezmialem Vakif University to use profile image data of real patients who visited Bezmialem Vakif University in our project. All patients parents approved the use of videos and photos of their children by signing a consent form as part of this study. All consent forms are available in digital format.
I confirm that all necessary patient/participant consent has been obtained and the appropriate institutional forms have been archived, and that any patient/participant/sample identifiers included were not known to anyone (e.g., hospital staff, patients or participants themselves) outside the research group so cannot be used to identify individuals.
Yes
I understand that all clinical trials and any other prospective interventional studies must be registered with an ICMJE-approved registry, such as ClinicalTrials.gov. I confirm that any such study reported in the manuscript has been registered and the trial registration ID is provided (note: if posting a prospective study registered retrospectively, please provide a statement in the trial ID field explaining why the study was not registered in advance).
Yes
I have followed all appropriate research reporting guidelines and uploaded the relevant EQUATOR Network research reporting checklist(s) and other pertinent material as supplementary files, if applicable.
Yes
All data produced in the present study are available upon reasonable request to the authors.
更多查看译文
关键词
skeleton class iii malocclusion,deep learning,machine learning models,early prediction
AI 理解论文
溯源树
样例
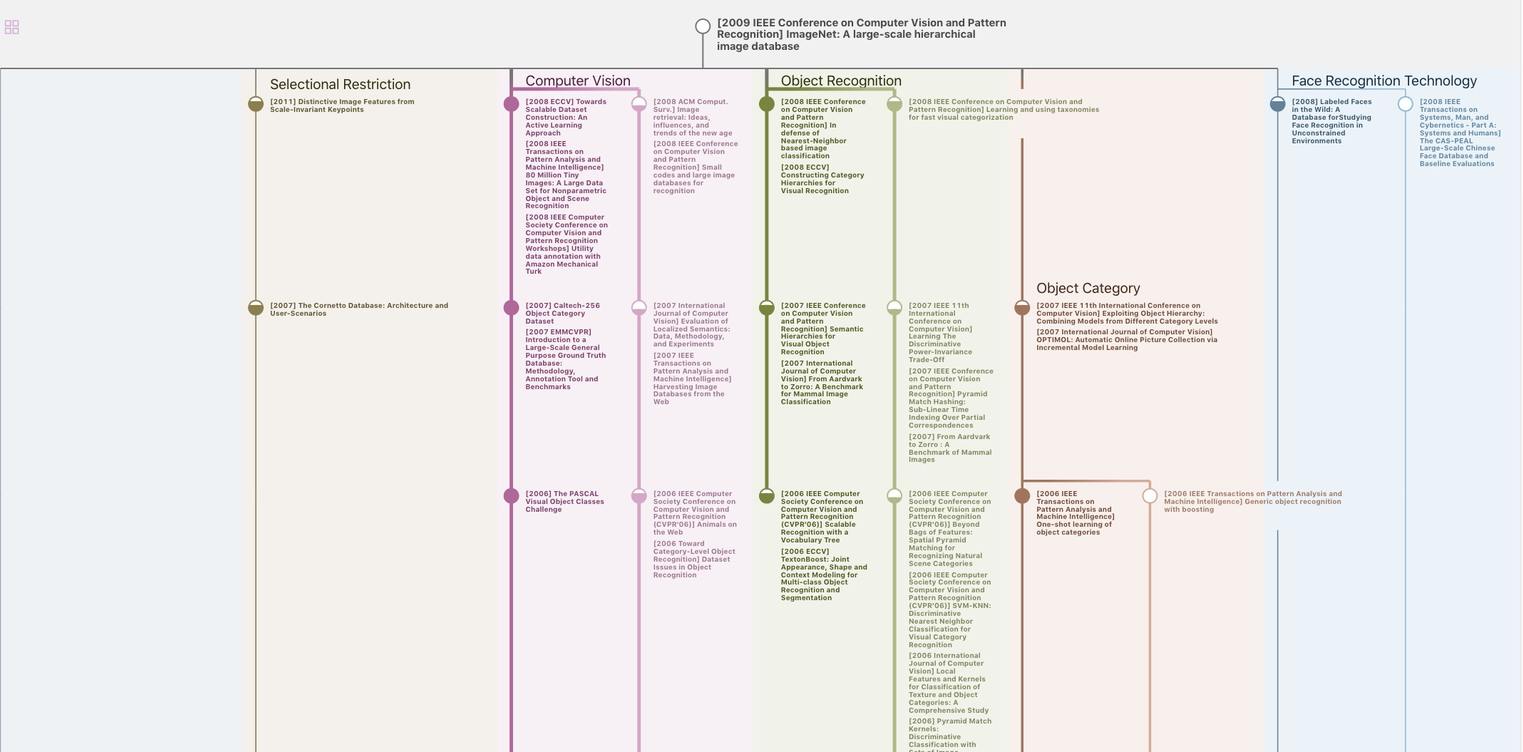
生成溯源树,研究论文发展脉络
Chat Paper
正在生成论文摘要