Systematic analysis of off-label and off-guideline cancer therapy usage in a real-world cohort of 153,122 U.S. patients
medRxiv (Cold Spring Harbor Laboratory)(2023)
摘要
Patients with cancer may be given treatments that are not officially approved (off-label) or recommended by guidelines (off-guideline) for multiple reasons including a lack of effective approved treatments. Here we present a systematic characterization of the patterns of off-label and off-guideline usage in 153,122 U.S. patients with 14 common cancer types using a large electronic health record (EHR)-derived de-identified database. We find that 18.3% and 3.9% of patients have received at least one line of off-label and off-guideline cancer drugs, respectively. Out of the 14 malignancies investigated, advanced bladder cancer has the highest proportion with 8.1% of patients receiving off-guideline treatments, most of which are recommended for non-small cell lung cancer. Patients with worse performance status, in later lines, or treated at academic hospitals are significantly more likely to receive off-label and off-guideline drugs. Underrepresented minority patients are less likely to receive off-guideline treatments in several cancer types. To quantify how predictable off-guideline usage is, we developed machine learning models to predict which drug a patient is likely to receive based on their clinical characteristics and previous treatments. Finally, we demonstrate that our systematic analysis of large real-world cohorts can identify interesting candidates for potential label expansion by identifying off-label treatments that demonstrate effectiveness in the real world setting. For example, we find that hormonal agents approved for breast cancer are used off-label in patients with ovarian cancer. Moreover, these hormonal agents show promising effectiveness in ovarian cancer with adjusted hazard ratio 0.53 (0.44, 0.65) compared to standard-of-care. This work demonstrates the power of large-scale computational analysis of real-world data for investigating non-standard cancer treatment usages.
### Competing Interest Statement
L.W., S.R., M.R.G, N.P., S.M., Y.G.L., and R.C. are employees of Roche-Genentech.
### Funding Statement
L.W., S.R., M.R.G, N.P., S.M., Y.G.L., and R.C. are supported by funding from Roche.
### Author Declarations
I confirm all relevant ethical guidelines have been followed, and any necessary IRB and/or ethics committee approvals have been obtained.
Yes
The details of the IRB/oversight body that provided approval or exemption for the research described are given below:
Portions of research conducted by Flatiron Health were approved with waiver of informed consent by the WCG Institutional Review Board prior to study conduct
I confirm that all necessary patient/participant consent has been obtained and the appropriate institutional forms have been archived, and that any patient/participant/sample identifiers included were not known to anyone (e.g., hospital staff, patients or participants themselves) outside the research group so cannot be used to identify individuals.
Yes
I understand that all clinical trials and any other prospective interventional studies must be registered with an ICMJE-approved registry, such as ClinicalTrials.gov. I confirm that any such study reported in the manuscript has been registered and the trial registration ID is provided (note: if posting a prospective study registered retrospectively, please provide a statement in the trial ID field explaining why the study was not registered in advance).
Yes
I have followed all appropriate research reporting guidelines and uploaded the relevant EQUATOR Network research reporting checklist(s) and other pertinent material as supplementary files, if applicable.
Yes
Requests for data sharing of these de-identified data by license/by permission for the specific purpose of replicating results in this manuscript can be submitted to dataaccess@flatiron.com.
更多查看译文
关键词
cancer,systematic analysis,off-label,off-guideline,real-world
AI 理解论文
溯源树
样例
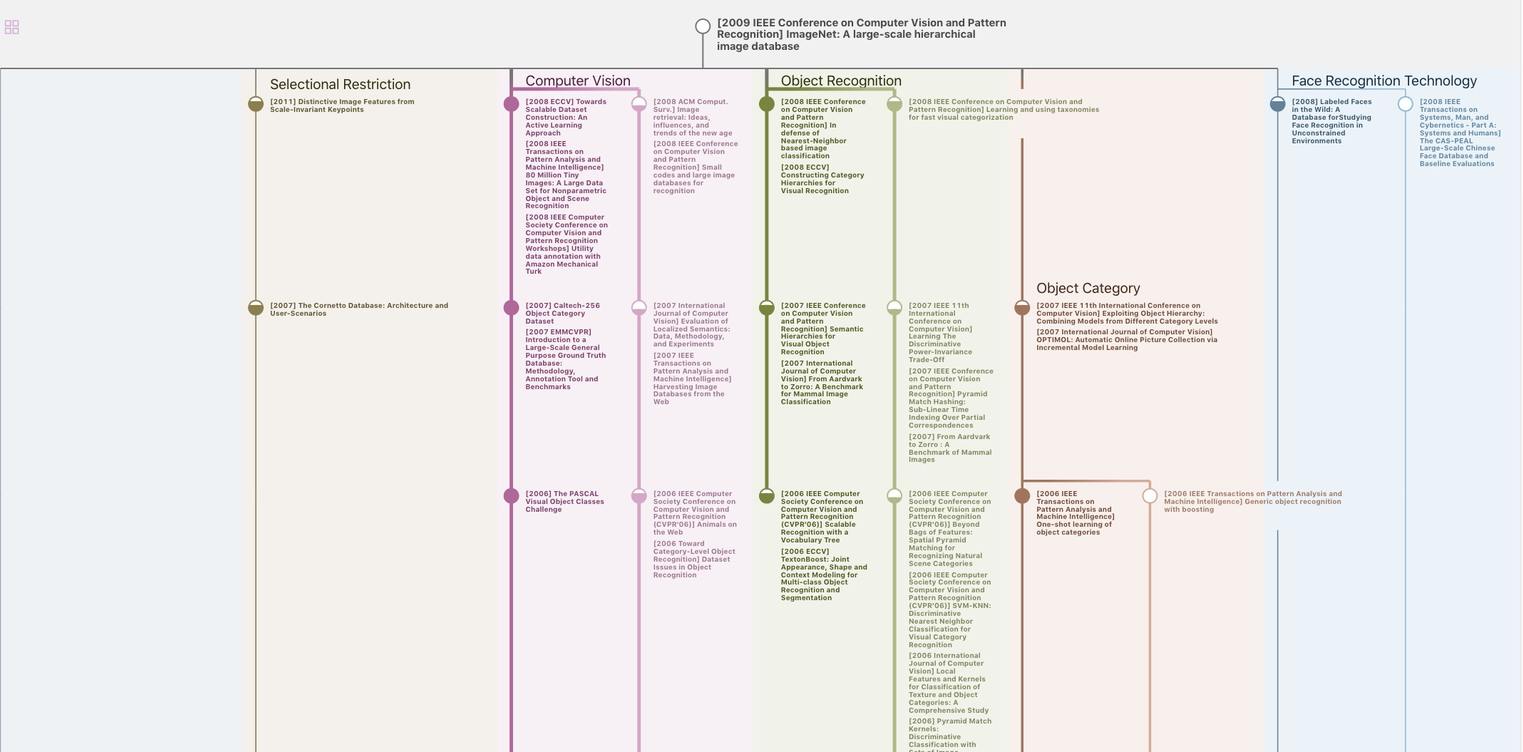
生成溯源树,研究论文发展脉络
Chat Paper
正在生成论文摘要