Multimodal MALDI imaging mass spectrometry for improved diagnosis of melanoma
medrxiv(2022)
摘要
Imaging mass spectrometry (IMS) provides promising avenues to augment histopathological investigation with rich spatio-molecular information. We have previously developed a classification model to differentiate melanoma from nevi lesions based on IMS protein data, a task that is challenging solely by histopathologic evaluation.
Most IMS-focused studies collect microscopy in tandem with IMS data, but this microscopy data is generally omitted in downstream data analysis. Microscopy, nevertheless, forms the basis for traditional histopathology and thus contains invaluable morphological information. In this work, we developed a multimodal classification pipeline that uses deep learning, in the form of a pre-trained artificial neural network, to extract the meaningful morphological features from histopathological images, and combine it with the IMS data.
To test whether this deep learning-based classification strategy can improve on our previous results in classification of melanocytic neoplasia, we utilized MALDI IMS data with collected serial H&E stained sections for 331 patients, and compared this multimodal classification pipeline to classifiers using either exclusively microscopy or IMS data. The multimodal pipeline achieved the best performance, with ROC-AUCs of 0.968 vs. 0.938 vs. 0.931 for the multimodal, unimodal microscopy and unimodal IMS pipelines respectively. Due to the use of a pre-trained network to perform the morphological feature extraction, this pipeline does not require any training on large amounts of microscopy data. As such, this framework can be readily applied to improve classification performance in other experimental settings where microscopy data is acquired in tandem with IMS experiments.
### Competing Interest Statement
NHP, JLM, RMC, and JLN, disclose a financial interest in Frontier Diagnostics, LLC (FDx). FDx has issued and pending patent applications in the US Patent Office that include part of the methods described in this paper. NV and MC, principals of Aspect Analytics NV, are paid consultants and provide services to FDx.
### Funding Statement
This work was funded by Small Business Innovative Research Grant No. R44CA228897-02, provided by the National Cancer Institute of the National Institutes of Health. Wanqiu Zhang was supported by a Baekeland PhD grant from Flanders Innovation and Entrepreneurship (VLAIO). KU Leuven: Research Fund (projects C16/15/059, C3/19/053, C24/18/022, C3/20/117, C3I-21-00316), Industrial Research Fund (Fellowships 13-0260, IOFm/16/004) and several Leuven Research and Development bilateral industrial projects; Flemish Government Agencies: FWO: EOS Project no G0F6718N (SeLMA), SBO project S005319N, Infrastructure project I013218N, TBM Project T001919N; PhD Grant (SB/1SA1319N), EWI: the Flanders AI Research Program, VLAIO: CSBO (HBC.2021.0076) Baekeland PhD (HBC.20192204), European Commission: European Research Council under the European Union's Horizon 2020 research and innovation programme (ERC Adv. Grant grant agreement No 885682); Other funding: Foundation 'Kom op tegen Kanker', CM (Christelijke Mutualiteit).
### Author Declarations
I confirm all relevant ethical guidelines have been followed, and any necessary IRB and/or ethics committee approvals have been obtained.
Yes
The details of the IRB/oversight body that provided approval or exemption for the research described are given below:
Sterling Institutional Review Board (Atlanta, GA) and the institutional review boards (IRB) of Indiana University (IRB number:1910325060), Vanderbilt University (IRB 030220), Duke University (IRB Pro00102363) determined this study exempt from IRB review under the terms of the US Department of Health and Human Services Policy for Protection of Human Research Subjects at 45 CFR 46.104(d).
I confirm that all necessary patient/participant consent has been obtained and the appropriate institutional forms have been archived, and that any patient/participant/sample identifiers included were not known to anyone (e.g., hospital staff, patients or participants themselves) outside the research group so cannot be used to identify individuals.
Yes
I understand that all clinical trials and any other prospective interventional studies must be registered with an ICMJE-approved registry, such as ClinicalTrials.gov. I confirm that any such study reported in the manuscript has been registered and the trial registration ID is provided (note: if posting a prospective study registered retrospectively, please provide a statement in the trial ID field explaining why the study was not registered in advance).
Yes
I have followed all appropriate research reporting guidelines and uploaded the relevant EQUATOR Network research reporting checklist(s) and other pertinent material as supplementary files, if applicable.
Yes
The data on which this study is based cannot be disclosed.
更多查看译文
关键词
mass spectrometry,imaging
AI 理解论文
溯源树
样例
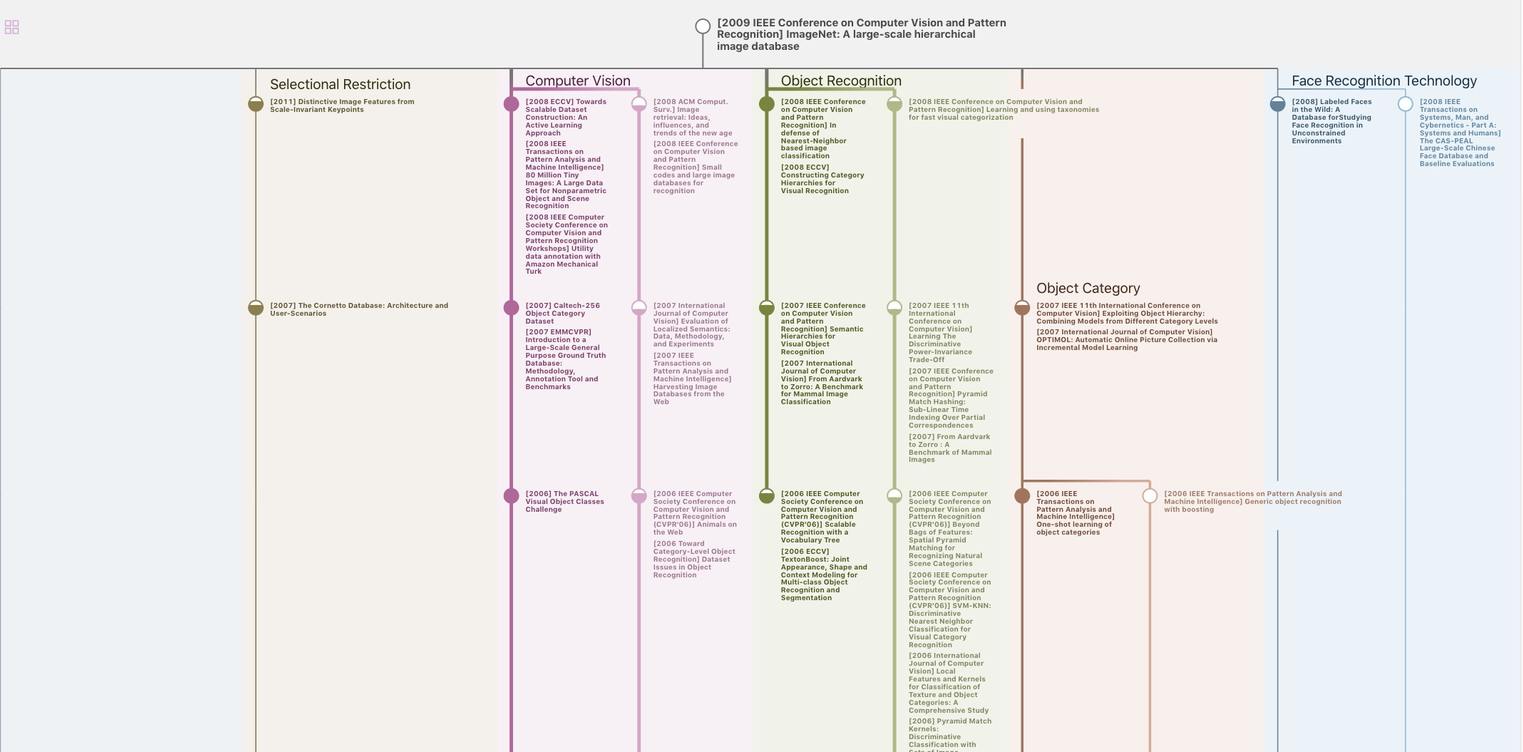
生成溯源树,研究论文发展脉络
Chat Paper
正在生成论文摘要